9.9 A Background-Noise and Process-Variation-Tolerant 109nW Acoustic Feature Extractor Based on Spike-Domain Divisive-Energy Normalization for an Always-On Keyword Spotting Device
2021 IEEE International Solid- State Circuits Conference (ISSCC)(2021)
摘要
In mobile and edge devices, always-on keyword spotting (KWS) is an essential function to detect wake-up words. Recent works achieved extremely low power dissipation down to ~500nW. However, most of them adopt noise-dependent training, i.e. training for a specific signal-to-noise ratio (SNR) and noise type, and therefore their accuracies degrade for different SNR levels and noise types that are not targeted in the training (Fig. 9.9.1, top left). To improve robustness, so-called noise-independent training can be considered, which is to use the training data that includes all the possible SNR levels and noise types. But, this approach is challenging for an ultra-low-power device since it demands a large neural network to learn all the possible features. A neural network of a fixed size has its own memory capacity limit and reaches a plateau in accuracy if it has to learn more than its limit (Fig. 9.9.1, top right). On the other hand, it is known that biological acoustic systems employ a simpler process, called divisive energy normalization (DN), to maintain accuracy even in varying noise conditions. In this work, therefore, by adopting such a DN, we prototype a normalized acoustic feature extractor chip (NAFE) in 65nm. The NAFE can take an acoustic signal from a microphone and produce spike-rate coded features. We pair NAFE with a spiking neural network (SNN) classifier chip, creating the end-to-end KWS system. The proposed system achieves 89-to-94% accuracy across -5 to 20dB SNRs and four different noise types on HeySnips, while the baseline without DN achieves a much lower accuracy of 71-87%. NAFE consumes up to 109nW and the KWS system 570nW.
更多查看译文
关键词
background-noise,spike-domain divisive-energy normalization,keyword spotting device,mobile edge devices,wake-up words,extremely low power dissipation,noise-dependent training,signal-to-noise ratio,training data,SNR levels,ultra-low-power device,memory capacity limit,biological acoustic systems,DN,noise conditions,NAFE,acoustic signal,spike-rate coded features,spiking neural network classifier chip,end-to-end KWS system,noise types,KWS system,divisive energy normalization,noise-independent training,acoustic feature extractor chip,always-on keyword spotting device,size 65.0 nm,power 109.0 nW,power 570.0 nW,noise figure -5.0 dB to 20.0 dB
AI 理解论文
溯源树
样例
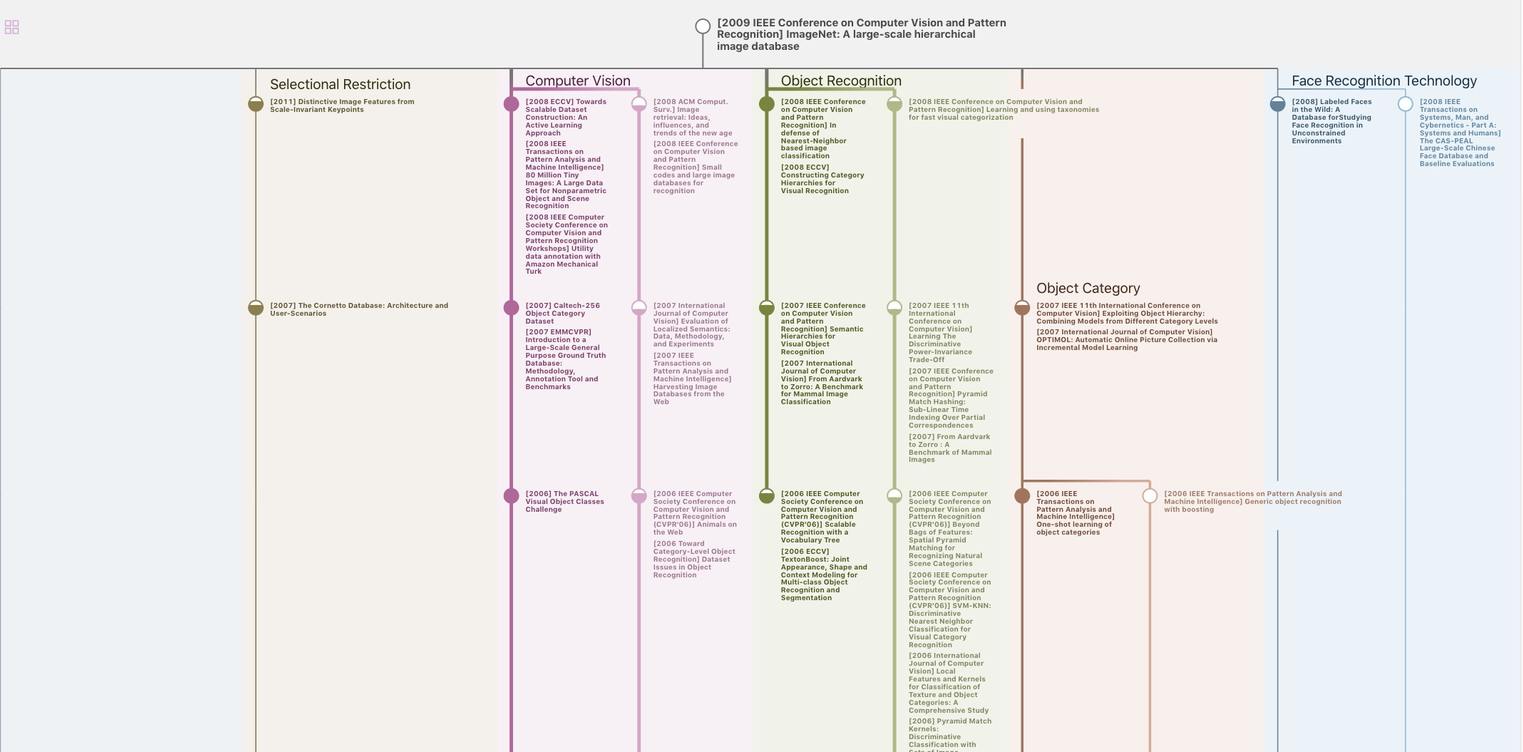
生成溯源树,研究论文发展脉络
Chat Paper
正在生成论文摘要