Max-Margin Deep Diverse Latent Dirichlet Allocation With Continual Learning
IEEE Transactions on Cybernetics(2022)
摘要
Deep probabilistic aspect models are widely utilized in document analysis to extract the semantic information and obtain descriptive topics. However, there are two problems that may affect their applications. One is that common words shared among all documents with low representational meaning may reduce the representation ability of learned topics. The other is introducing supervision information to hierarchical topic models to fully utilize the side information of documents that is difficult. To address these problems, in this article, we first propose deep diverse latent Dirichlet allocation (DDLDA), a deep hierarchical topic model that can yield more meaningful semantic topics with less common and meaningless words by introducing shared topics. Moreover, we develop a variational inference network for DDLDA, which helps us to further generalize DDLDA to a supervised deep topic model called max-margin DDLDA (mmDDLDA) by employing max-margin principle as the classification criterion. Compared to DDLDA, mmDDLDA can discover more discriminative topical representations. In addition, a continual hybrid method with stochastic-gradient MCMC and variational inference is put forward for deep latent Dirichlet allocation (DLDA)-based models to make them more practical in real-world applications. The experimental results demonstrate that DDLDA and mmDDLDA are more efficient than existing unsupervised and supervised topic models in discovering highly discriminative topic representations and achieving higher classification accuracy. Meanwhile, DLDA and our proposed models trained by the proposed continual learning approach cannot only show good performance on preventing catastrophic forgetting but also fit the evolving new tasks well.
更多查看译文
关键词
Models, Statistical,Semantics
AI 理解论文
溯源树
样例
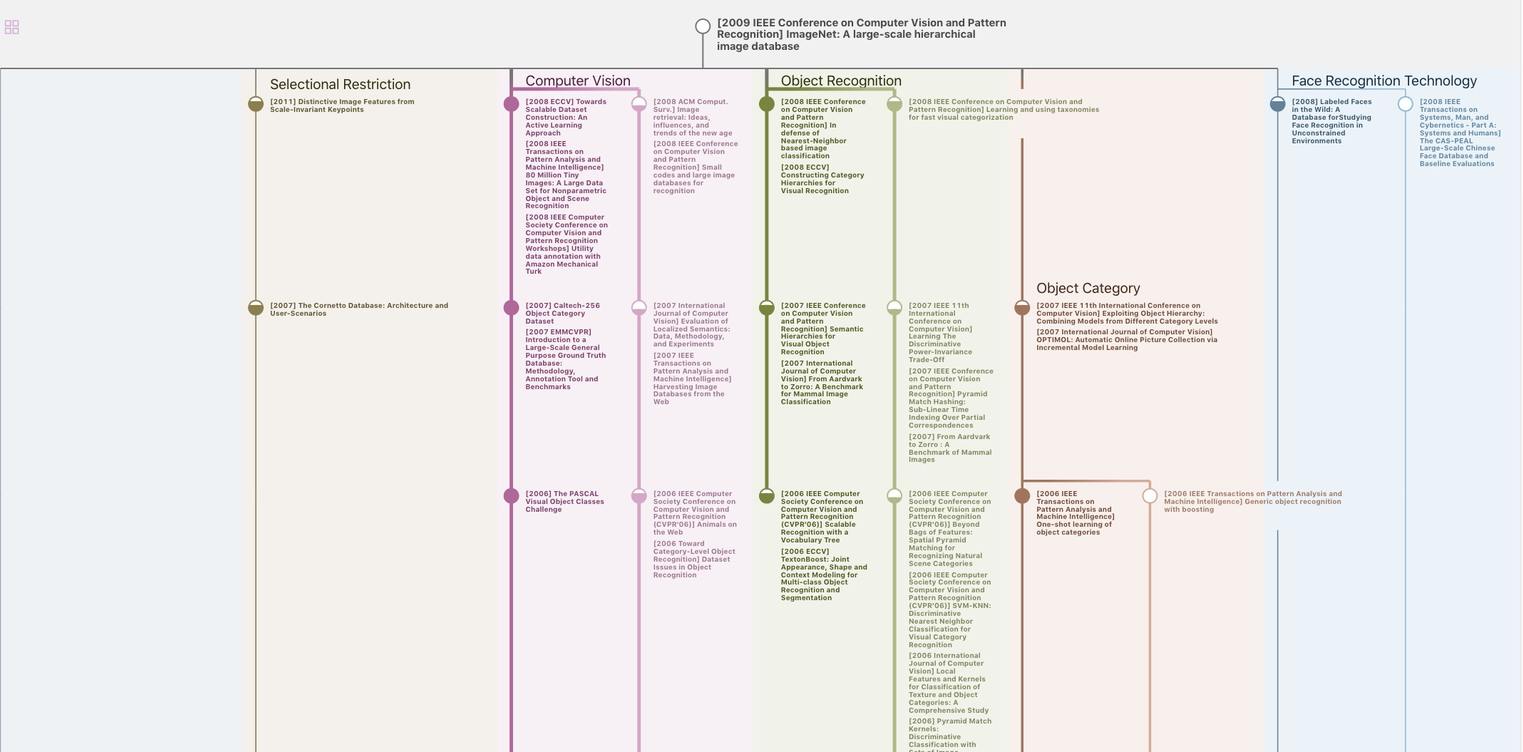
生成溯源树,研究论文发展脉络
Chat Paper
正在生成论文摘要