Interpretable data-driven methods for subgrid-scale closure in LES for transcritical LOX/GCH4 combustion
Combustion and Flame(2022)
摘要
Although data-driven methods have shown high accuracy as closure models for simulating turbulent flames, these models are often criticized for lack of physical interpretability, wherein they provide answers but no insight into their underlying rationale. In this work, we show that two interpretable machine learning algorithms, namely the random forest regressor and the sparse symbolic regression, can offer opportunities for discovering analytic expressions for subgrid-scale (SGS) terms of a turbulent transcritical flame. These transcritical conditions are found in various combustion systems that operate under high pressures that surpass the thermodynamic critical limit of fuel-oxidizer mixtures, and require the consideration of complex fluid behaviors that can cast doubts on the validity of existing subgrid-scale (SGS) models in large-eddy simulations. To this end, direct numerical simulations (DNS) of transcritical liquid-oxygen/gaseous-methane (LOX/GCH4) inert and reacting flows are performed. Using this data, a priori analysis is performed on the Favre-filtered DNS data to compare the accuracy of random forest SGS-models with conventional physics-based SGS-models. SGS stresses calculated with the gradient model are shown to have good agreement with the exact terms extracted from filtered DNS. Results demonstrate that random forests can perform as effectively as algebraic models when modeling subgrid stresses, when trained on a sufficiently representative database and with a suitable choice of the feature set. The employment of the random forest feature importance score is shown to enable the discovery of a analytic model for subgrid-scale stresses through sparse symbolic regression. The generalizability of random forest and sparse symbolic regression is demonstrated by modeling the subgrid-scale temperature, a term that arises from filtering the non-linear real-fluid equation-of-state, with good accuracy.
更多查看译文
关键词
Turbulence modeling,Direct numerical simulation,Transcritical combustion,Random forests,Machine learning,Explainable artificial intelligence
AI 理解论文
溯源树
样例
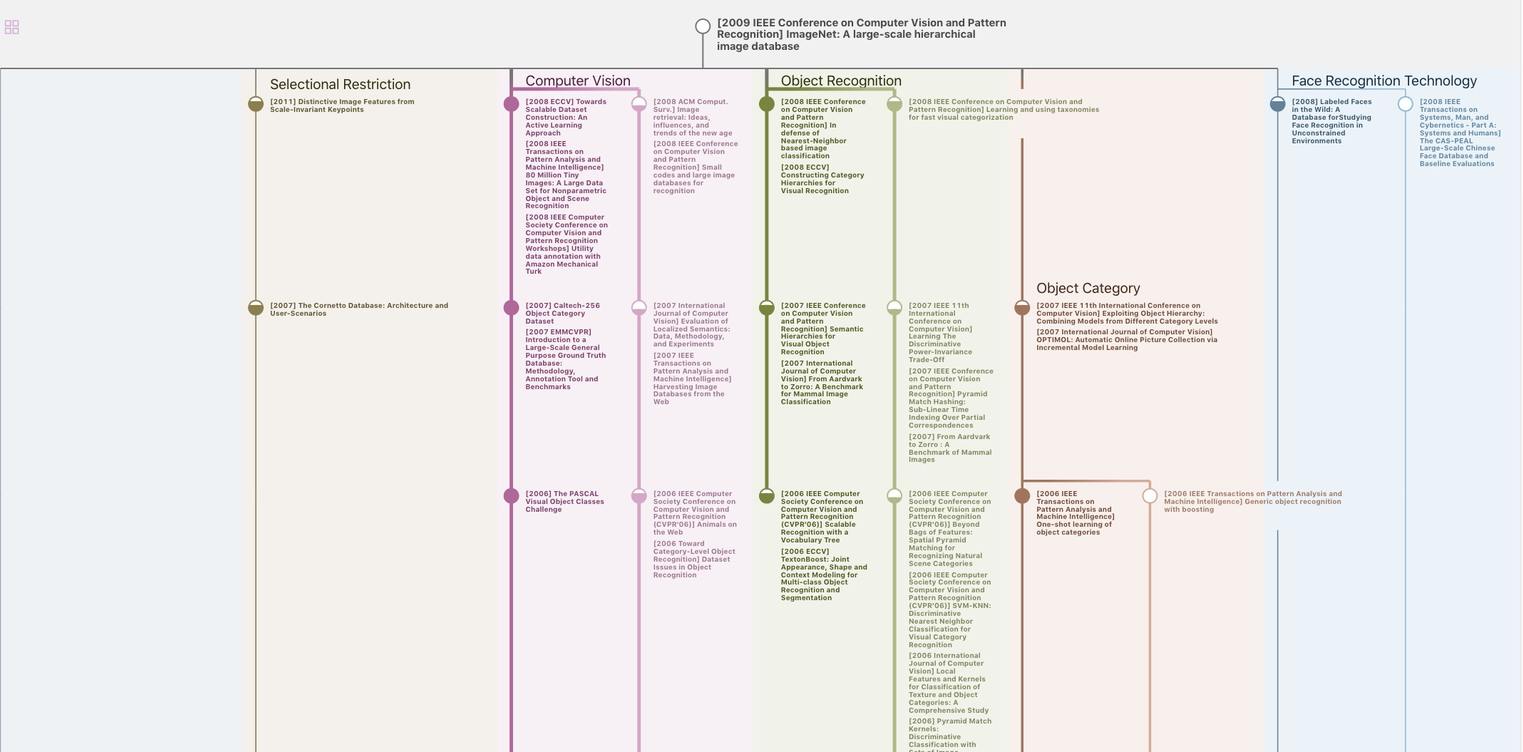
生成溯源树,研究论文发展脉络
Chat Paper
正在生成论文摘要