Estimation Of Evapotranspiration And Energy Fluxes Using A Deep-Learning Based High-Resolution Emissivity Model And The Two-Source Energy Balance Model With Suas Information
AUTONOMOUS AIR AND GROUND SENSING SYSTEMS FOR AGRICULTURAL OPTIMIZATION AND PHENOTYPING V(2020)
摘要
Surface temperature is necessary for the estimation of energy fluxes and evapotranspiration from satellites and airborne data sources. For example, the Two-Source Energy Balance (TSEB) model uses thermal information to quantify canopy and soil temperatures as well as their respective energy balance components. While surface (also called kinematic) temperature is desirable for energy balance analysis, obtaining this temperature is not straightforward due to a lack of spatially estimated narrowband (sensor-specific) and broadband emissivities of vegetation and soil, further complicated by spectral characteristics of the UAV thermal camera. This study presents an effort to spatially model narrowband and broadband emissivities for a microbolometer thermal camera at UAV information resolution (similar to 0.15 m) based on Landsat and NASA HyTES information using a deep learning (DL) model. The DL model is calibrated using equivalent optical Landsat / UAV spectral information to spatially estimate narrowband emissivity values of vegetation and soil in the 7-14-nm range at UAV resolution. The resulting DL narrowband emissivity values were then used to estimate broadband emissivity based on a developed narrowband-broadband emissivity relationship using the MODIS UCSB Emissivity Library database. The narrowband and broadband emissivities were incorporated into the TSEB model to determine their impact on the estimation of instantaneous energy balance components against ground measurements. The proposed effort was applied to information collected by the Utah State University AggieAir small Unmanned Aerial Systems (sUAS) Program as part of the ARS-USDA GRAPEX Project (Grape Remote sensing Atmospheric Profile and Evapotranspiration eXperiment) over a vineyard located in Lodi, California. A comparison of resulting energy balance component estimates, with and without the inclusion of high-resolution narrowband and broadband emissivities, against eddy covariance (EC) measurements under different scenarios are presented and discussed.
更多查看译文
关键词
High-resolution evapotranspiration, narrowband emissivity, broadband emissivity, microbolometer camera, deep learning, land surface temperature, UAV, microbolometer camera, NASA HYTES, UCSB MODIS Emissivity, Landsat
AI 理解论文
溯源树
样例
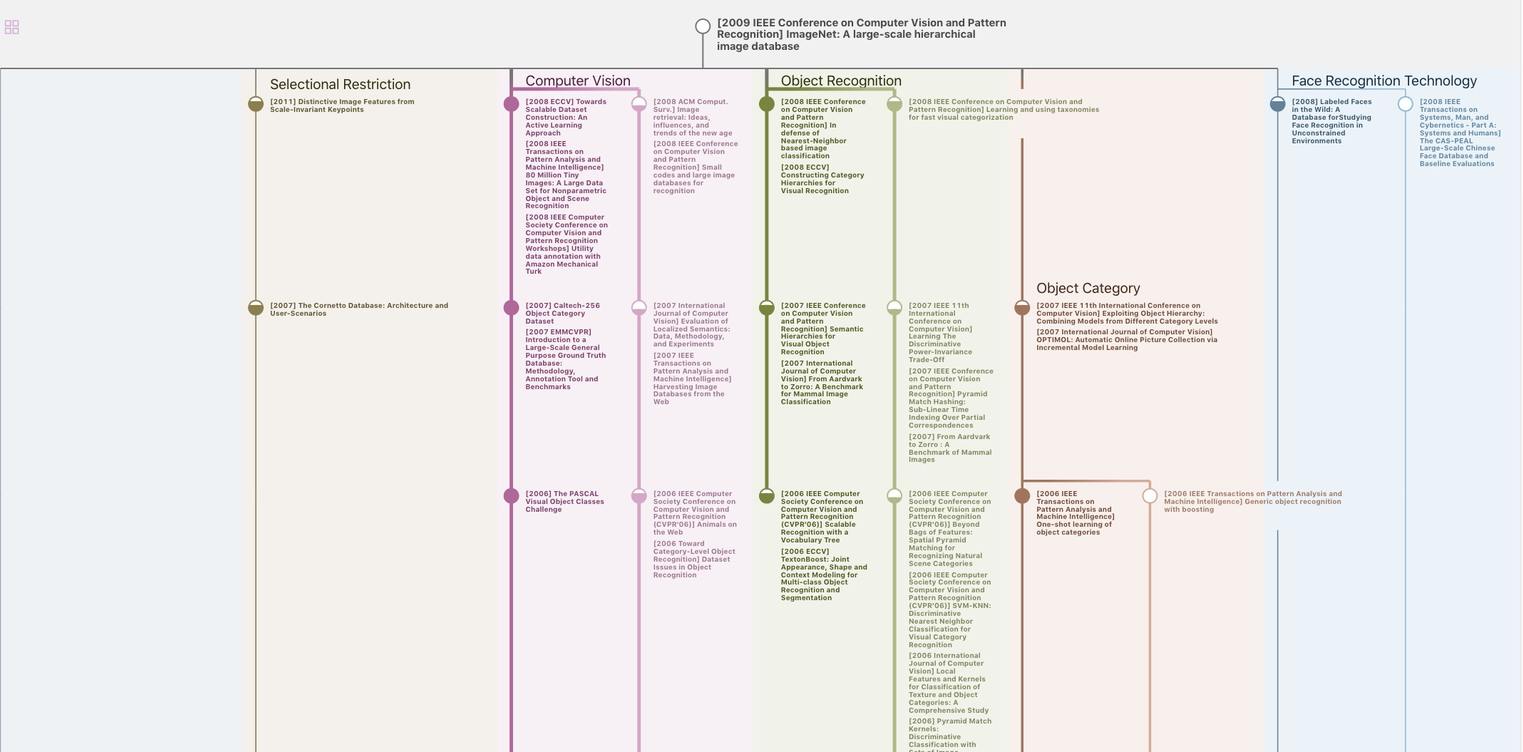
生成溯源树,研究论文发展脉络
Chat Paper
正在生成论文摘要