MaAST: Map Attention with Semantic Transformers for Efficient Visual Navigation
2021 IEEE INTERNATIONAL CONFERENCE ON ROBOTICS AND AUTOMATION (ICRA 2021)(2021)
摘要
Visual navigation for autonomous agents is a core task in the fields of computer vision and robotics. Learning-based methods, such as deep reinforcement learning, have the potential to outperform the classical solutions developed for this task; however, they come at a significantly increased computational load. Through this work, we design a novel approach that focuses on performing better or comparable to the existing learning-based solutions but under a clear time/computational budget. To this end, we propose a method to encode vital scene semantics such as traversable paths, unexplored areas, and observed scene objects-alongside raw visual streams such as KGB, depth, and semantic segmentation masks-into a semantically informed, top-down egocentric map representation. Further, to enable the effective use of this information, we introduce a novel 2-D map attention mechanism, based on the successful multi-layer Transformer networks. We conduct experiments on 3-D reconstructed indoor PointGoal visual navigation and demonstrate the effectiveness of our approach. We show that by using our novel attention schema and auxiliary rewards to better utilize scene semantics, we outperform multiple baselines trained with only raw inputs or implicit semantic information while operating with an 80% decrease in the agent's experience.
更多查看译文
关键词
egocentric map representation,map attention,multilayer Transformer networks,3-D reconstructed indoor PointGoal visual navigation,attention schema,utilize scene semantics,implicit semantic information,agent,MaAST,semantic transformers,efficient visual navigation,autonomous agents,core task,computer vision,robotics,learning-based methods,deep reinforcement learning,classical solutions,increased computational load,existing learning-based solutions,vital scene semantics,traversable paths,unexplored areas,scene objects-alongside raw visual streams,semantic segmentation masks
AI 理解论文
溯源树
样例
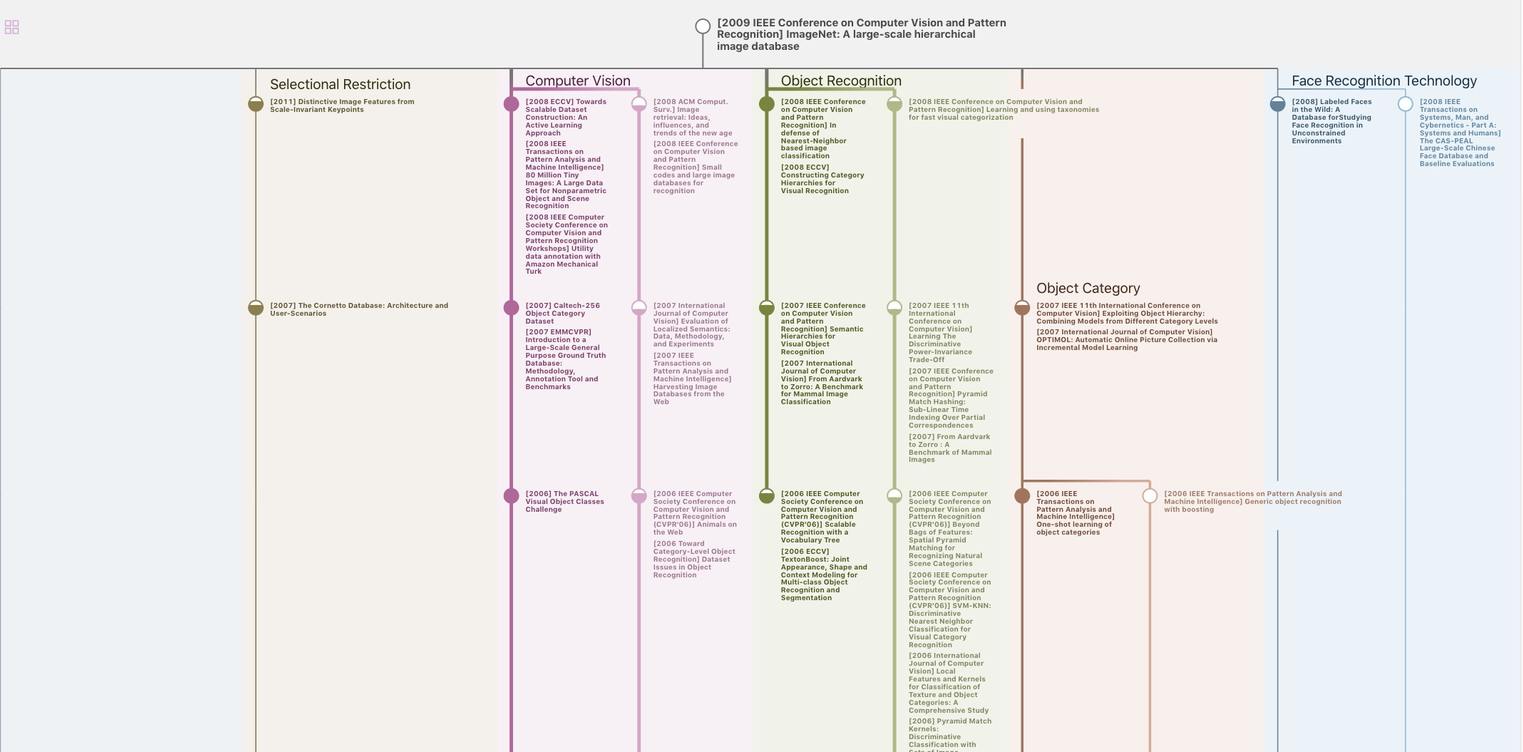
生成溯源树,研究论文发展脉络
Chat Paper
正在生成论文摘要