Residual Non-degenerate Temporal Network for Human Action Recognition
ieee international conference computer and communications(2020)
摘要
Recent research on video human action has progressed with the development of 3-demensional deep convolutional networks (3-D ConNets). In particular, spatiotemporal features exhibited improved performance. However, the temporal information, which commonly exists in video, has not been fully exploited in existing 3-D ConNets. In this paper, we propose a novel Residual Non-degenerate Temporal Network (RNTN) for human action recognition, which can exploit sufficiently temporal information from frames. Specially, RNTN mainly consists of residual nondegenerate temporal blocks (RNTB) and 3-D effective channel attention blocks (3D-ECA). In RNTB, the expression of temporal features is enhanced effectively. In 3D-ECA, the potential connection between features was strengthened by channel feature interactive with the adjacent channel features. Our approach provides the state-of-the-art performance on the datasets of UCF-101(98.33%) and HMDB-51(80.04%).
更多查看译文
关键词
action recognition,deep learning,3D convolution
AI 理解论文
溯源树
样例
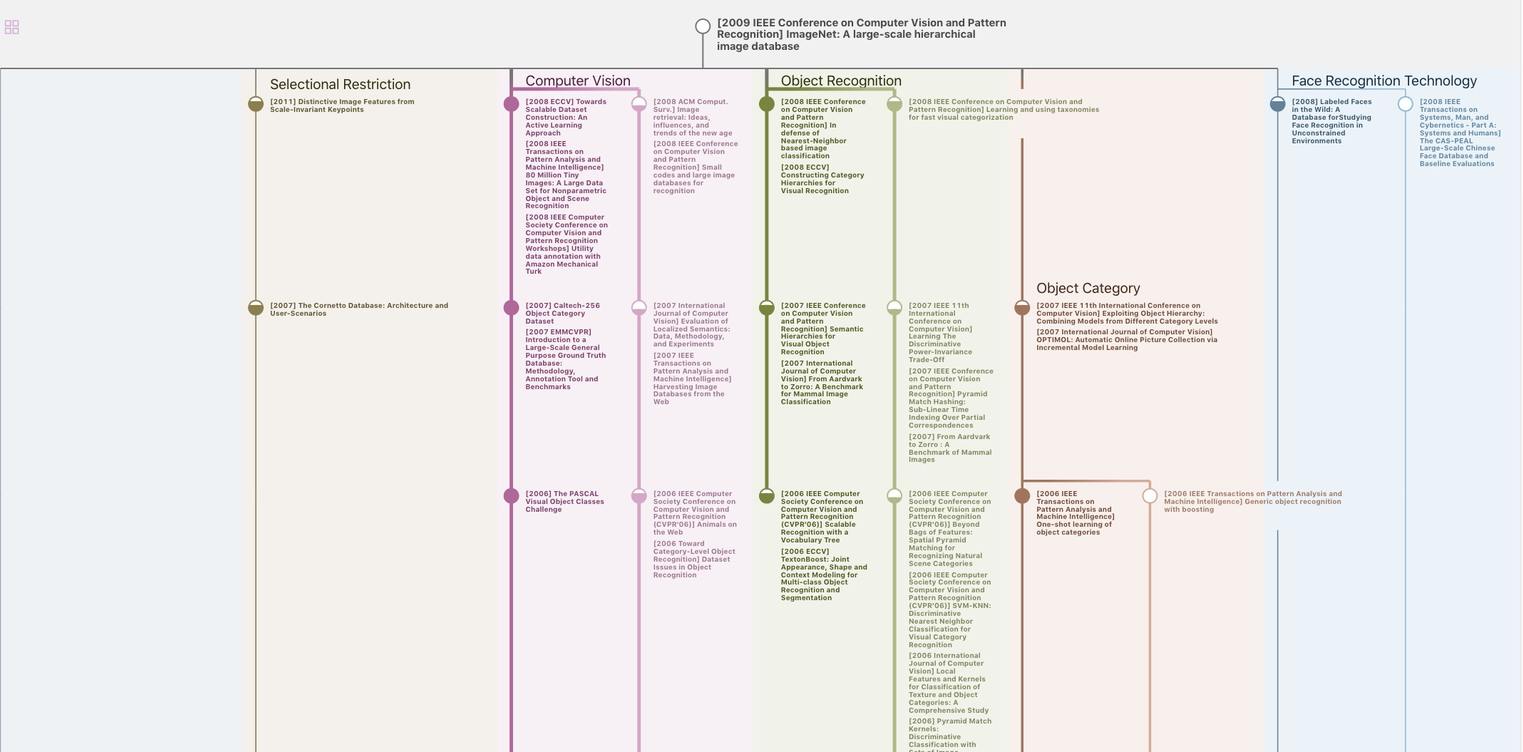
生成溯源树,研究论文发展脉络
Chat Paper
正在生成论文摘要