Deep Two-View Structure-from-Motion Revisited
2021 IEEE/CVF CONFERENCE ON COMPUTER VISION AND PATTERN RECOGNITION, CVPR 2021(2021)
摘要
Two-view structure-from-motion (SfM) is the cornerstone of 3D reconstruction and visual SLAM. Existing deep learning-based approaches formulate the problem by either recovering absolute pose scales from two consecutive frames or predicting a depth map from a single image, both of which are ill-posed problems. In contrast, we propose to revisit the problem of deep two-view SfM by leveraging the well-posedness of the classic pipeline. Our method consists of 1) an optical flow estimation network that predicts dense correspondences between two frames; 2) a normalized pose estimation module that computes relative camera poses from the 2D optical flow correspondences, and 3) a scale-invariant depth estimation network that leverages epipolar geometry to reduce the search space, refine the dense correspondences, and estimate relative depth maps. Extensive experiments show that our method outperforms all state-of-the-art two-view SfM methods by a clear margin on KITTI depth, KITTI VO, MVS, Scenes11, and SUN3D datasets in both relative pose and depth estimation.
更多查看译文
关键词
pose estimation module,camera poses,2D optical flow correspondences,scale-invariant depth estimation network,deep two-view structure-from-motion,deep learning,depth map,optical flow estimation network,depth maps estimation,two-view SfM,3D reconstruction,visual SLAM
AI 理解论文
溯源树
样例
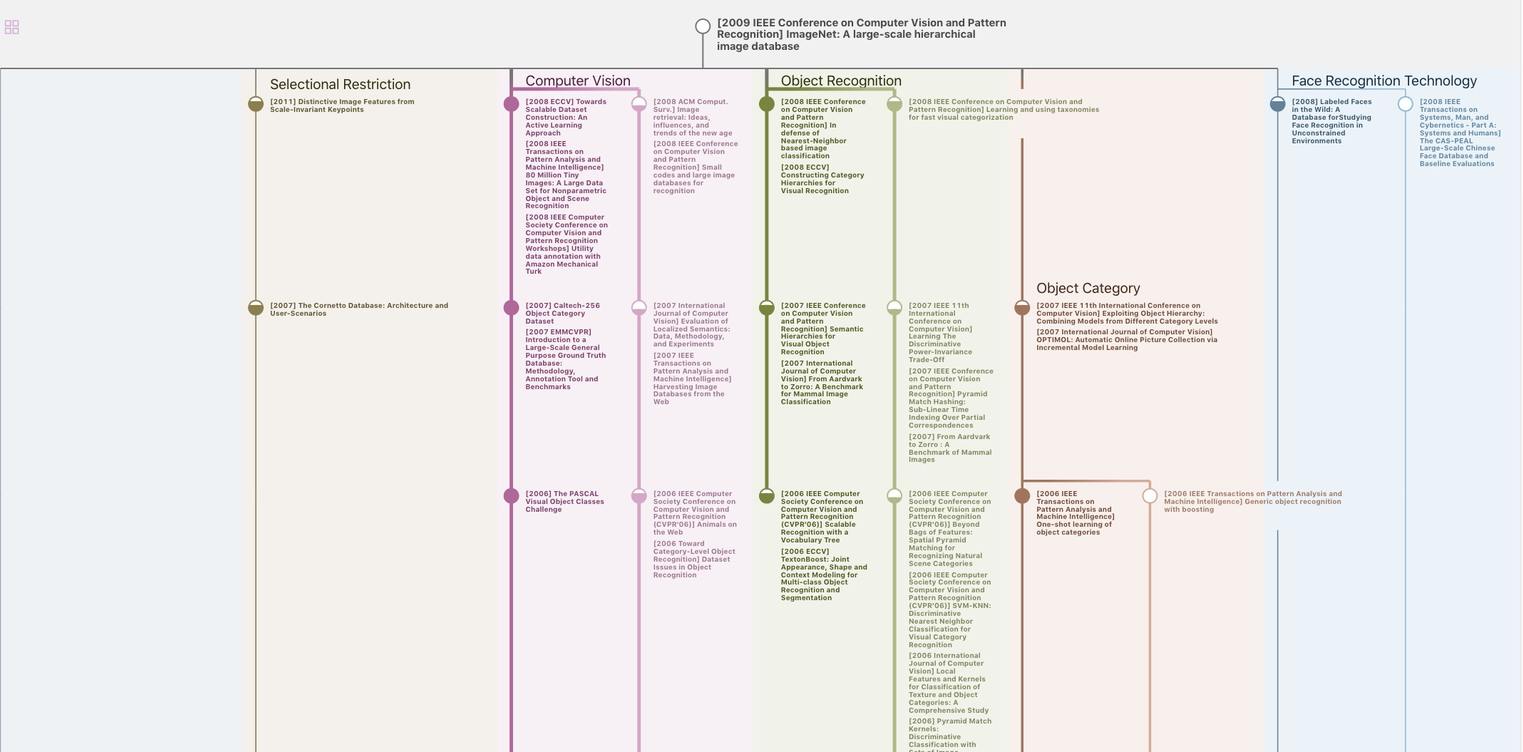
生成溯源树,研究论文发展脉络
Chat Paper
正在生成论文摘要