Calibrating Network Traffic With One-Dimensional Convolutional Neural Network With Autoencoder And Independent Recurrent Neural Network For Mobile Malware Detection
SECURITY AND COMMUNICATION NETWORKS(2021)
摘要
In response to the surging challenge in the number and types of mobile malware targeting smart devices and their sophistication in malicious behavior camouflage, we propose to compose a traffic behavior modeling method based on one-dimensional convolutional neural network with autoencoder and independent recurrent neural network (1DCAE-IndRNN) for mobile malware detection. The design solves the problem that most existing approaches for mobile malware traffic detection struggle with capturing the network traffic dynamics and the sequential characteristics of anomalies in the traffic. We reconstruct and apply the one-dimensional convolutional neural network to extract local features from multiple network flows. The autoencoder is applied to digest the principal traffic features from the neural network and is integrated into the independent recurrent neural network construction to highlight the sequential relationship between the highly significant features. In addition, the Softmax function with the LReL U activation function is adjusted and embedded to the neurons of the independent recurrent neural network to effectively alleviate the problem of unstable training. We conduct a series of experiments to evaluate the effectiveness of the proposed method and its performance for the 1DCAE-IndRNN-integrated detection procedure. The detection results of the public Android malware dataset CICAndMal2017 show that the proposed method achieves up to 98% detection accuracy and recall rates with clear advantages over other benchmark methods.
更多查看译文
AI 理解论文
溯源树
样例
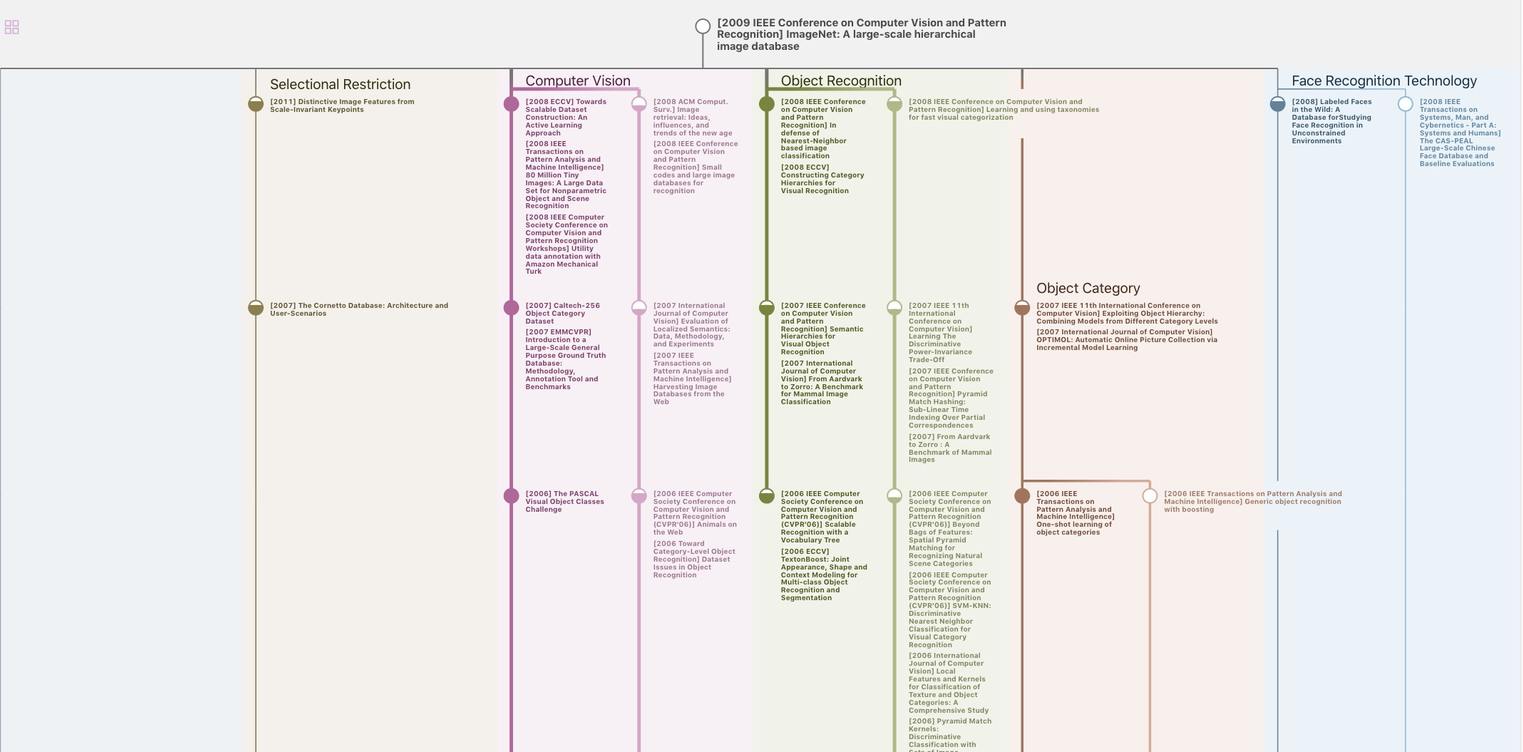
生成溯源树,研究论文发展脉络
Chat Paper
正在生成论文摘要