Zero-shot Transfer Learning for Gray-box Hyper-parameter Optimization
user-5ed732bc4c775e09d87b4c18(2021)
摘要
Zero-shot hyper-parameter optimization refers to the process of selecting hyper- parameter configurations that are expected to perform well for a given dataset upfront, without access to any observations of the losses of the target response. Existing zero-shot approaches are posed as initialization strategies for Bayesian Optimization and they often rely on engineered meta-features to measure dataset similarity, operating under the assumption that the responses of similar datasets behaves similarly with respect to the same hyper-parameters. Solutions for zero- shot HPO are embarrassingly parallelizable and thus can reduce vastly the required wallclock time of learning a single model. We propose a very simple HPO model called Gray-box Zero(0)-Shot Initialization (GROSI) as a conditional parametric surrogate that learns a universal response model by exploiting the relationship between the hyper-parameters and the dataset meta-features directly. In contrast to existing HPO solutions, we achieve transfer of knowledge without engineered meta- features, but rather through a shared model that is trained simultaneously across all datasets. We design and optimize a novel loss function that allows us to regress from the dataset/hyper-parameter pair unto the response. Experiments on 120 datasets demonstrate the strong performance of GROSI, compared to conventional initialization strategies. We also show that by fine-tuning GROSI to the target dataset, we can outperform state-of-the-art sequential HPO algorithms.
更多查看译文
关键词
Initialization,Bayesian optimization,Parametric statistics,Hyperparameter,Gray box testing,Algorithm,Transfer of learning,Parallelizable manifold,Computer science,Response model
AI 理解论文
溯源树
样例
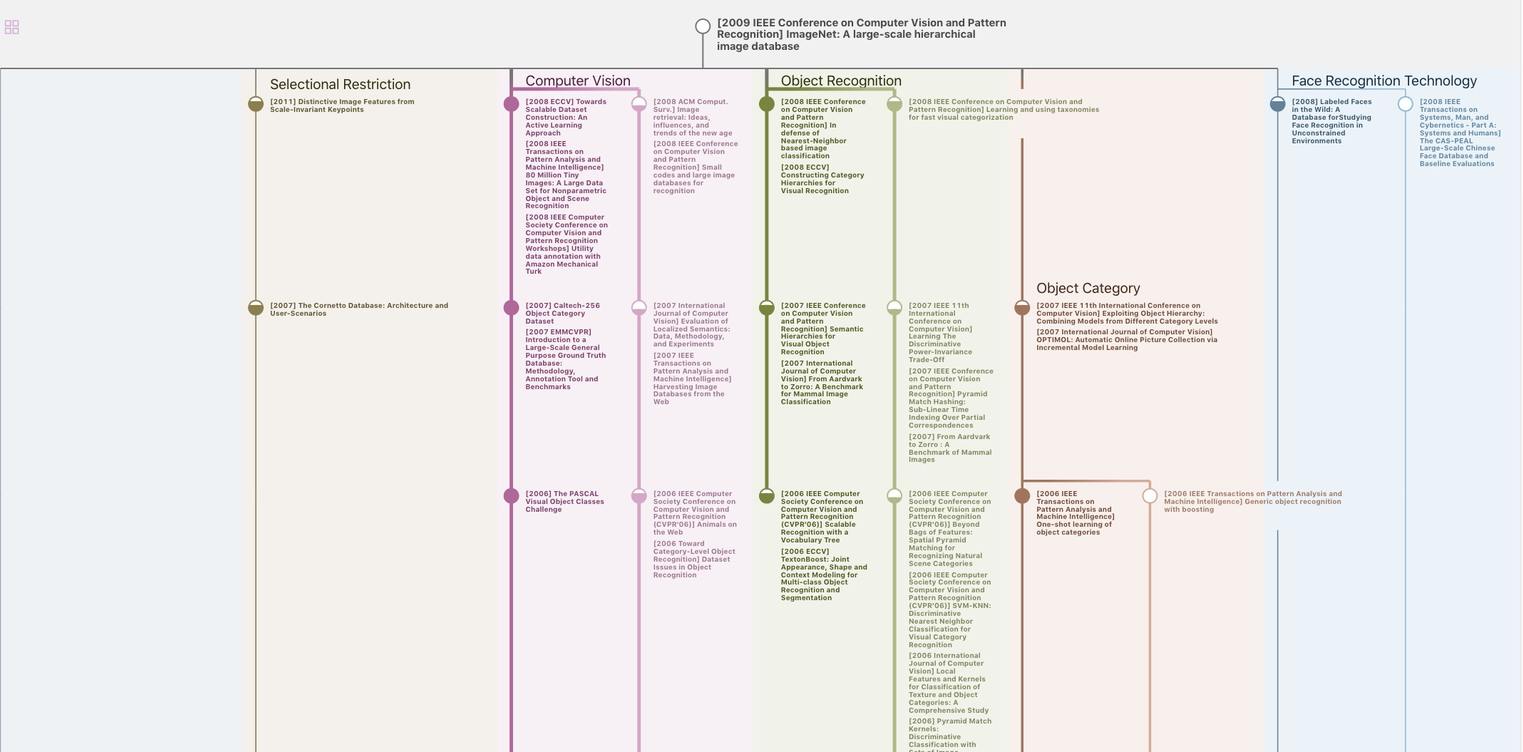
生成溯源树,研究论文发展脉络
Chat Paper
正在生成论文摘要