Inactive Behavior Analytics In On-Site Lectures
PROCEEDINGS OF 2020 IEEE INTERNATIONAL CONFERENCE ON TEACHING, ASSESSMENT, AND LEARNING FOR ENGINEERING (IEEE TALE 2020)(2020)
摘要
Detection of at-risk students is a fundamental issue in enhancing learning supports, and has been proposed based on students' learning activity in learning analytics. However, it is not clear which activity we should focus on to detect at-risk students such as low performance students. In this study, we proposed a clustering-based method for at-risk student detection based on three main clusters of students: inactive, passive, active students. Our method focused on reading behaviors and action behaviors in an e-book system. In addition, we consider which period of learning activities is effective for detecting at-risk students. The learning logs of 289 students of Cyber-Security course were collected for our analysis. In our comparison at different moment during the lecture, we found that the cluster of inactive students detected after 35 minutes of lecture got significant lower grades than other students, when the lecture was not too short nor too easy.
更多查看译文
关键词
learning analytics, inactive students, at-risk student detection, in-class data mining, behavior pattern analysis
AI 理解论文
溯源树
样例
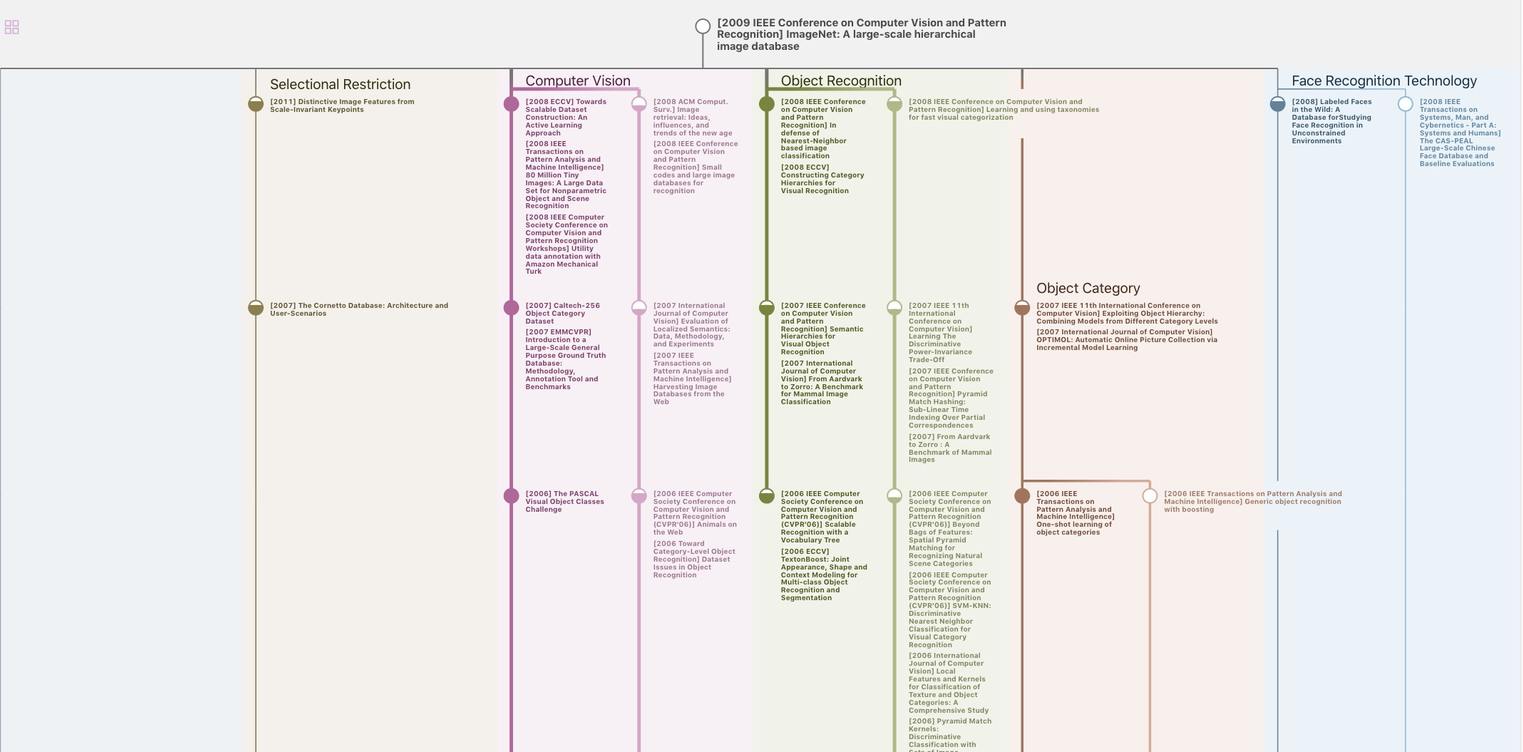
生成溯源树,研究论文发展脉络
Chat Paper
正在生成论文摘要