Inference of random effects for linear mixed-effects models with a fixed number of clusters
Annals of the Institute of Statistical Mathematics(2022)
摘要
We consider a linear mixed-effects model with a clustered structure, where the parameters are estimated using maximum likelihood (ML) based on possibly unbalanced data. Inference with this model is typically done based on asymptotic theory, assuming that the number of clusters tends to infinity with the sample size. However, when the number of clusters is fixed, classical asymptotic theory developed under a divergent number of clusters is no longer valid and can lead to erroneous conclusions. In this paper, we establish the asymptotic properties of the ML estimators of random-effects parameters under a general setting, which can be applied to conduct valid statistical inference with fixed numbers of clusters. Our asymptotic theorems allow both fixed effects and random effects to be misspecified, and the dimensions of both effects to go to infinity with the sample size.
更多查看译文
关键词
Confidence interval,Consistency,Maximum likelihood
AI 理解论文
溯源树
样例
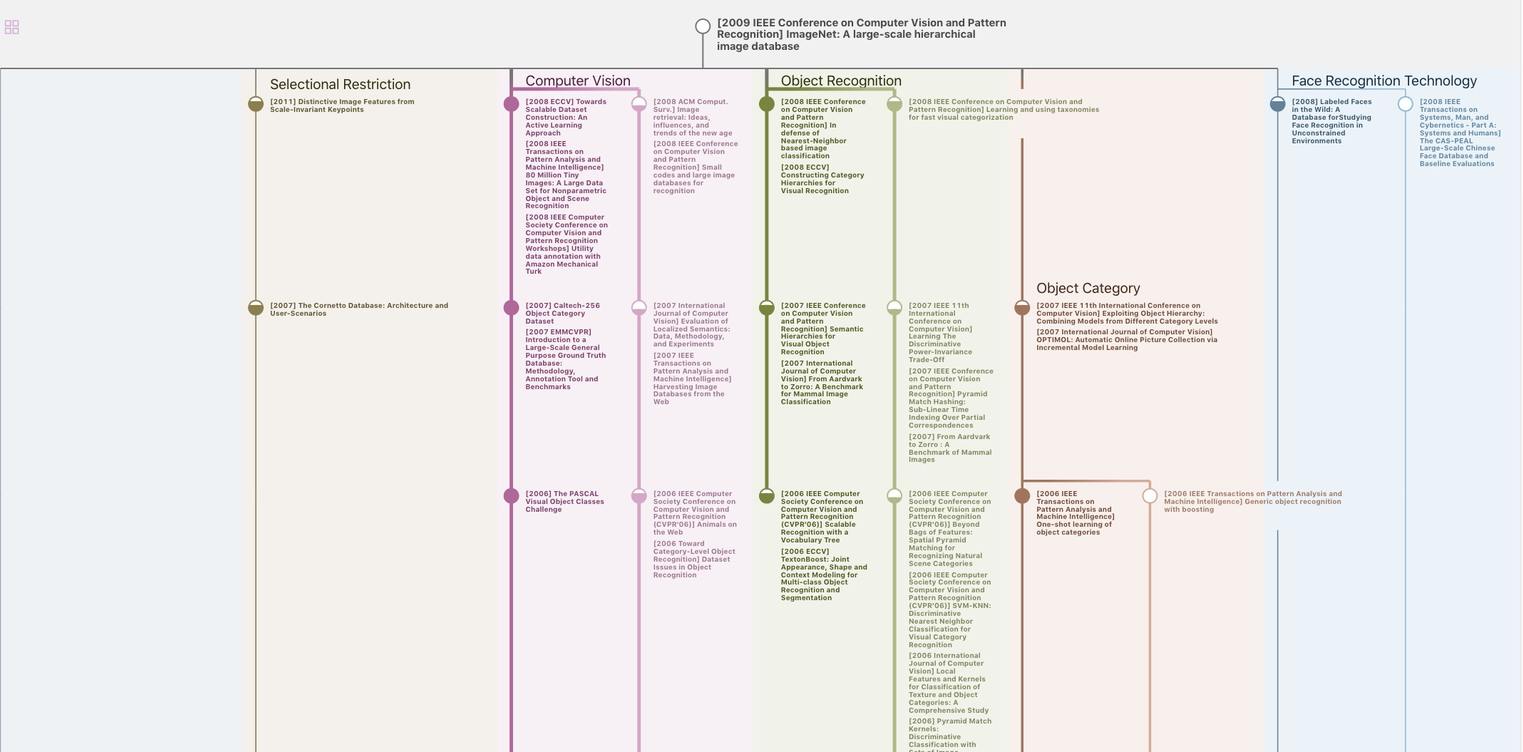
生成溯源树,研究论文发展脉络
Chat Paper
正在生成论文摘要