Density Estimation by Monte Carlo and Quasi-Monte Carlo
Monte Carlo and Quasi-Monte Carlo Methods(2022)
摘要
Estimating the density of a continuous random variable X has been studied extensively in statistics, in the setting where n independent observations of X are given a priori and one wishes to estimate the density from that. Popular methods include histograms and kernel density estimators. In this review paper, we are interested instead in the situation where the observations are generated by Monte Carlo simulation from a model. Then, one can take advantage of variance reduction methods such as stratification, conditional Monte Carlo, and randomized quasi-Monte Carlo (RQMC), and obtain a more accurate density estimator than with standard Monte Carlo for a given computing budget. We discuss several ways of doing this, proposed in recent papers, with a focus on methods that exploit RQMC. A first idea is to directly combine RQMC with a standard kernel density estimator. Another one is to adapt a simulation-based derivative estimation method such as smoothed perturbation analysis or the likelihood ratio method to obtain a continuous estimator of the cumulative density function (CDF), whose derivative is an unbiased estimator of the density. This can then be combined with RQMC. We summarize recent theoretical results with these approaches and give numerical illustrations of how they improve the convergence of the mean square integrated error.
更多查看译文
关键词
Density estimation, Conditional Monte Carlo, Likelihood ratio, Kernel density
AI 理解论文
溯源树
样例
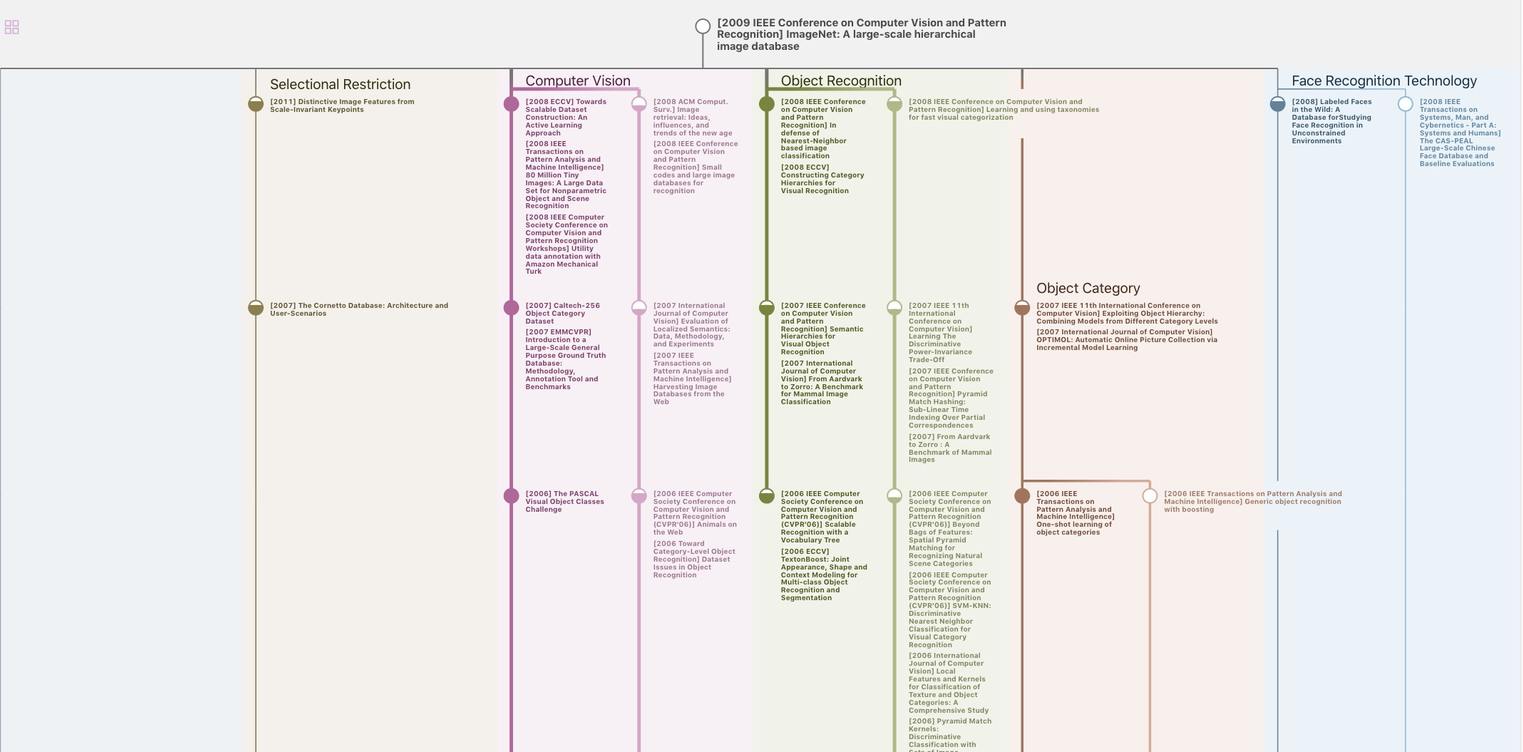
生成溯源树,研究论文发展脉络
Chat Paper
正在生成论文摘要