Differentially Private Histograms under Continual Observation: Streaming Selection into the Unknown
INTERNATIONAL CONFERENCE ON ARTIFICIAL INTELLIGENCE AND STATISTICS, VOL 151(2022)
摘要
We generalize the continuous observation privacy setting from Dwork et al. (2010a) and Chan et al. (2011) by allowing each event in a stream to be a subset of some (possibly unknown) universe of items. We design differentially private (DP) algorithms for histograms in several settings, including top-k selection, with privacy loss that scales with polylog(T), where T is the maximum length of the input stream. We present a meta-algorithm that can use existing one-shot top-k private algorithms as a subroutine to continuously release DP histograms from a stream. Further, we present more practical DP algorithms for two settings: 1) continuously releasing the top-k counts from a histogram over a known domain when an event can consist of an arbitrary number of items, and 2) continuously releasing histograms over an unknown domain when an event has few items.
更多查看译文
关键词
private histograms,continual observation,streaming
AI 理解论文
溯源树
样例
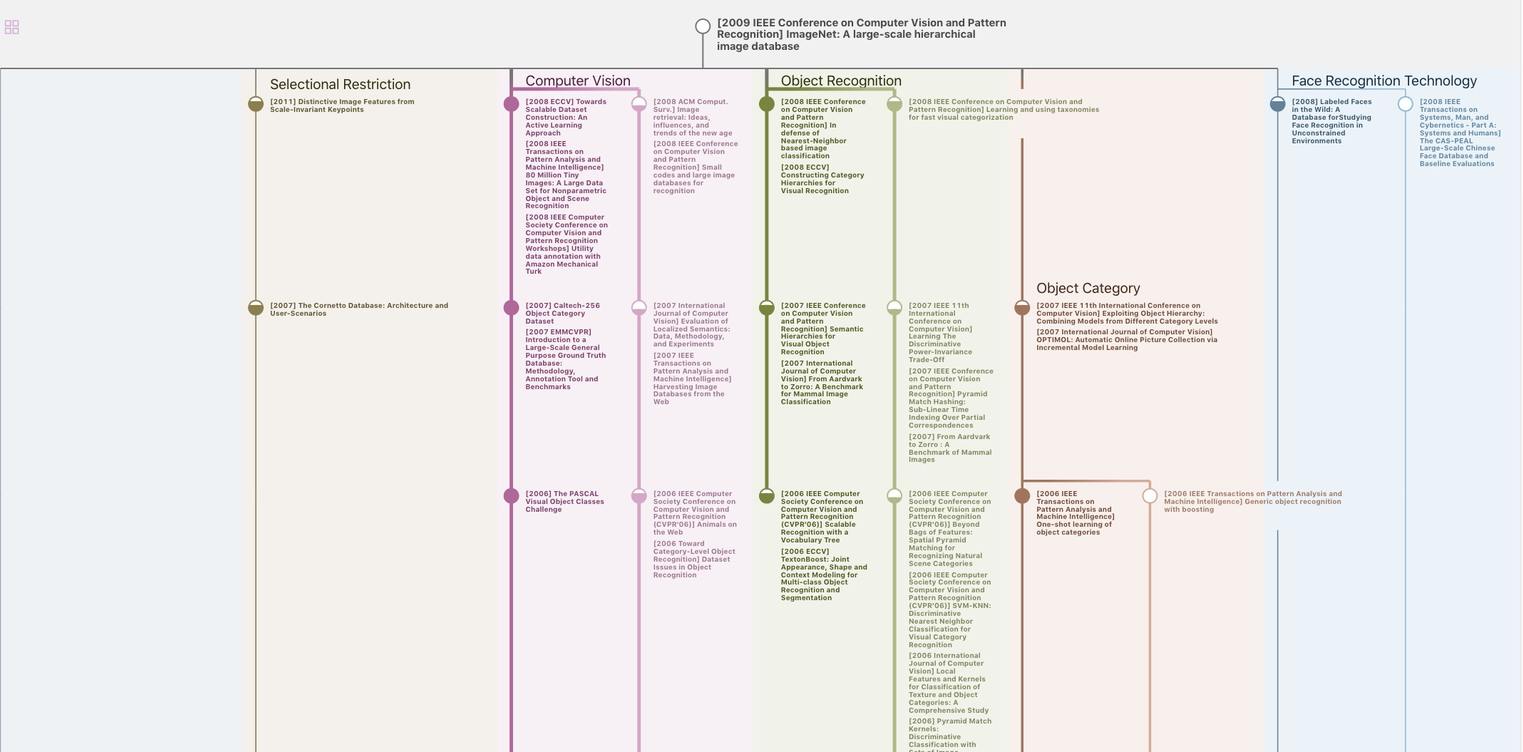
生成溯源树,研究论文发展脉络
Chat Paper
正在生成论文摘要