LemgoRL: An open-source Benchmark Tool to Train Reinforcement Learning Agents for Traffic Signal Control in a real-world simulation scenario
arxiv(2021)
摘要
Sub-optimal control policies in intersection traffic signal controllers (TSC) contribute to congestion and lead to negative effects on human health and the environment. Reinforcement learning (RL) for traffic signal control is a promising approach to design better control policies and has attracted considerable research interest in recent years. However, most work done in this area used simplified simulation environments of traffic scenarios to train RL-based TSC. To deploy RL in real-world traffic systems, the gap between simplified simulation environments and real-world applications has to be closed. Therefore, we propose LemgoRL, a benchmark tool to train RL agents as TSC in a realistic simulation environment of Lemgo, a medium-sized town in Germany. In addition to the realistic simulation model, LemgoRL encompasses a traffic signal logic unit that ensures compliance with all regulatory and safety requirements. LemgoRL offers the same interface as the well-known OpenAI gym toolkit to enable easy deployment in existing research work. Our benchmark tool drives the development of RL algorithms towards real-world applications. We provide LemgoRL as an open-source tool at https://github.com/rl-ina/lemgorl.
更多查看译文
关键词
Training,Machine learning algorithms,Conferences,Clustering algorithms,Transportation,Reinforcement learning,Benchmark testing
AI 理解论文
溯源树
样例
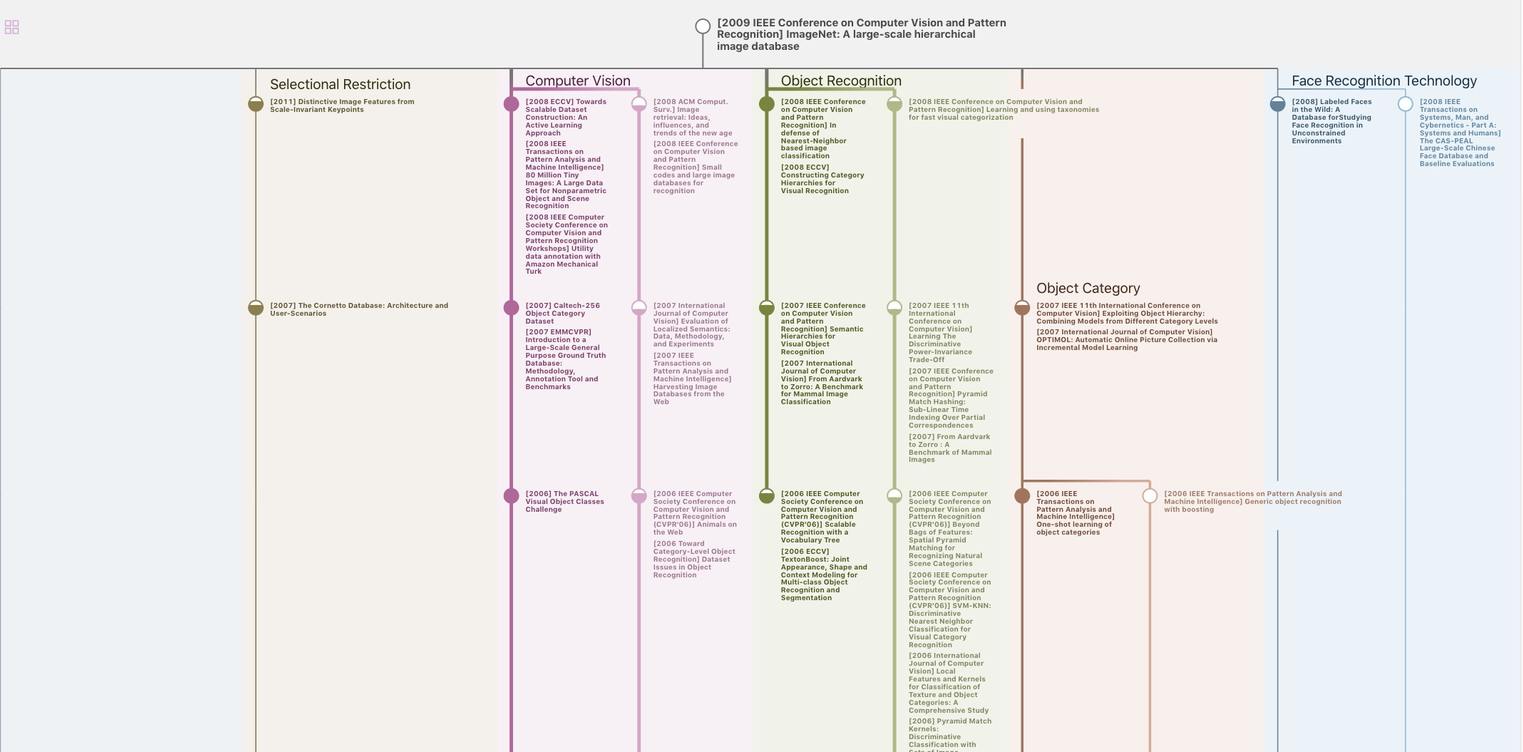
生成溯源树,研究论文发展脉络
Chat Paper
正在生成论文摘要