FLAS: Computation and Communication Efficient Federated Learning via Adaptive Sampling
IEEE Transactions on Network Science and Engineering(2022)
摘要
Federated learning enables collaborative deep learning over multiple clients without sharing their local data, and it becomes increasingly popular due to the good balance between data privacy and model usability. Generally, it faces the heavy communication overhead when a large number of clients are involved and the low convergence rate incurred by non-IID data. However, few existing solutions cannot simultaneously address the communication and statistic challenges. In this paper, we propose a computation and communication efficient federated learning via adaptive sampling. By capturing different data distribution among clients, we utilize the concept of self-paced learning to adaptively adjust thresholds to filter training data for each client and also to select suitable clients to be involved in each global learning round. We prove its correctness through theoretical analysis and also evaluate its performance through experimental evaluations on real-world datasets. Detailed experimental results show that it can effectively reduce the communication cost while achieving the good trade-off between accuracy and efficiency.
更多查看译文
关键词
Adaptive threshold,client sampling,data sampling,federated learning,self-paced learning
AI 理解论文
溯源树
样例
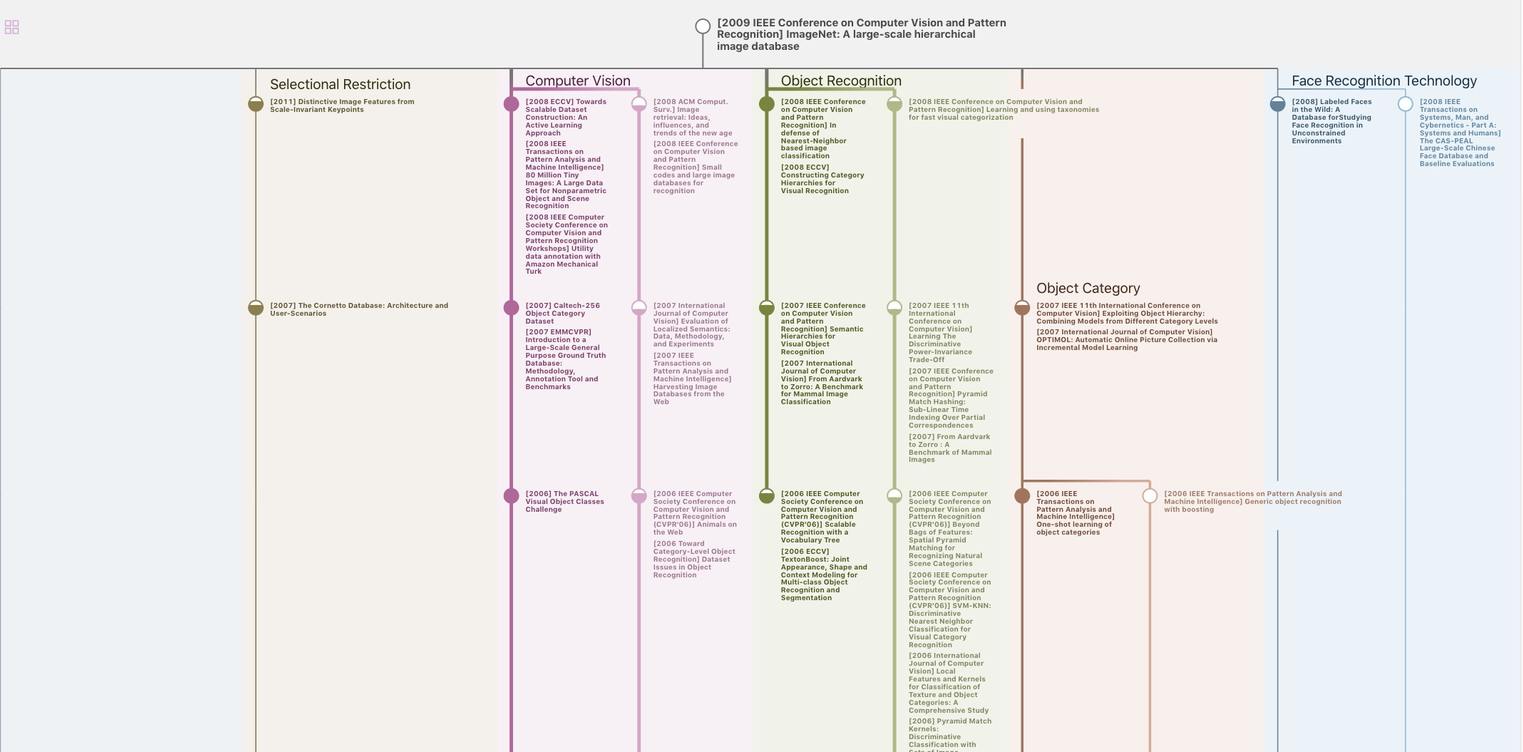
生成溯源树,研究论文发展脉络
Chat Paper
正在生成论文摘要