An Optimized Cnn-Based Quality Assessment Model For Screen Content Image
SIGNAL PROCESSING-IMAGE COMMUNICATION(2021)
摘要
Most existing convolutional neural network (CNN) based models designed for natural image quality assessment (IQA) employ image patches as training samples for data augmentation, and obtain final quality score by averaging all predicted scores of image patches. This brings two problems when applying these methods for screen content image (SCI) quality assessment. Firstly, SCI contains more complex content compared to natural image. As a result, qualities of SCI patches are different, and the subjective differential mean opinion score (DMOS) is not appropriate as qualities of all image patches. Secondly, the average score of image patches does not represent the quality of entire SCI since the human visual system (HVS) is sensitive to image patches containing texture and edge information. In this paper, we propose a novel quadratic optimized model based on the deep convolutional neural network (QODCNN) for full-reference (FR) and no-reference (NR) SCI quality assessment to overcome these two problems. The contribution of our algorithm can be concluded as follows: 1) Considering the characteristics of SCIs, a valid network architecture is designed for both NR and FR visual quality evaluation of SCIs, which makes the networks learn the feature differences for FR-IQA; 2) with the consideration of correlation between local quality and DMOS, a training data selection method is proposed to fine-tune the pre-trained model with valid SCI patches; 3) an adaptive pooling approach is employed to fuse patch quality to obtain image quality, owns strong noise robust and effects on both FR and NR IQA. Experimental results verify that our model outperforms both current no-reference and full-reference image quality assessment methods on the benchmark screen content image quality assessment database (SIQAD). Cross-database evaluation shows high generalization ability and high effectiveness of our model.
更多查看译文
关键词
Image quality assessment, Screen content image, No-reference, Full-reference, Convolutional neural network, Quality pooling, Data selection
AI 理解论文
溯源树
样例
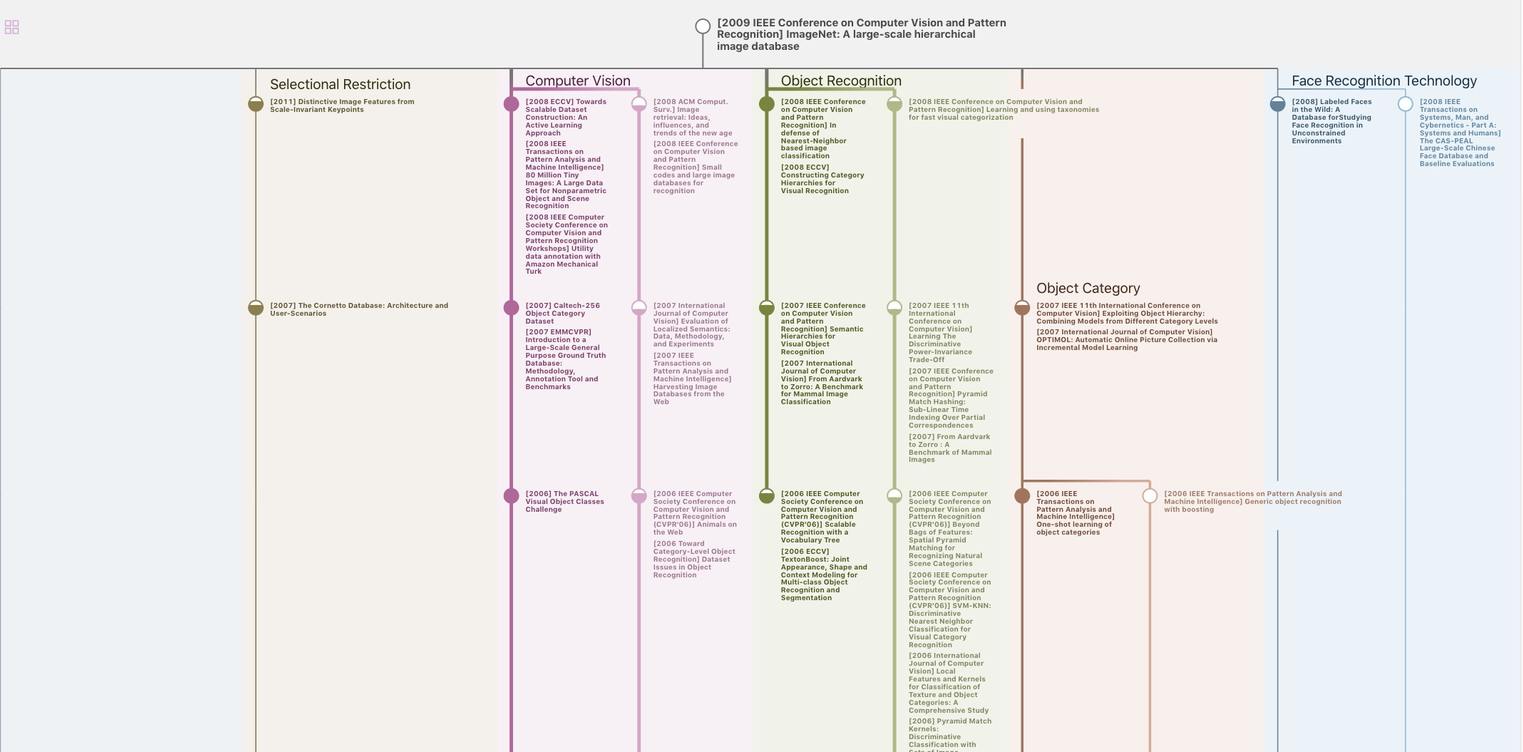
生成溯源树,研究论文发展脉络
Chat Paper
正在生成论文摘要