Learning with Neural Tangent Kernels in Near Input Sparsity Time
arxiv(2021)
摘要
The Neural Tangent Kernel (NTK) characterizes the behavior of infinitely wide neural nets trained under least squares loss by gradient descent (Jacot et al., 2018). However, despite its importance, the super-quadratic runtime of kernel methods limits the use of NTK in large-scale learning tasks. To accelerate kernel machines with NTK, we propose a near input sparsity time algorithm that maps the input data to a randomized low-dimensional feature space so that the inner product of the transformed data approximates their NTK evaluation. Furthermore, we propose a feature map for approximating the convolutional counterpart of the NTK (Arora et al., 2019), which can transform any image using a runtime that is only linear in the number of pixels. We show that in standard large-scale regression and classification tasks a linear regressor trained on our features outperforms trained NNs and Nystrom method with NTK kernels.
更多查看译文
关键词
neural tangent kernels,learning
AI 理解论文
溯源树
样例
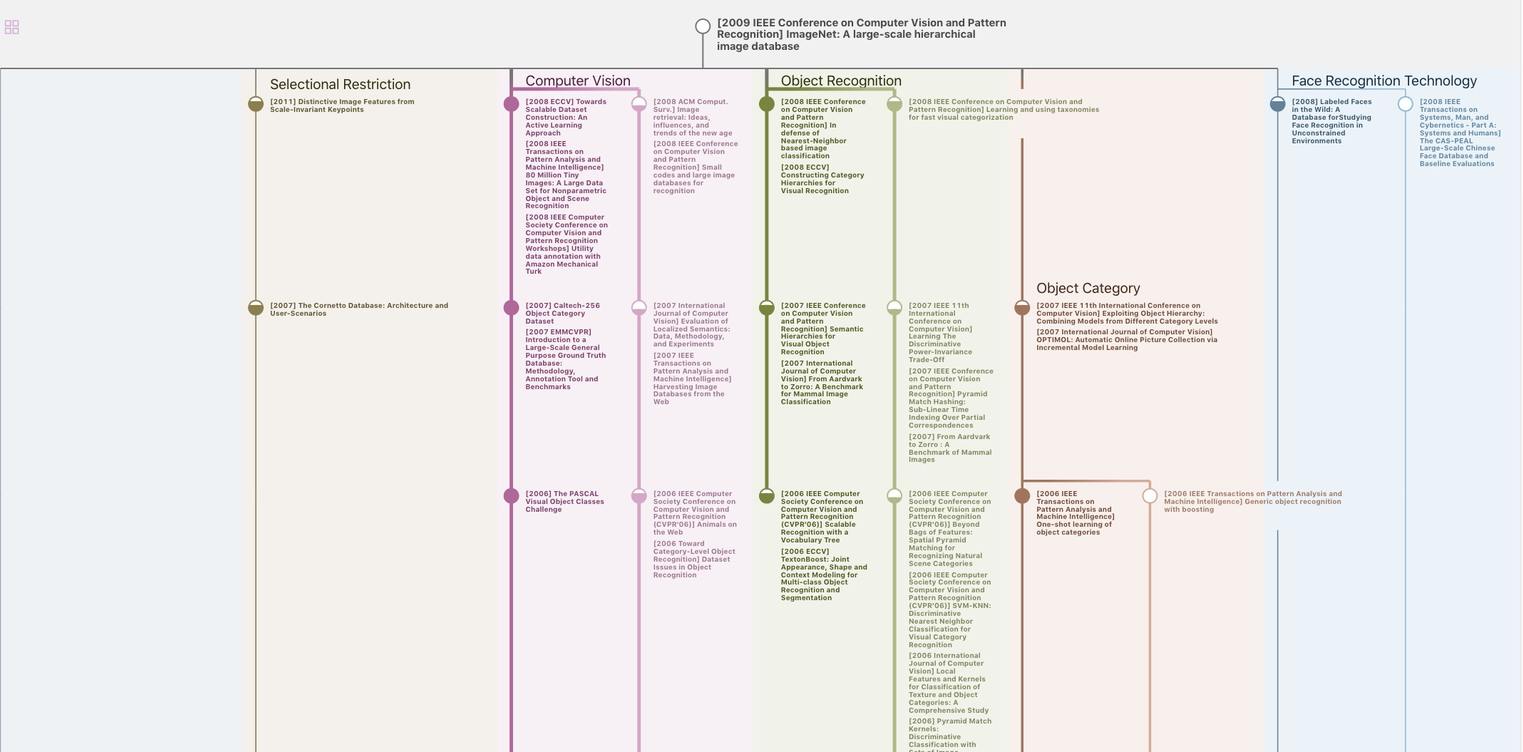
生成溯源树,研究论文发展脉络
Chat Paper
正在生成论文摘要