Full Gradient DQN Reinforcement Learning: A Provably Convergent Scheme
arxiv(2021)
摘要
We analyze the DQN reinforcement learning algorithm as a stochastic approximation scheme using the o.d.e. (for `ordinary differential equation') approach and point out certain theoretical issues. We then propose a modified scheme called Full Gradient DQN (FG-DQN, for short) that has a sound theoretical basis and compare it with the original scheme on sample problems. We observe a better performance for FG-DQN.
更多查看译文
关键词
Markov decision process (MDP), Approximate dynamic programming, Deep Reinforcement Learning (DRL), Stochastic approximation, Deep Q-network (DQN), Full Gradient DQN, Bellman error minimization, Primary 93E35, Secondary 68T05, 90C40, 93E35
AI 理解论文
溯源树
样例
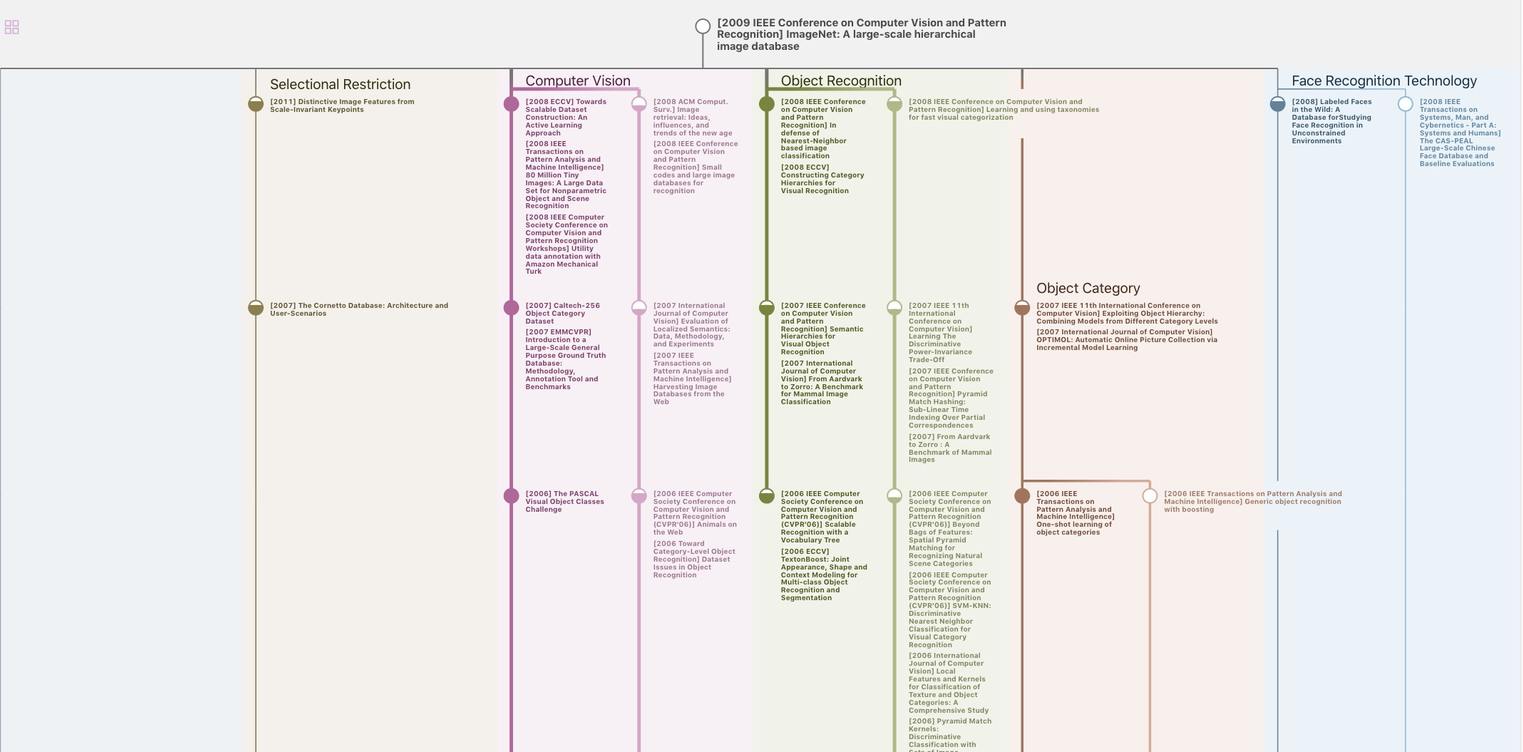
生成溯源树,研究论文发展脉络
Chat Paper
正在生成论文摘要