Multi-level Hyperedge Distillation for Social Linking Prediction on Sparsely Observed Networks
the web conference(2021)
摘要
ABSTRACT Social linking prediction is one of the most fundamental problems in online social networks and has attracted researchers’ persistent attention. Most of the existing works predict unobserved links using graph neural networks (GNNs) to learn node embeddings upon pair-wise relations. Despite promising results given enough observed links, these models are still challenging to achieve heart-stirring performance when observed links are extremely limited. The main reason is that they only focus on the smoothness of node representations on pair-wise relations. Unfortunately, this assumption may fall when the networks do not have enough observed links to support it. To this end, we go beyond pair-wise relations and propose a new and novel framework using hypergraph neural networks with multi-level hyperedge distillation strategies. To break through the limitations of sparsely observed links, we introduce the hypergraph to uncover higher-level relations, which is exceptionally crucial to deduce unobserved links. A hypergraph allows one edge to connect multiple nodes, making it easier to learn better higher-level relations for link prediction. To overcome the restrictions of manually designed hypergraphs, which is constant in most hypergraph researches, we propose a new method to learn high-quality hyperedges using three novel hyperedges distillation strategies automatically. The generated hyperedges are hierarchical and follow the power-law distribution, which can significantly improve the link prediction performance. To predict unobserved links, we present a novel hypergraph neural networks named HNN. HNN takes the multi-level hypergraphs as input and makes the node embeddings smooth on hyperedges instead of pair-wise links only. Extensive evaluations on four real-world datasets demonstrate our model’s superior performance over state-of-the-art baselines, especially when the observed links are extremely reduced.
更多查看译文
关键词
hypergraph learning, sparsely observed networks, linking prediction
AI 理解论文
溯源树
样例
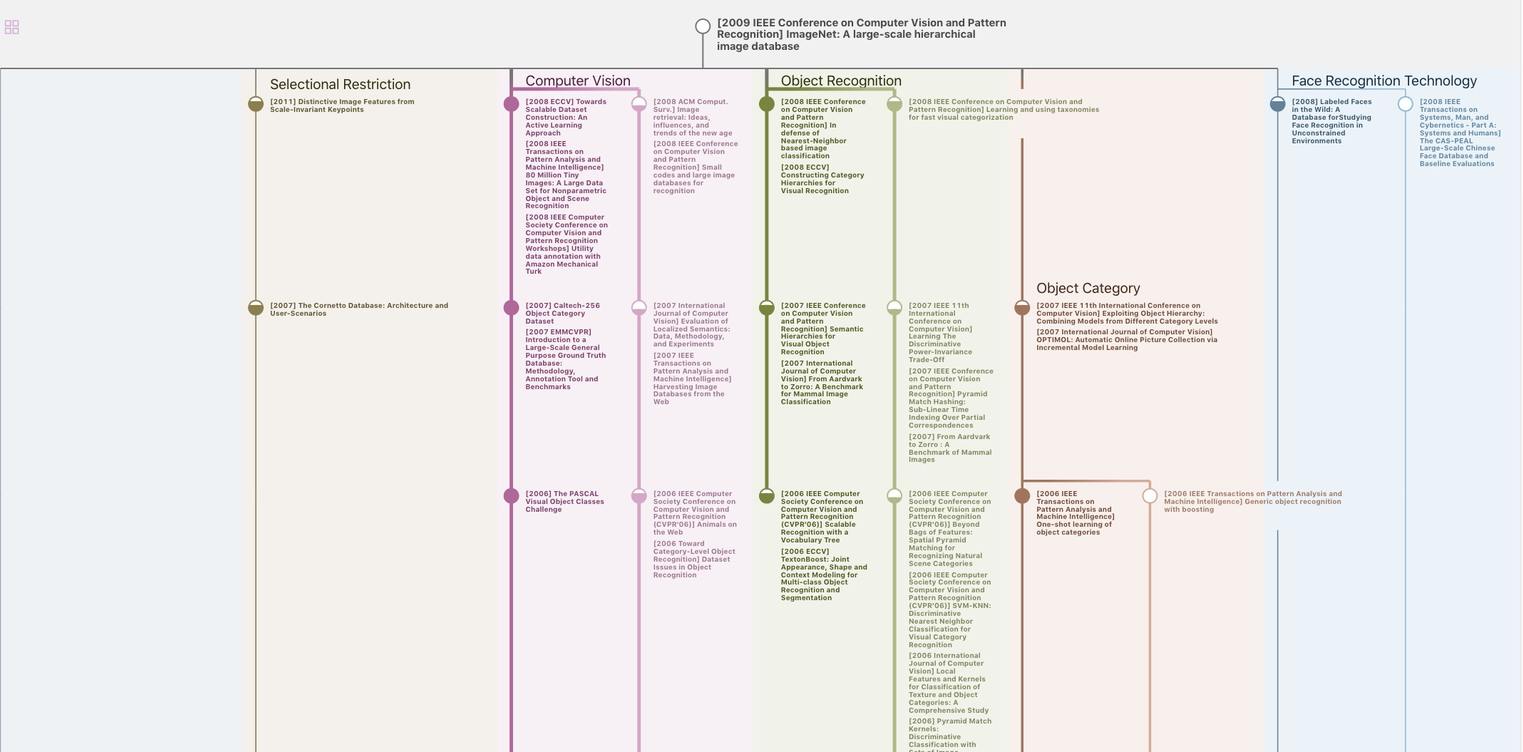
生成溯源树,研究论文发展脉络
Chat Paper
正在生成论文摘要