Experimental Leg Inverse Dynamics Learning of Multi-legged Walking Robot
MODELLING AND SIMULATION FOR AUTONOMOUS SYSTEMS (MESAS 2020)(2021)
摘要
Rough terrain locomotion is a domain where multi-legged robots benefit from their relatively complex morphology compared to the wheeled or tracked robots. Efficient rough terrain locomotion requires the legged robot sense contacts with the terrain to adapt its behavior and cope with the terrain irregularities. Usage of inverse dynamics to estimate the leg state and detect the leg contacts with the terrain suffers from computational complexity. Furthermore, it requires a precise analytical model identification that does not cope with adverse changes of the leg parameters such as friction changes due to the joint wear, the increased weight of the leg due to the mud deposits, and possible leg morphology change due to damage. In this paper, we report the experimental study on the locomotion performance with machine learning-based inverse dynamics model learning. Experimental examining three different learning models show that a simplified model is sufficient for leg collision detection learning. Moreover, the learned model is faster for calculation and generalizes better than more complex models when the leg parameters change.
更多查看译文
关键词
dynamics,robot,learning,multi-legged
AI 理解论文
溯源树
样例
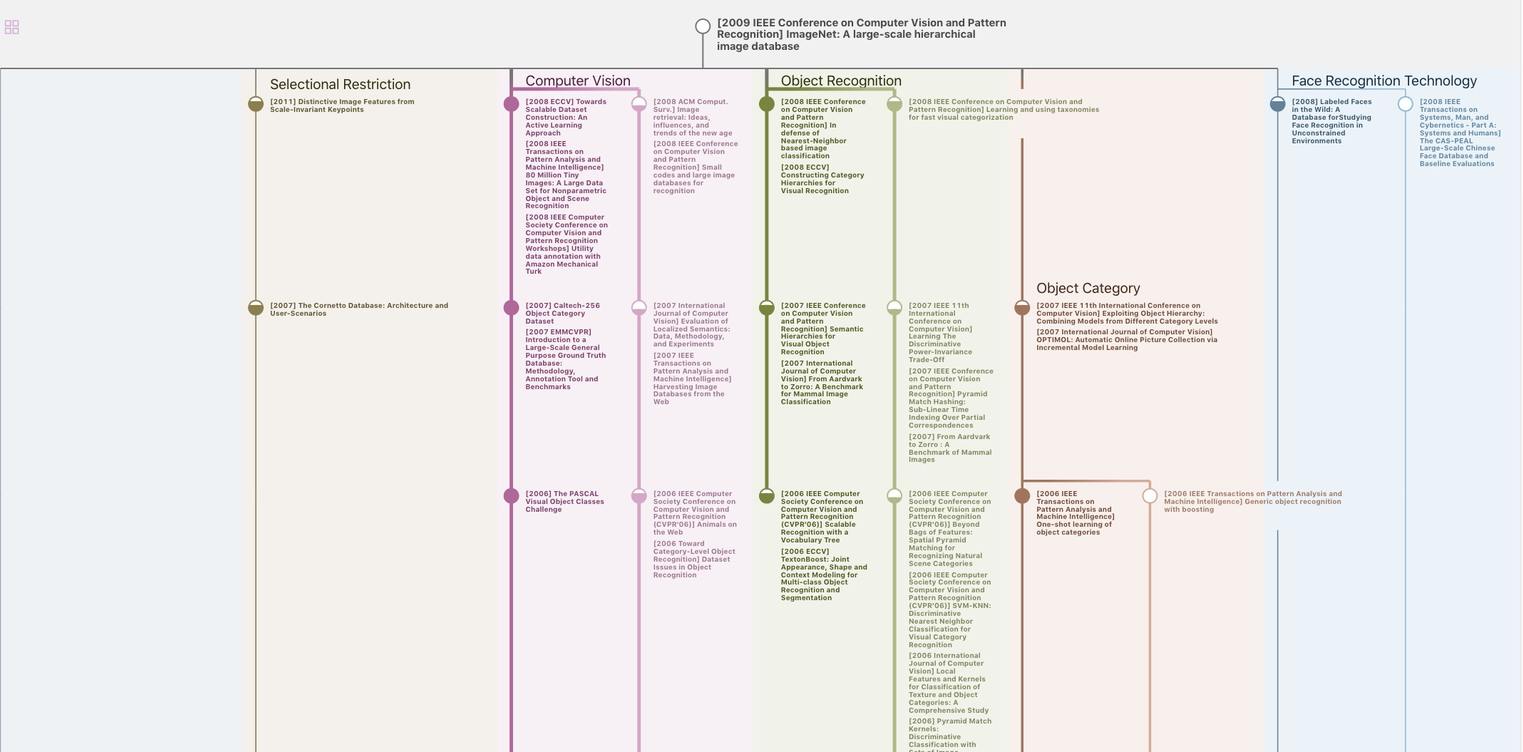
生成溯源树,研究论文发展脉络
Chat Paper
正在生成论文摘要