DRO: Deep Recurrent Optimizer for Video to Depth
arxiv(2023)
摘要
There are increasing interests of studying the video-to-depth (V2D) problem with machine learning techniques. While earlier methods directly learn a mapping from images to depth maps and camera poses, more recent works enforce multi-view geometry constraints through optimization embedded in the learning framework. This paper presents a novel optimization method based on recurrent neural networks to further exploit the potential of neural networks in V2D. Specifically, our neural optimizer alternately updates the depth and camera poses through iterations to minimize a feature-metric cost, and two gated recurrent units iteratively improve the results by tracing historical information. Extensive experimental results demonstrate that our method outperforms previous methods and is more efficient in computation and memory consumption than cost-volume-based methods. In particular, our self-supervised method outperforms previous supervised methods on the KITTI and ScanNet datasets. Our source code is available at https://github.com/aliyun/dro-sfm.
更多查看译文
关键词
Deep learning for visual perception,computer vision for transportation,visual learning
AI 理解论文
溯源树
样例
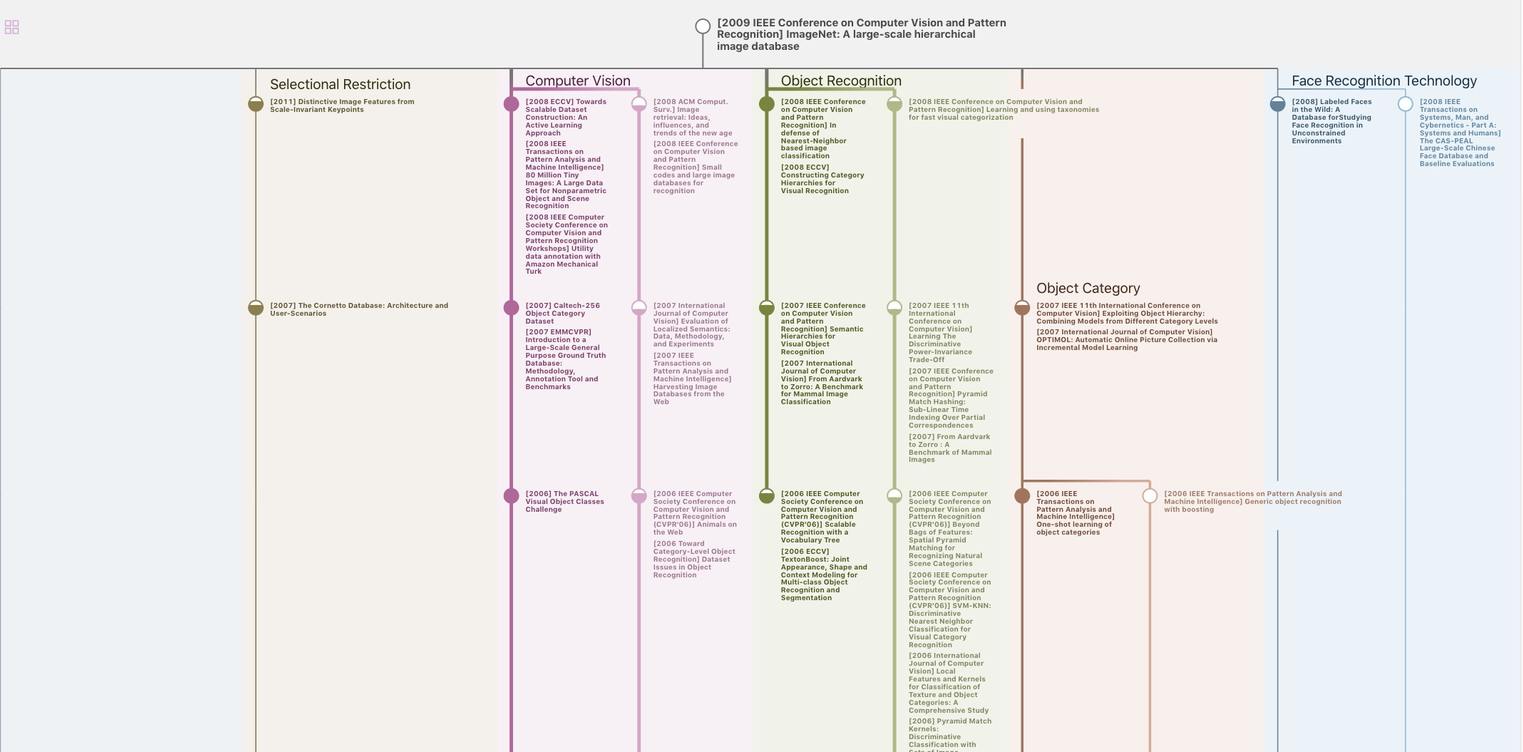
生成溯源树,研究论文发展脉络
Chat Paper
正在生成论文摘要