Development of a Dual-Attention U-Net Model for Sea Ice and Open Water Classification on SAR Images
IEEE GEOSCIENCE AND REMOTE SENSING LETTERS(2022)
摘要
This study develops a deep learning (DL) model to classify the sea ice and open water from synthetic aperture radar (SAR) images. We use the U-Net, a well-known fully convolutional network (FCN) for pixel-level segmentation, as the model backbone. We employ a DL-based feature extracting model, ResNet-34, as the encoder of the U-Net. To achieve high accuracy classifications, we integrate the dual-attention mechanism into the original U-Net to improve the feature representations, forming a dual-attention U-Net model (DAU-Net). The SAR images are obtained from Sentinel-1A. The dual-polarized information and the incident angle of SAR images are model inputs. We used 15 dual-polarized images acquired near the Bering Sea to train the model and employ the other three images to test the model. Experiments show that the DAU-Net could achieve pixel-level classification; the dual-attention mechanism can improve the classification accuracy. Compared with the original U-Net, DAU-Net improves the intersection over union (IoU) by 7.48.% points, 0.96.% points, and 0.83.% points on three test images. Compared with the recently published model DenseNetFCN, the three improvement IoU values of DAU-Net are 3.04.% points, 2.53.% points, and 2.26.% points, respectively.
更多查看译文
关键词
Sea ice, Radar polarimetry, Feature extraction, Decoding, Oceans, Kernel, Image segmentation, Dual-attention, sea ice and open water classification, synthetic aperture radar (SAR) image, U-Net
AI 理解论文
溯源树
样例
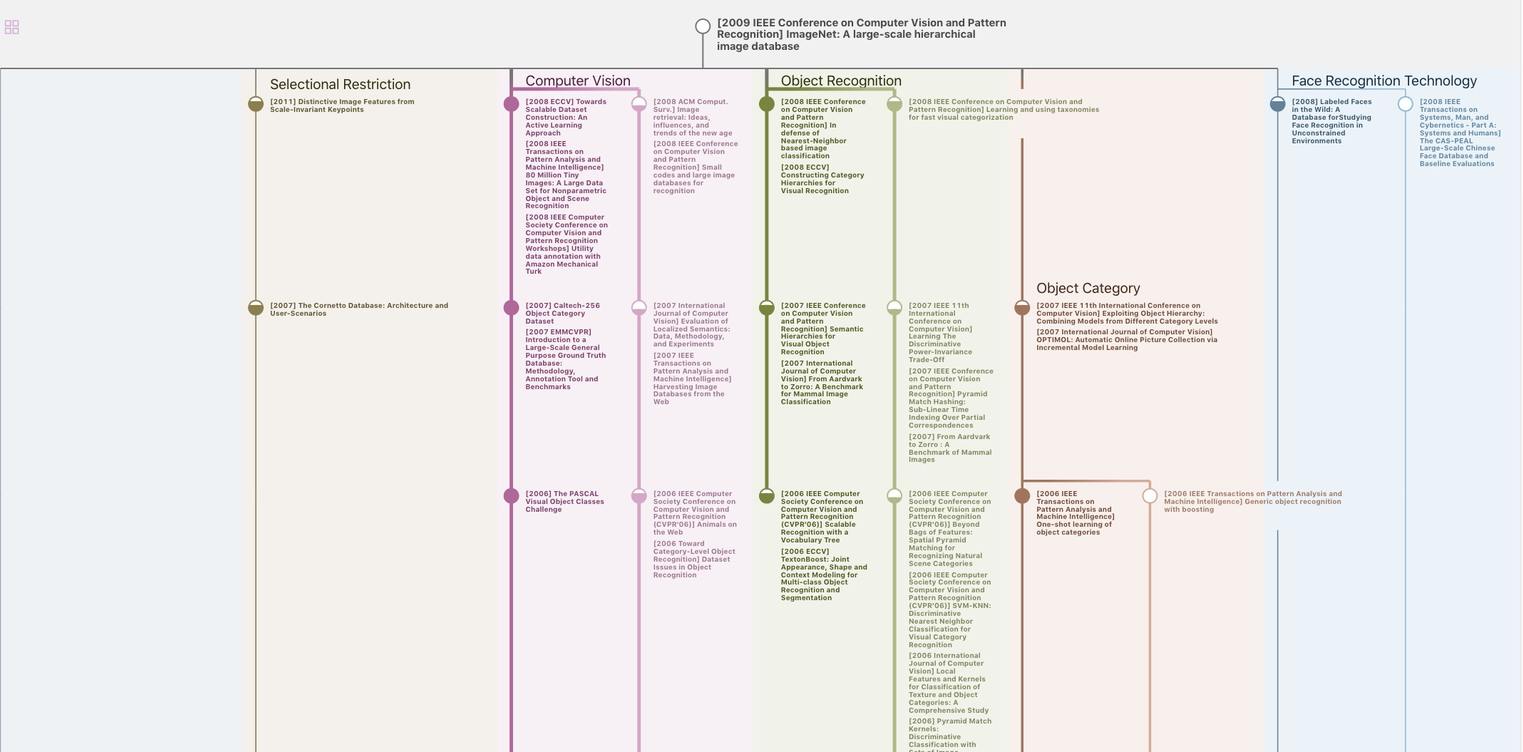
生成溯源树,研究论文发展脉络
Chat Paper
正在生成论文摘要