The surprising impact of mask-head architecture on novel class segmentation.
ICCV(2021)
摘要
Instance segmentation models today are very accurate when trained on large annotated datasets, but collecting mask annotations at scale is prohibitively expensive. We address the partially supervised instance segmentation problem in which one can train on (significantly cheaper) bounding boxes for all categories but use masks only for a subset of categories. In this work, we focus on a popular family of models which apply differentiable cropping to a feature map and predict a mask based on the resulting crop. Within this family, we show that the architecture of the mask-head plays a surprisingly important role in generalization to classes for which we do not observe masks during training. While many architectures perform similarly when trained in fully supervised mode, we show that they often generalize to novel classes in dramatically different ways. We call this phenomenon the strong mask generalization effect, which we exploit by replacing the typical mask-head of 2-4 layers with significantly deeper off-the-shelf architectures (e.g. ResNet, Hourglass models). We also show that the choice of mask-head architecture alone can lead to SOTA results on the partially supervised COCO benchmark without the need of specialty modules or losses proposed by prior literature. Finally, we demonstrate that our effect is general, holding across underlying detection methodologies, (e.g. both anchor-based or anchor free or no detector at all) and across different backbone networks. Code and pre-trained models are available at https://git.io/deepmac.
更多查看译文
关键词
Segmentation,grouping and shape,Detection and localization in 2D and 3D
AI 理解论文
溯源树
样例
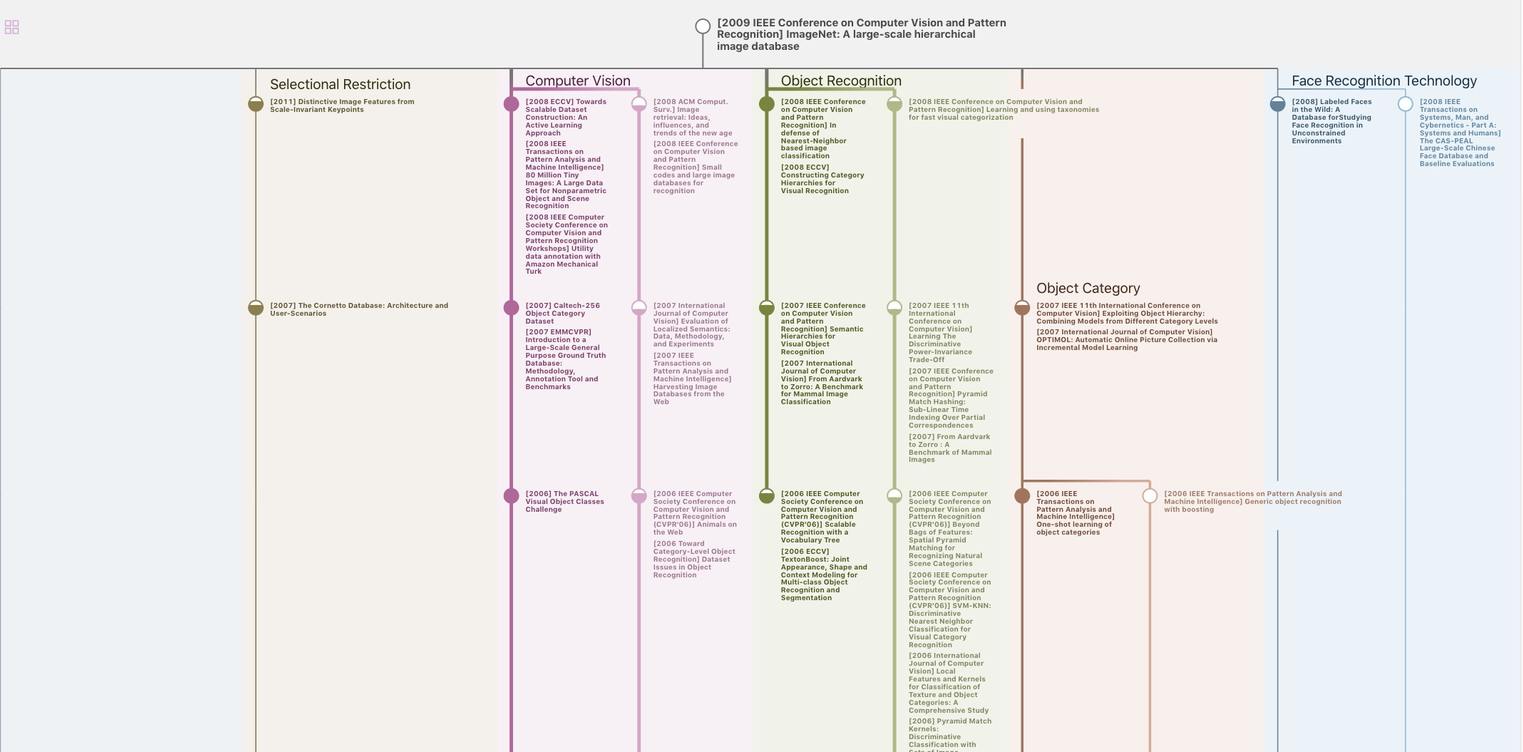
生成溯源树,研究论文发展脉络
Chat Paper
正在生成论文摘要