Promoting Fairness through Hyperparameter Optimization
2021 21ST IEEE INTERNATIONAL CONFERENCE ON DATA MINING (ICDM 2021)(2021)
摘要
Considerable research effort has been guided towards algorithmic fairness but real-world adoption of bias reduction techniques is still scarce. Existing methods are either metric-or model-specific, require access to sensitive attributes at inference time, or carry high development or deployment costs. This work explores the unfairness that emerges when optimizing ML models solely for predictive performance, and how to mitigate it with a simple and easily deployed intervention: fairness-aware hyperparameter optimization (HO). We propose and evaluate fairness-aware variants of three popular HO algorithms: Fair Random Search, Fair TPE, and Fairband. We validate our approach on a real-world bank account opening fraud case-study, as well as on three datasets from the fairness literature. Results show that, without extra training cost, it is feasible to find models with 111% mean fairness increase and just 6% decrease in performance when compared with fairness-blind HO.
1
更多查看译文
关键词
fairness,trustworthy AI,hyperparameter optimization,machine learning
AI 理解论文
溯源树
样例
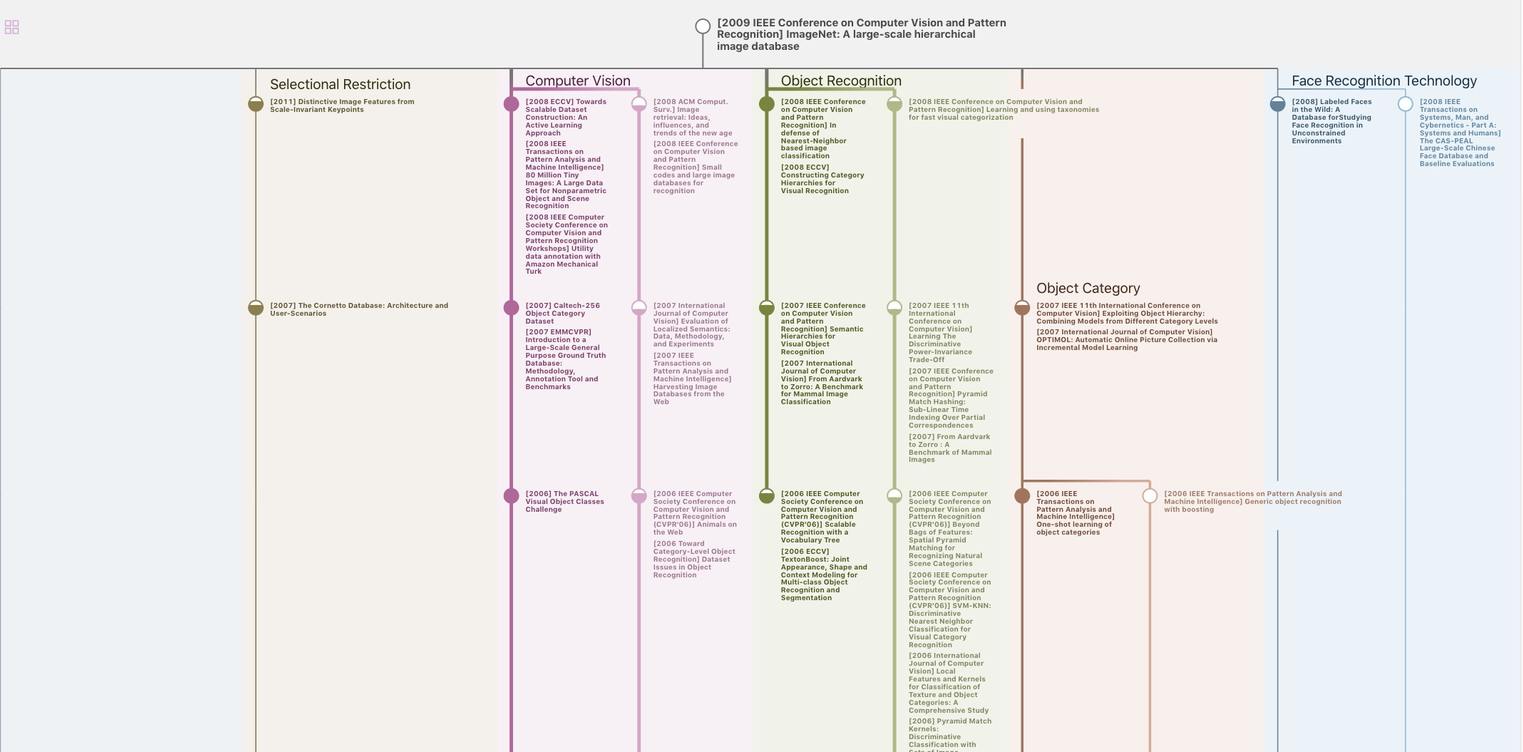
生成溯源树,研究论文发展脉络
Chat Paper
正在生成论文摘要