Multi-Label Classification of Fundus Images With Graph Convolutional Network and Self-Supervised Learning
IEEE Signal Processing Letters(2021)
摘要
The accurate diagnosis of fundus disease can effectively reduce the disease's further deterioration and provide targeted treatment plans for patients. Fundus image classification is a multi-label classification task due to one fundus image may contain one or more diseases. For multi-label classification of fundus images, we propose two new multi-label classification networks -- MCG-Net based on graph convolutional network and MCGS-Net based on graph convolutional network and self-supervised learning. Here, the graph convolutional network is used to capture the relevant information of the multi-label fundus images, and self-supervised learning is used to enhance the generalization ability of the network by learning more unannotated data. We use the ROC curve, Precision score, Recall score, Kappa score, F-1 score, and AUC value as the evaluation metrics and test on two datasets. Compared with other methods, our methods have better classification performance and generalization ability. Our methods can significantly improve classification performance and enhance the generalization ability of multi-label fundus image classification.
更多查看译文
关键词
Fundus images,graph convolutional network,multi-label classification,self-supervised learning
AI 理解论文
溯源树
样例
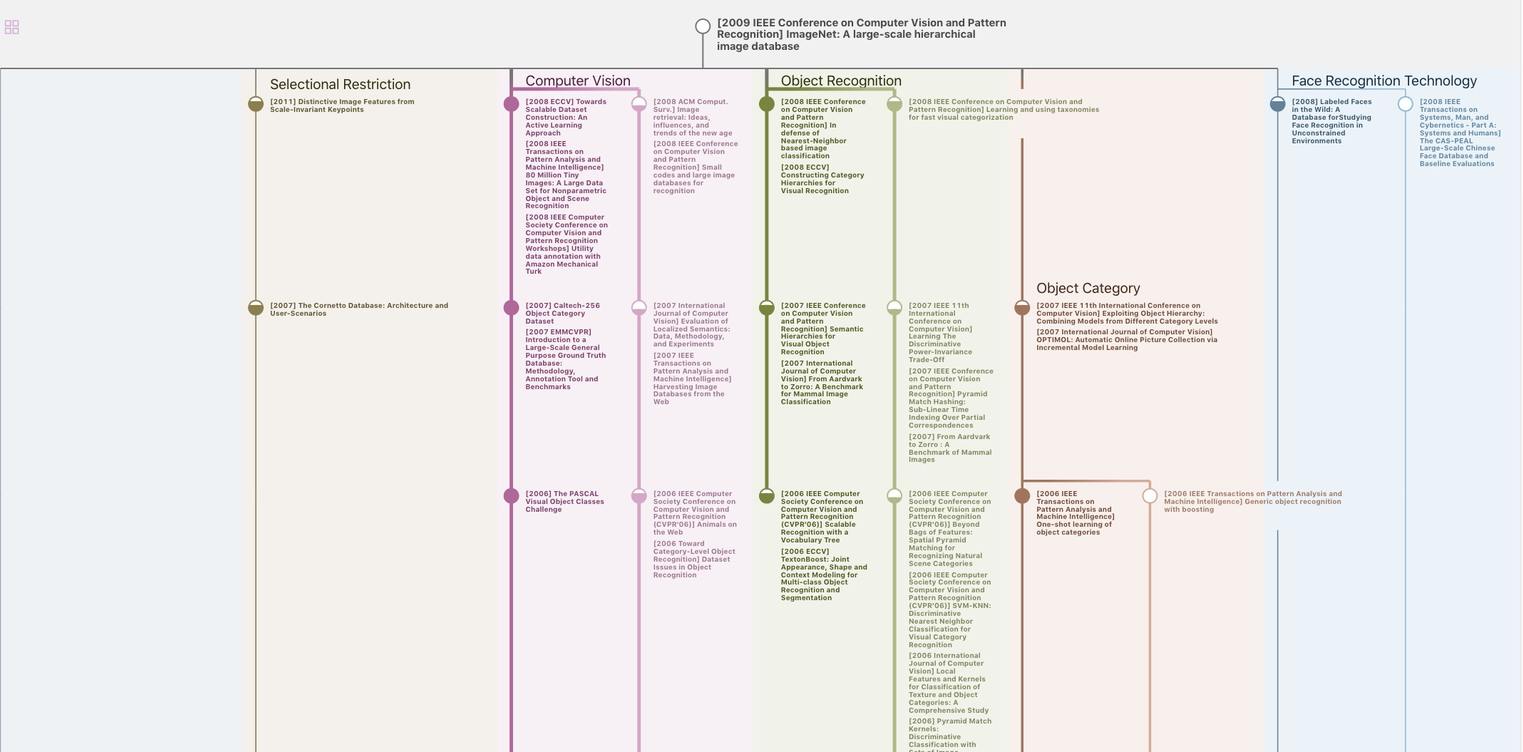
生成溯源树,研究论文发展脉络
Chat Paper
正在生成论文摘要