Spectral Decomposition For Graded Multi-Scale Topology Optimization
COMPUTER METHODS IN APPLIED MECHANICS AND ENGINEERING(2021)
摘要
Multi-scale topology optimization (MTO) is exploited today in applications that require designs with large surface-to-volume ratio. Further, with the advent of additive manufacturing, MTO has gained significant prominence. However, a major drawback of MTO is that it is computationally expensive. As an alternate, graded MTO has been proposed where the design features at the smaller scale are graded variations of a single microstructure. This leads to significant reduction in computational cost, while retaining many of the benefits of MTO.Graded MTO fundamentally rests on interpolation of elasticity matrices. The direct method of interpolation used today unfortunately does not guarantee positive-definiteness of the resulting matrices. Consequently, during the graded MTO algorithm the strain energy may become negative and non-physical.In this paper, we propose a simple but effective spectral decomposition-based approach which guarantees positive-definite elasticity matrices. The proposed method relies on a spectral (eigen) decomposition of instances of the elasticity matrices, followed by regression of eigenvalues and interpolation of eigenvector orientations. The resulting elasticity matrix can then be used for stable optimization. The direct and spectral decomposition methods are compared here for robustness, accuracy and speed, through several numerical experiments. (C) 2021 Elsevier B.V. All rights reserved.
更多查看译文
关键词
Spectral decomposition, Elasticity matrix, Lattice, Multi-scale topology optimization, Asymptotic homogenization
AI 理解论文
溯源树
样例
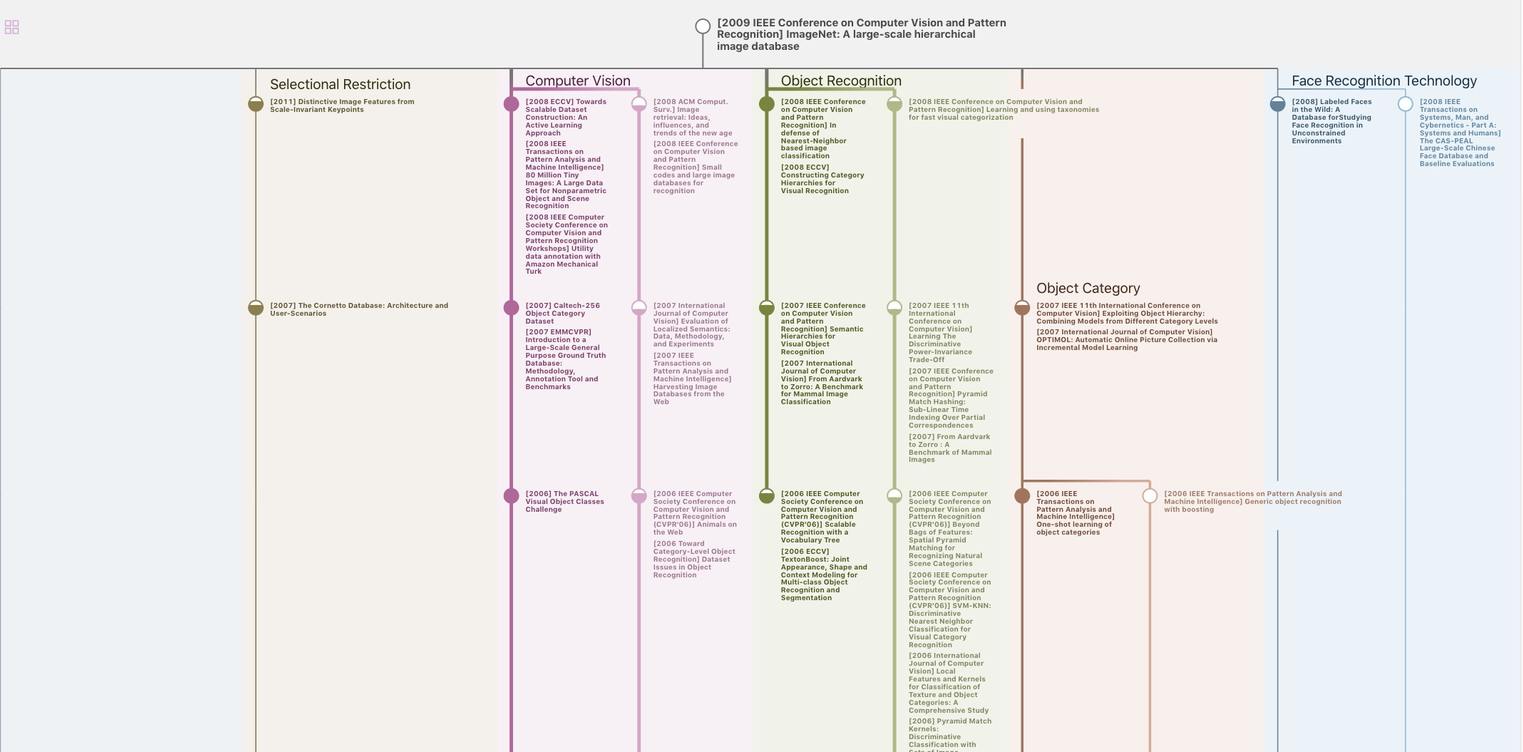
生成溯源树,研究论文发展脉络
Chat Paper
正在生成论文摘要