Accelerated Multicontrast Reconstruction For Synthetic Mri Using Joint Parallel Imaging And Variable Splitting Networks
MEDICAL PHYSICS(2021)
摘要
Purpose: Synthetic magnetic resonance imaging (MRI) requires the acquisition of multicontrast images to estimate quantitative parameter maps, such as T-1, T-2, and proton density (PD). The study aims to develop a multicontrast reconstruction method based on joint parallel imaging (JPI) and joint deep learning (JDL) to enable further acceleration of synthetic MRI.Methods: The JPI and JDL methods are extended and combined to improve reconstruction for better-quality, synthesized images. JPI is performed as a first step to estimate the missing k-space lines, and JDL is then performed to correct and refine the previous estimate with a trained neural network. For the JDL architecture, the original variable splitting network (VS-Net) is modified and extended to form a joint variable splitting network (JVS-Net) to apply to multicontrast reconstructions. The proposed method is designed and tested for multidynamic multiecho (MDME) images with Cartesian uniform under-sampling using acceleration factors between 4 and 8.Results: It is demonstrated that the normalized root-mean-square error (nRMSE) is lower and the structural similarity index measure (SSIM) values are higher with the proposed method compared to both the JPI and JDL methods individually. The method also demonstrates the potential to produce a set of synthesized contrast-weighted images that closely resemble those from the fully sampled acquisition without erroneous artifacts.Conclusion: Combining JPI and JDL enables the reconstruction of highly accelerated synthetic MRIs. (C) 2021 American Association of Physicists in Medicine
更多查看译文
关键词
deep learning, multicontrast, parallel imaging, synthetic MRI
AI 理解论文
溯源树
样例
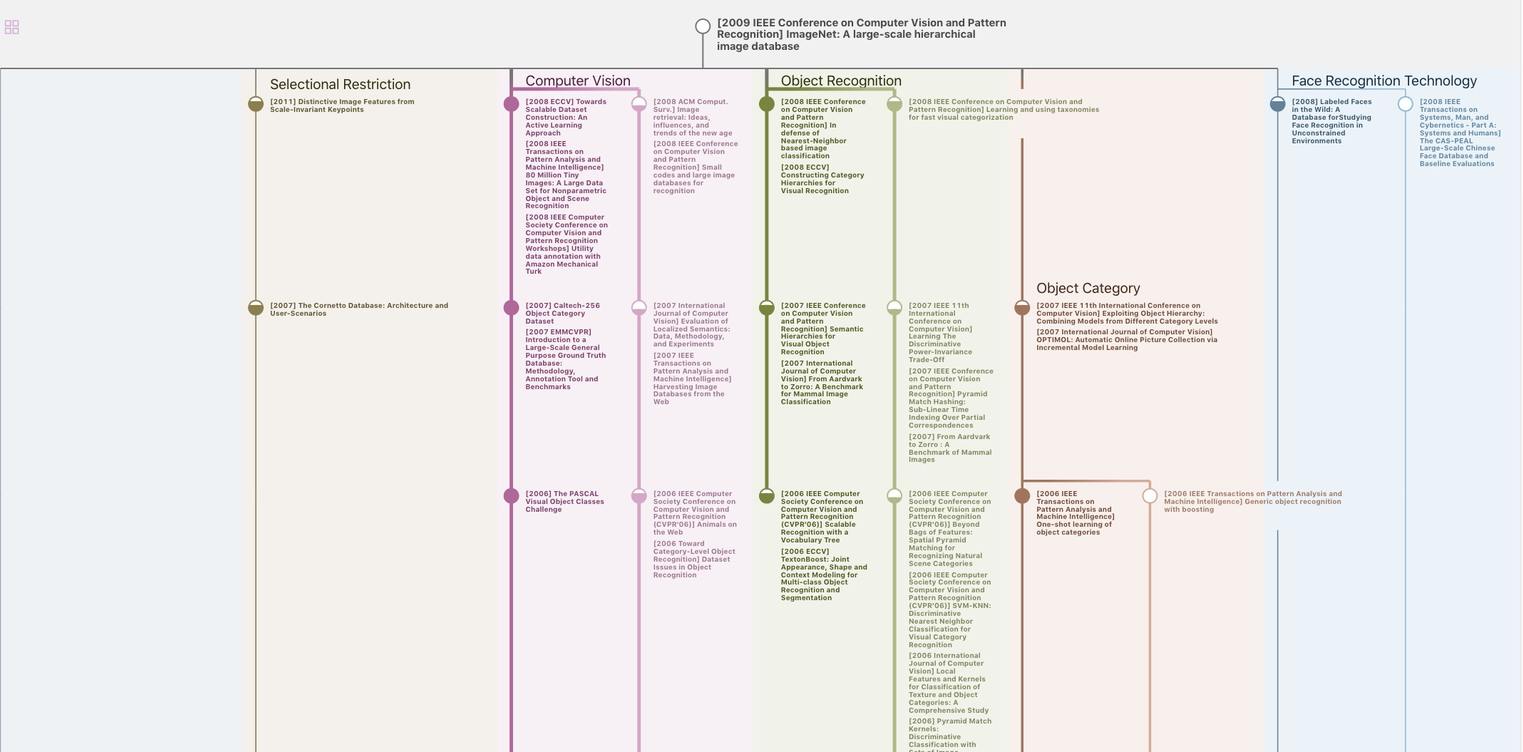
生成溯源树,研究论文发展脉络
Chat Paper
正在生成论文摘要