Continual Density Ratio Estimation in an Online Setting
arxiv(2021)
摘要
In online applications with streaming data, awareness of how far the training or test set has shifted away from the original dataset can be crucial to the performance of the model. However, we may not have access to historical samples in the data stream. To cope with such situations, we propose a novel method, Continual Density Ratio Estimation (CDRE), for estimating density ratios between the initial and current distributions ($p/q_t$) of a data stream in an iterative fashion without the need of storing past samples, where $q_t$ is shifting away from $p$ over time $t$. We demonstrate that CDRE can be more accurate than standard DRE in terms of estimating divergences between distributions, despite not requiring samples from the original distribution. CDRE can be applied in scenarios of online learning, such as importance weighted covariate shift, tracing dataset changes for better decision making. In addition, (CDRE) enables the evaluation of generative models under the setting of continual learning. To the best of our knowledge, there is no existing method that can evaluate generative models in continual learning without storing samples from the original distribution.
更多查看译文
关键词
estimation,density,ratio,online setting
AI 理解论文
溯源树
样例
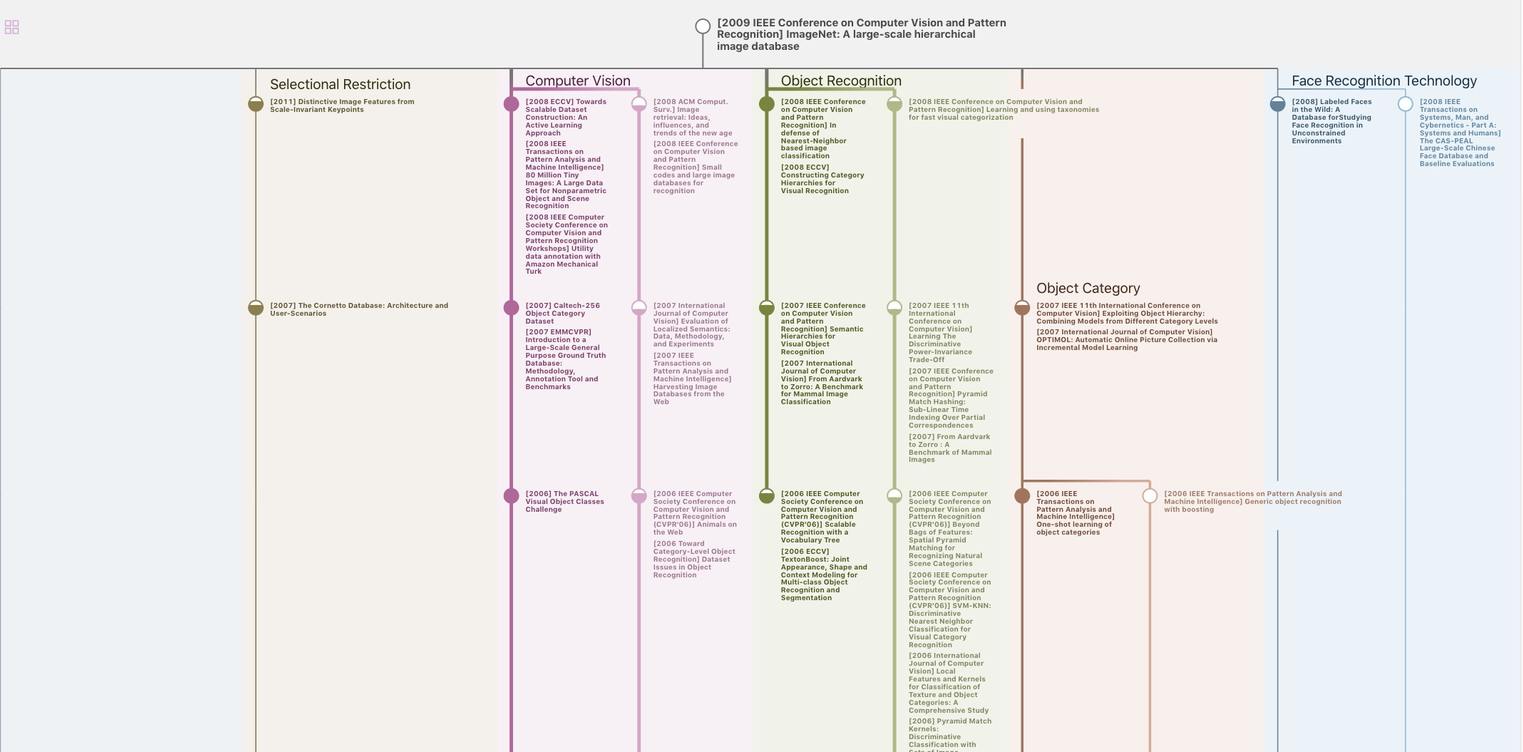
生成溯源树,研究论文发展脉络
Chat Paper
正在生成论文摘要