Efficient Dialog Policy Learning With Hindsight, User Modeling, and Adaptation
IEEE Transactions on Cognitive and Developmental Systems(2023)
摘要
Goal-oriented dialog systems aim to efficiently and accurately exchange information with people using natural language. A goal-oriented dialog policy is used for suggesting language actions for such dialog systems. Reinforcement learning (RL) has been used for computing dialog policies from the experience of language-based interaction. Learning efficiency is particularly important in dialog policy learning, due to the considerable cost of interacting with human users, and the potentially very poor user experience from low-quality conversations. In this article, we develop deep RL algorithms to improve the efficiency of dialog policy learning. Our contribution is threefold, aiming at the central goal of improving the efficiency of dialog policy learning. First, we present a novel "hindsight" approach to make use of unsuccessful dialog instances to provide the dialog learning agent with extra positive feedback. Second, we introduce user modeling, and enable the dialog agent to learn from simulated interaction experience. Third, we have developed a metalearning algorithm that enables the dialog agent to adaptively learn from simulated users and hindsight experience at the same time. The threefold contribution altogether, for the first time, enables our dialog agent outperforming a number of state-of-the-art dialog policy learning methods, as demonstrated via our experimental results.
更多查看译文
关键词
Deep learning,dialog systems,intrinsically motivated learning,metalearning,reinforcement learning (RL)
AI 理解论文
溯源树
样例
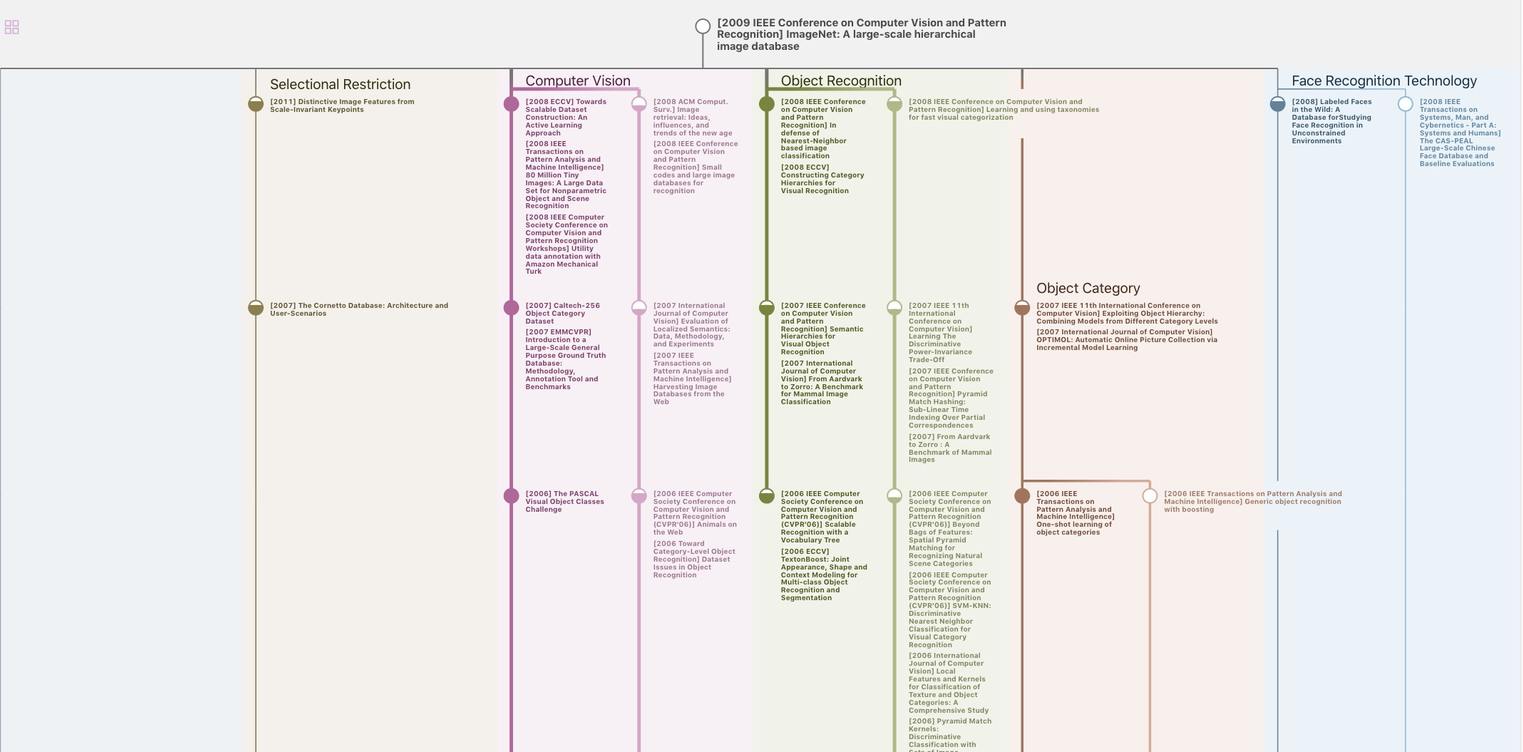
生成溯源树,研究论文发展脉络
Chat Paper
正在生成论文摘要