Margin Cosreid Network For Pedestrian Re-Identification
APPLIED SCIENCES-BASEL(2021)
摘要
This paper proposes a margin CosReid network for effective pedestrian re-identification. Aiming to overcome the overfitting, gradient explosion, and loss function non-convergence problems caused by traditional CNNs, the proposed GBNeck model can realize a faster, stronger generalization, and more discriminative feature extraction task. Furthermore, to enhance the classification ability of the softmax loss function within classes, the margin cosine softmax loss (MCSL) is proposed through a boundary margin introduction to ensure intraclass compactness and interclass separability of the learning depth features and thus to build a stronger metric-based learning model for pedestrian re-identification. The effectiveness of the margin CosReid network was verified on the mainstream datasets Market-1501 and DukeMTMC-reID compared with other state-of-the-art pedestrian re-identification methods.
更多查看译文
关键词
pedestrian re-identification, CNN, softmax loss
AI 理解论文
溯源树
样例
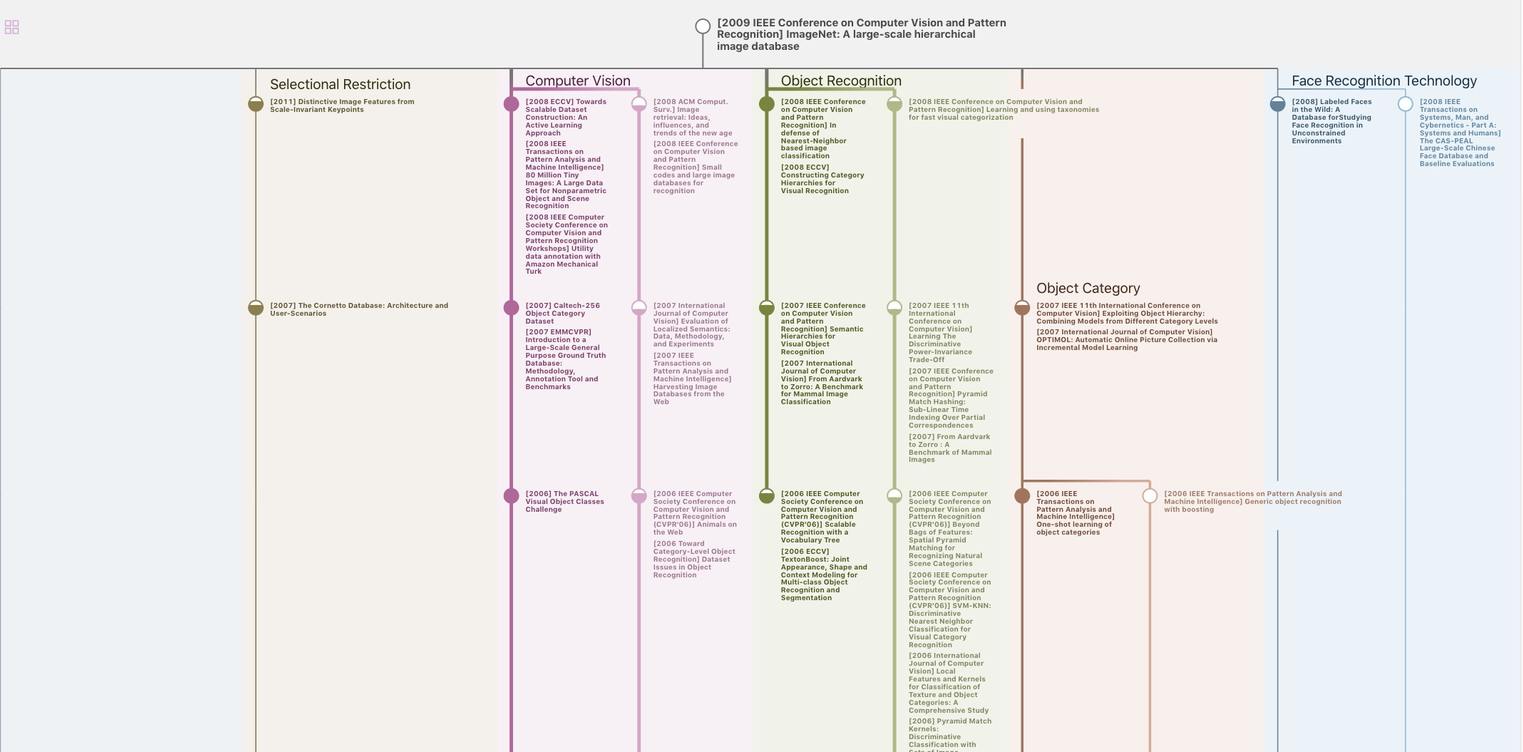
生成溯源树,研究论文发展脉络
Chat Paper
正在生成论文摘要