Deep Generative Modelling: A Comparative Review of VAEs, GANs, Normalizing Flows, Energy-Based and Autoregressive Models
IEEE Transactions on Pattern Analysis and Machine Intelligence(2022)
摘要
Deep generative models are a class of techniques that train deep neural networks to model the distribution of training samples. Research has fragmented into various interconnected approaches, each of which make trade-offs including run-time, diversity, and architectural restrictions. In particular, this compendium covers energy-based models, variational autoencoders, generative adversarial networks, autoregressive models, normalizing flows, in addition to numerous hybrid approaches. These techniques are compared and contrasted, explaining the premises behind each and how they are interrelated, while reviewing current state-of-the-art advances and implementations.
更多查看译文
关键词
Deep learning,generative models,energy-based models,variational autoencoders,generative adversarial networks,autoregressive models,normalizing flows
AI 理解论文
溯源树
样例
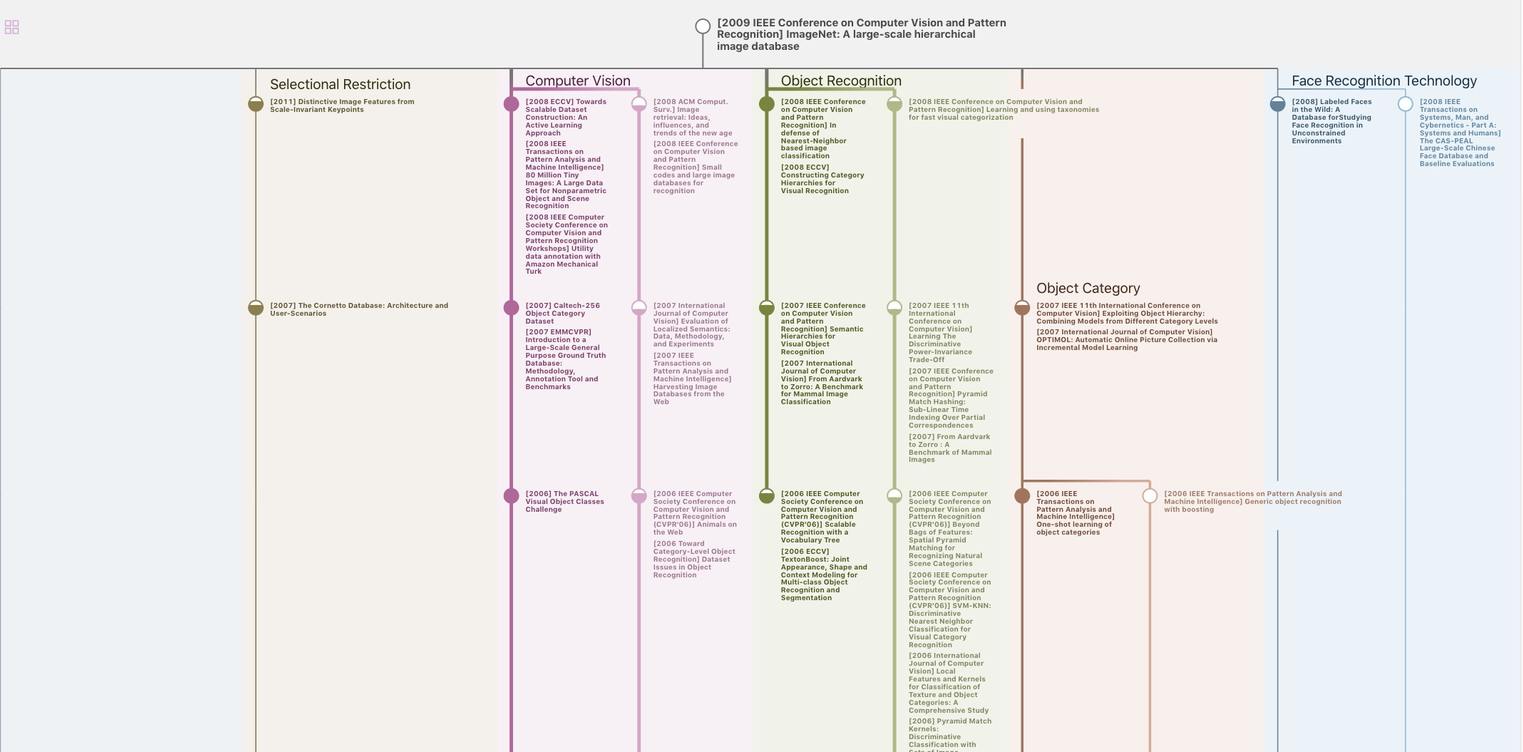
生成溯源树,研究论文发展脉络
Chat Paper
正在生成论文摘要