Congestion Reduction via Personalized Incentives
user-5edf3a5a4c775e09d87cc848(2021)
摘要
With rapid population growth and urban development, traffic congestion has become an inescapable issue, especially in large cities. Many congestion reduction strategies have been proposed in the past, ranging from roadway extension to transportation demand management. In particular, congestion pricing schemes have been used as negative reinforcements for traffic control. In this project, we study an alternative approach of offering positive incentives to drivers to take different routes. More specifically, we propose an algorithm to reduce traffic congestion and improve routing efficiency via offering personalized incentives to drivers. We exploit the wide-accessibility of smart devices to communicate with drivers and develop an incentive offering mechanism using individuals' preferences and aggregate traffic information. The incentives are offered after solving a large-scale optimization problem in order to minimize the total travel time (or minimize any cost function of the network such as total Carbon emission). Since this massive size optimization problem needs to be solved continually in the network, we developed a distributed computational approach. The proposed distributed algorithm is guaranteed to converge under a mild set of assumptions that are verified with real data. We evaluated the performance of our algorithm using traffic data from the Los Angeles area. Our experiments show congestion reduction of up to 11% in arterial roads and highways.
更多查看译文
关键词
Congestion reduction,Personalized incentives,Travel demand management,Behavior change
AI 理解论文
溯源树
样例
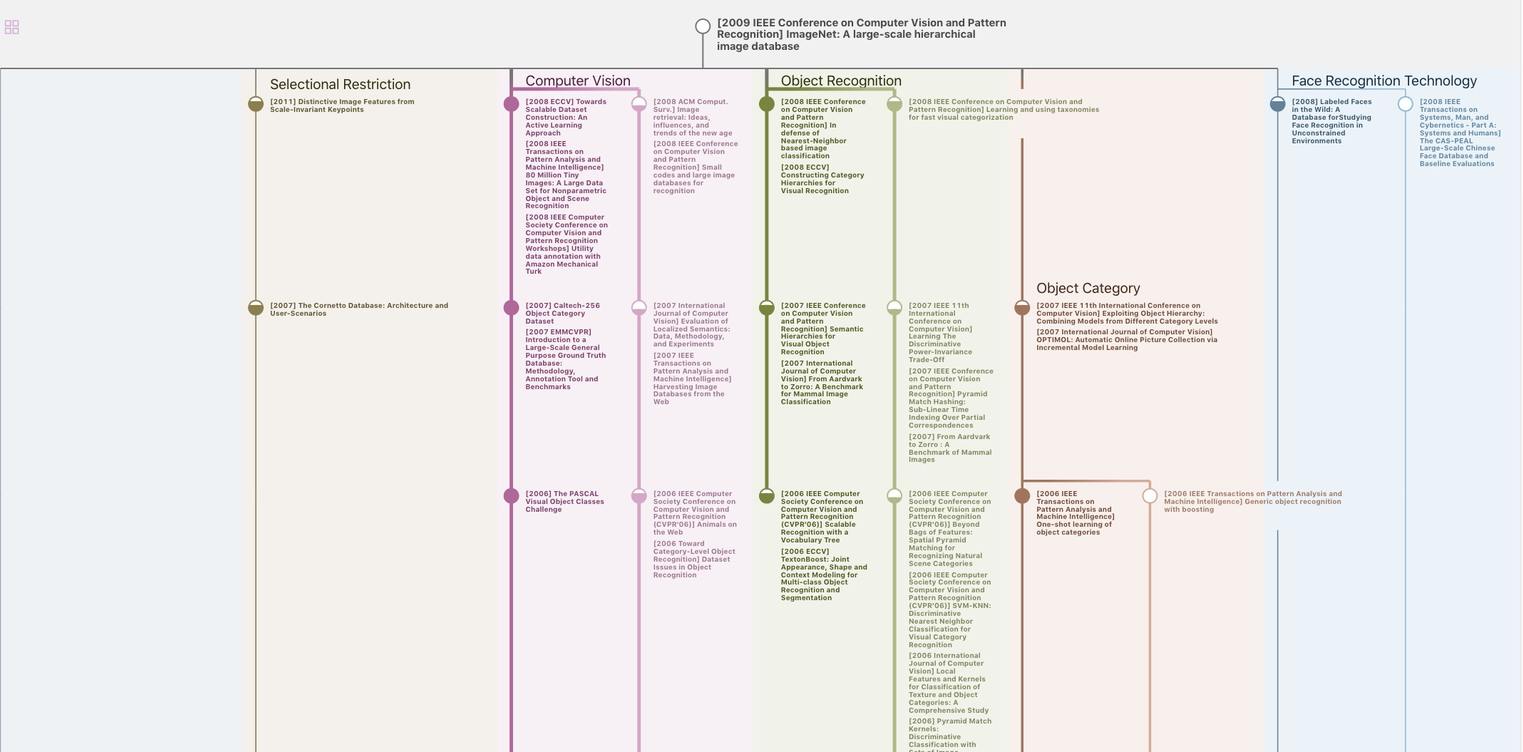
生成溯源树,研究论文发展脉络
Chat Paper
正在生成论文摘要