Thinking Aloud: Dynamic Context Generation Improves Zero-Shot Reasoning Performance of GPT-2
arxiv(2021)
摘要
Thinking aloud is an effective meta-cognitive strategy human reasoners apply to solve difficult problems. We suggest to improve the reasoning ability of pre-trained neural language models in a similar way, namely by expanding a task's context with problem elaborations that are dynamically generated by the language model itself. Our main result is that dynamic problem elaboration significantly improves the zero-shot performance of GPT-2 in a deductive reasoning and natural language inference task: While the model uses a syntactic heuristic for predicting an answer, it is capable (to some degree) of generating reasoned additional context which facilitates the successful application of its heuristic. We explore different ways of generating elaborations, including fewshot learning, and find that their relative performance varies with the specific problem characteristics (such as problem difficulty). Moreover, the effectiveness of an elaboration can be explained in terms of the degree to which the elaboration semantically coheres with the corresponding problem. In particular, elaborations that are most faithful to the original problem description may boost accuracy by up to 24%.
更多查看译文
关键词
dynamic context generation,aloud,zero-shot
AI 理解论文
溯源树
样例
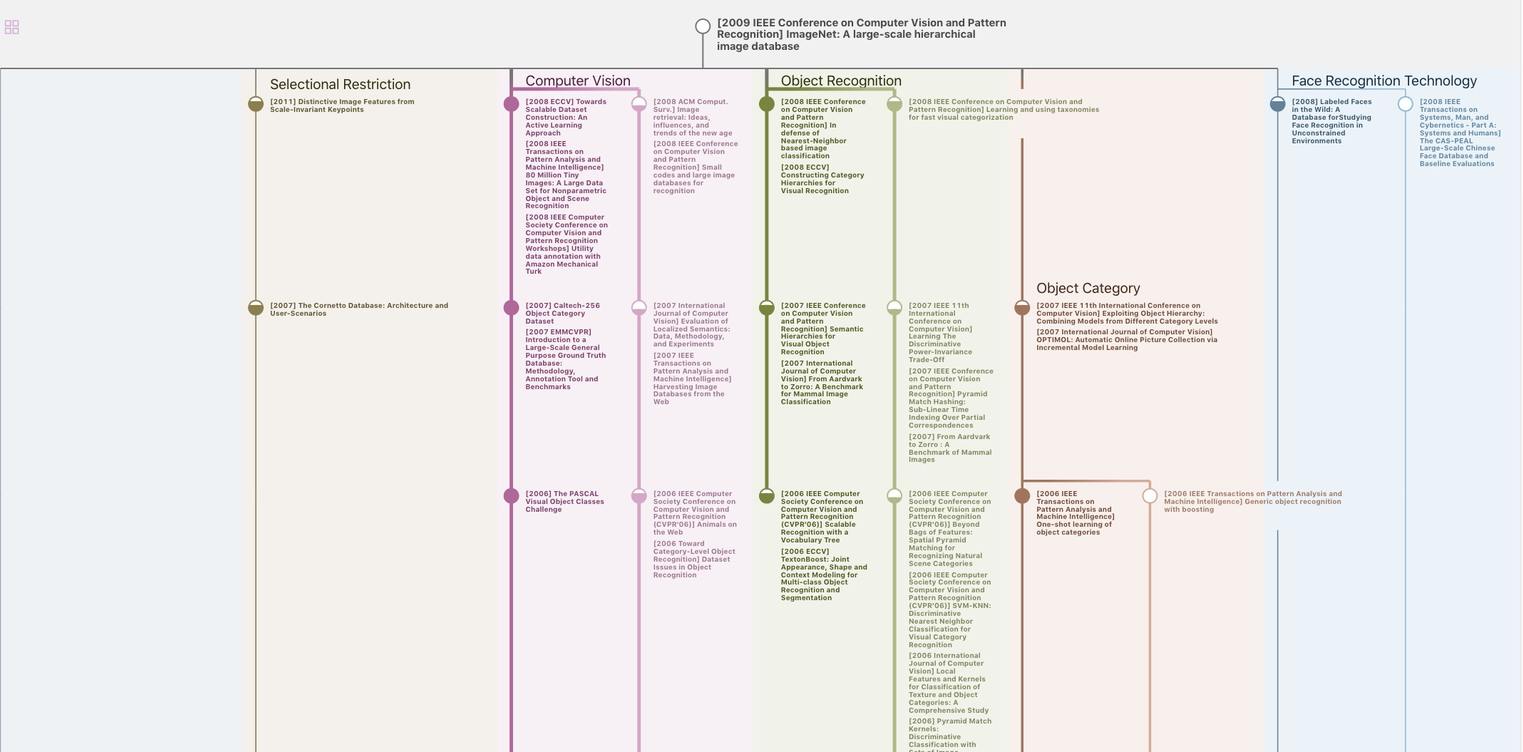
生成溯源树,研究论文发展脉络
Chat Paper
正在生成论文摘要