Learning Continuous Cost-to-Go Functions for Non-holonomic Systems
2021 IEEE/RSJ INTERNATIONAL CONFERENCE ON INTELLIGENT ROBOTS AND SYSTEMS (IROS)(2021)
摘要
This paper presents a supervised learning method to generate continuous cost-to-go functions of non-holonomic systems directly from the workspace description. Supervision from informative examples reduces training time and improves network performance. The manifold representing the optimal trajectories of a non-holonomic system has high-curvature regions which can not be efficiently captured with uniform sampling. To address this challenge, we present an adaptive sampling method which makes use of sampling based planners along with local, closed-form solutions to generate training samples. The cost-to-go function over a specific workspace is represented as a neural network whose weights are generated by a second, higher order network. The networks are trained in an end-to-end fashion. In our previous work, this architecture was shown to successfully learn to generate the cost-to-go functions of holonomic systems using uniform sampling. In this work, we show that uniform sampling fails for non-holonomic systems. However, with the proposed adaptive sampling methodology, our network can generate near-optimal trajectories for non-holonomic systems while avoiding obstacles. Experiments show that our method is two orders of magnitude faster compared to traditional approaches in cluttered environments.
更多查看译文
关键词
learning,systems,functions,cost-to-go,non-holonomic
AI 理解论文
溯源树
样例
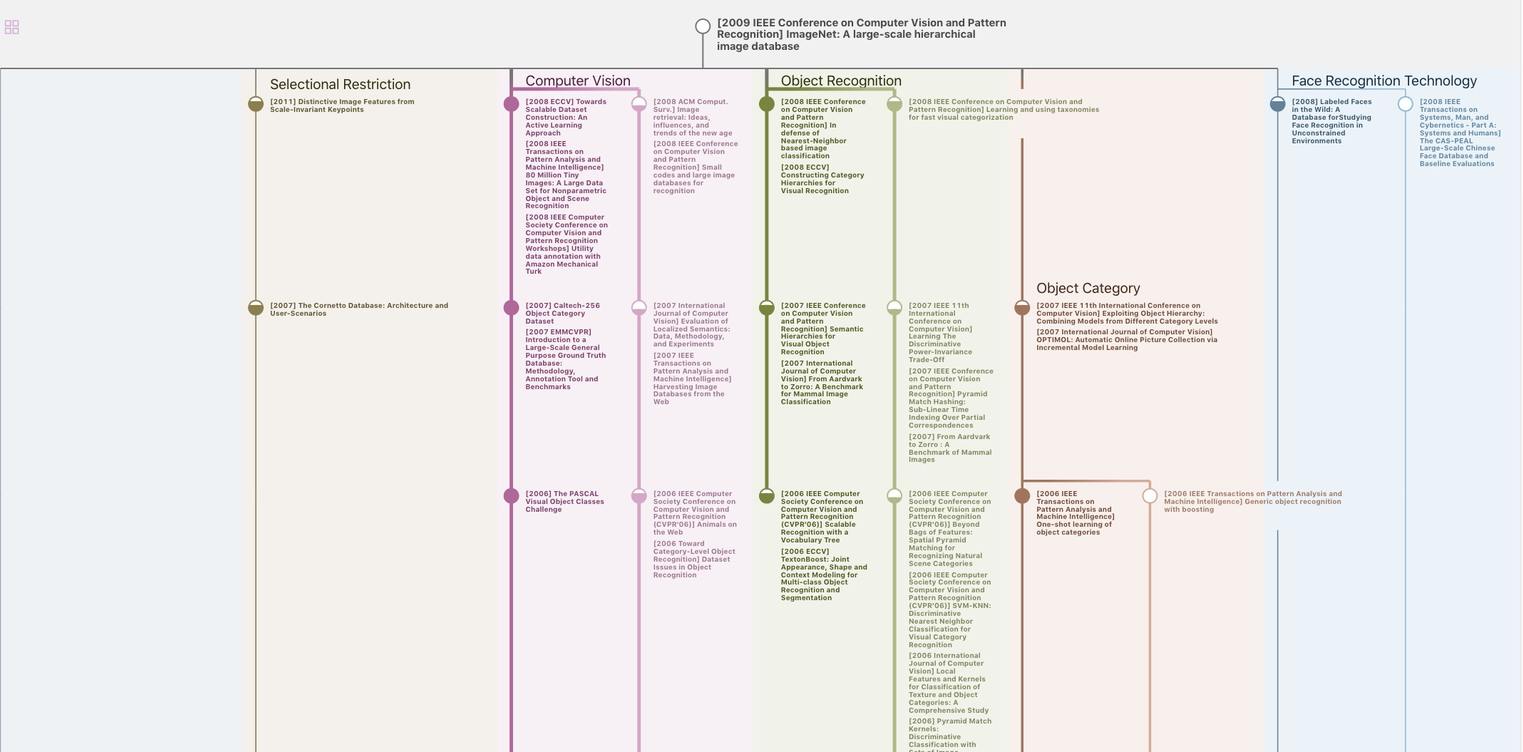
生成溯源树,研究论文发展脉络
Chat Paper
正在生成论文摘要