Echocardiographic phenotyping of the continuum of myocardial functional remodelling in left ventricular hypertrophy - machine learning validated with cardiac magnetic resonance
European Journal of Echocardiography(2021)
摘要
Abstract Funding Acknowledgements Type of funding sources: Public grant(s) – EU funding. Main funding source(s): Horizon 2020 European Commission Project H2020-MSCA-ITN-2016 (764738) and the Clinical Research in Cardiology grant from the Spanish Cardiac Society Background Exploring phenotypes of left ventricular hypertrophy (LVH) and interpreting the relationship of genotype and phenotype are contemporary clinical challenges. Machine learning (ML) can help by integrating whole-cardiac cycle echo data and separating patients based on subtle differences of cardiac function. The aim is to investigate if an unsupervised ML approach has the potential to explore the LVH spectrum and recognize phenotypes related to distinct disease aetiologies and genotypes. Methods The cohort consisted of 342 participants: patients with hypertrophic cardiomyopathy (HCM)(n = 27), HCM relatives (n = 31), hypertensive patients (HTN) (n = 189), and healthy individuals (n = 95). All had echocardiography performed, whereas magnetic resonance (MR) and genetic testing were performed when clinically indicated. Myocardial deformation of the LV and left atrium, aortic and mitral blood-pool Doppler, as well as the septal mitral annular tissue Doppler velocity profiles were used as input for ML. Clinical data, including echo measurements, were not part of the learning, but used to validate the ML-derived phenotypes. An unsupervised ML algorithm was used to create an output space where participants were positioned based on cardiac function. Regression was used to estimate the echo and clinical characteristics of different regions in the space. Results The ML analysis of HCM and relative data shows grouping of HCM patients in the right-most region of the output space (Fig 1B). This region was related to LV outflow tract obstruction, mitral inflow fusion, systolic impairment with septal involvement, as well as LA and LV strain impairment (Fig 1A). Clinical data concurred - showing reduced global longitudinal strain, elevated LV mass, and a pattern of systolic and diastolic impairment - defining a comprehensive phenotype of LV remodelling related to HCM. Exploration of the genotype/phenotype relationship revealed G + P- relatives grouping on the transition from the healthy to the remodelling region. Projection of the HTN and healthy individuals into the HCM space defined the LVH disease spectrum, with healthy individuals projecting in the existing healthy region and HTNs in the transition from health to extreme remodelling (Fig 1C). MR findings of late gadolinium enhancement correlated with the ML-derived functional remodelling phenotype (Fig 1C). Furthermore, 6 patients with a clinical need for septal myectomy were located in the extreme remodelling part of the output space (Fig 1C, red circles). Conclusion ML can integrate complex, whole-cardiac cycle echo data to group patients based on similarity of cardiac function. Using an interpretable ML approach, we can explore the spectrum of LV remodelling in different aetiologies and interpret the relationship between genotype and phenotype. The methodology can accommodate new patients by projecting them into the existing space to aid in clinical interpretation, risk assessment and patient management. Abstract Figure 1
更多查看译文
AI 理解论文
溯源树
样例
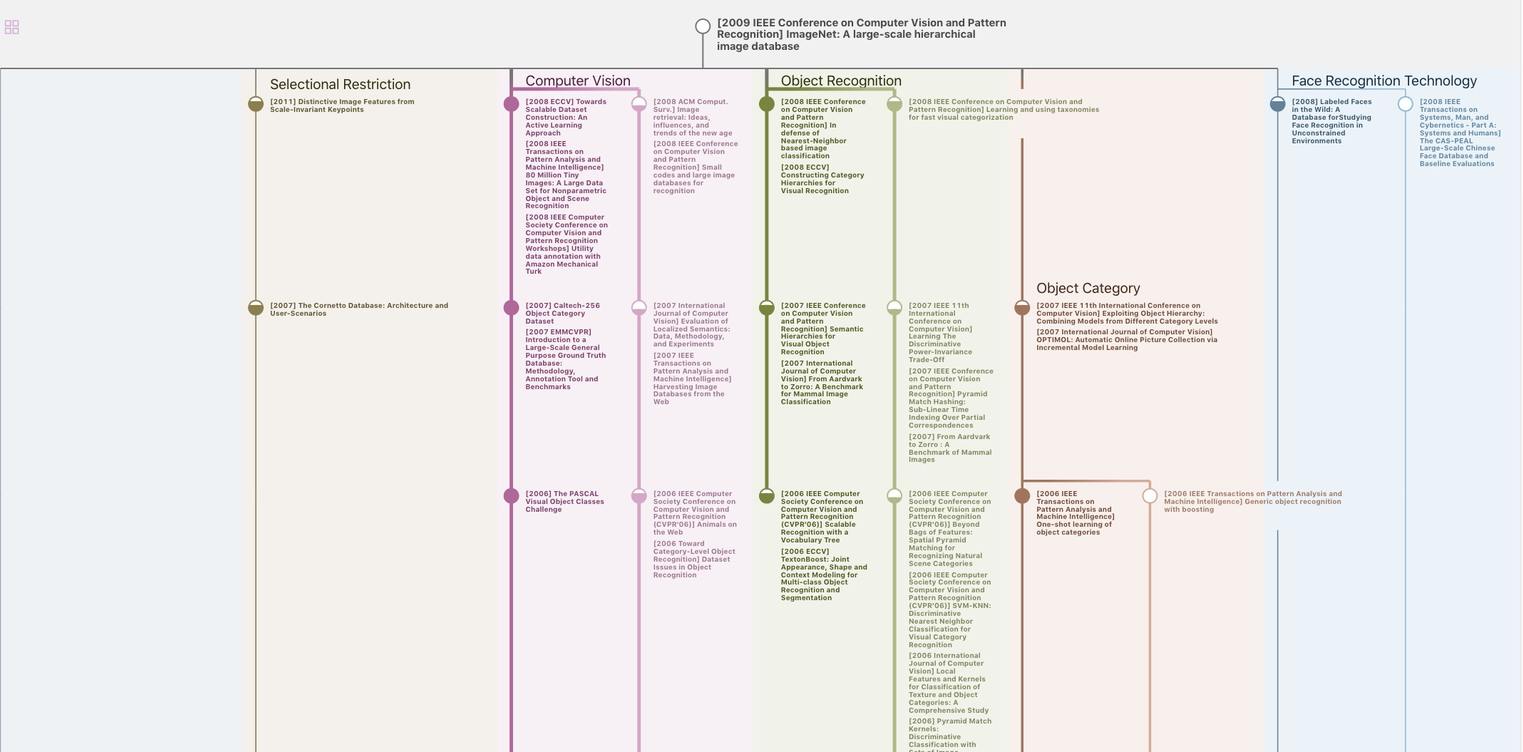
生成溯源树,研究论文发展脉络
Chat Paper
正在生成论文摘要