Anchor pruning for object detection
Computer Vision and Image Understanding(2022)
摘要
This paper proposes anchor pruning for object detection in one-stage anchor-based detectors. While pruning techniques are widely used to reduce the computational cost of convolutional neural networks, they tend to focus on optimizing the backbone networks where often most computations are. In this work we demonstrate an additional pruning technique, specifically for object detection: anchor pruning. With more efficient backbone networks and a growing trend of deploying object detectors on embedded systems where post-processing steps such as non-maximum suppression can be a bottleneck, the impact of the anchors used in the detection head is becoming increasingly more important. In this work, we show that many anchors in the object detection head can be removed without any loss in accuracy. With additional retraining, anchor pruning can even lead to improved accuracy. Extensive experiments on SSD and MS COCO show that the detection head can be made up to 44% more efficient while simultaneously increasing accuracy. Further experiments on RetinaNet and PASCAL VOC show the general effectiveness of our approach. We also introduce ‘overanchorized’ models that can be used together with anchor pruning to eliminate hyperparameters related to the initial shape of anchors. Code and models are available at .
更多查看译文
关键词
68T07,68T45
AI 理解论文
溯源树
样例
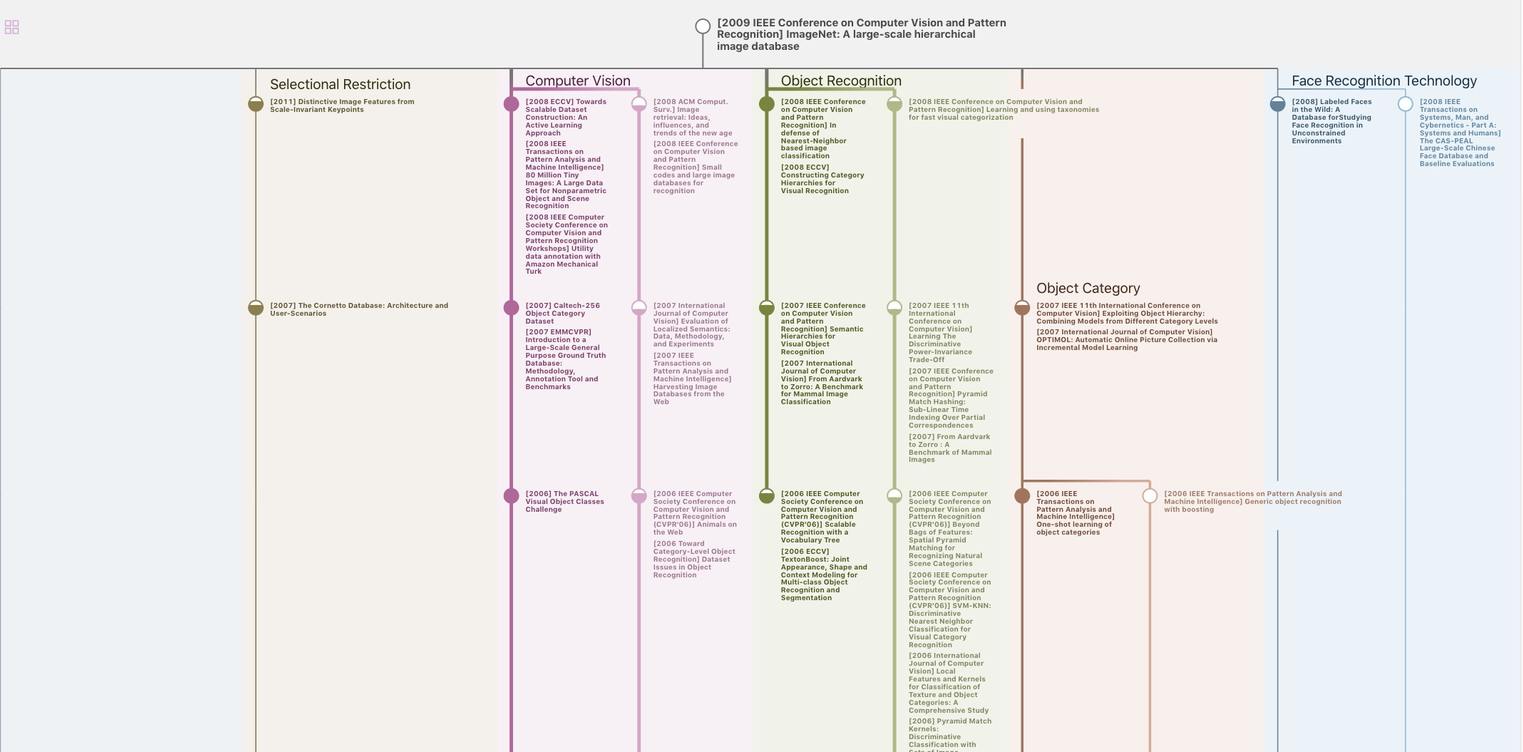
生成溯源树,研究论文发展脉络
Chat Paper
正在生成论文摘要