Hands-On Training About Overfitting
PLOS COMPUTATIONAL BIOLOGY(2021)
摘要
Author summaryEvery teacher strives for an a-ha moment, a sudden revelation by the student who gained a fundamental insight she will always remember. In the past years, authors of this paper have been tailoring their courses in machine learning to include material that could lead students to such discoveries. We aim to expose machine learning to practitioners-not only computer scientists but also molecular biologists and students of biomedicine, that is, the end-users of bioinformatics' computational approaches. In this article, we lay out a course that aims to teach about overfitting, one of the key concepts in machine learning that needs to be understood, mastered, and avoided in data science applications. We propose a hands-on approach that uses an open-source workflow-based data science toolbox that combines data visualization and machine learning. In the proposed training about overfitting, we first deceive the students, then expose the problem, and finally challenge them to find the solution. In the paper, we present three lessons in overfitting and associated data analysis workflows and motivate the use of introduced computation methods by relating them to concepts conveyed by instructors.Overfitting is one of the critical problems in developing models by machine learning. With machine learning becoming an essential technology in computational biology, we must include training about overfitting in all courses that introduce this technology to students and practitioners. We here propose a hands-on training for overfitting that is suitable for introductory level courses and can be carried out on its own or embedded within any data science course. We use workflow-based design of machine learning pipelines, experimentation-based teaching, and hands-on approach that focuses on concepts rather than underlying mathematics. We here detail the data analysis workflows we use in training and motivate them from the viewpoint of teaching goals. Our proposed approach relies on Orange, an open-source data science toolbox that combines data visualization and machine learning, and that is tailored for education in machine learning and explorative data analysis.
更多查看译文
AI 理解论文
溯源树
样例
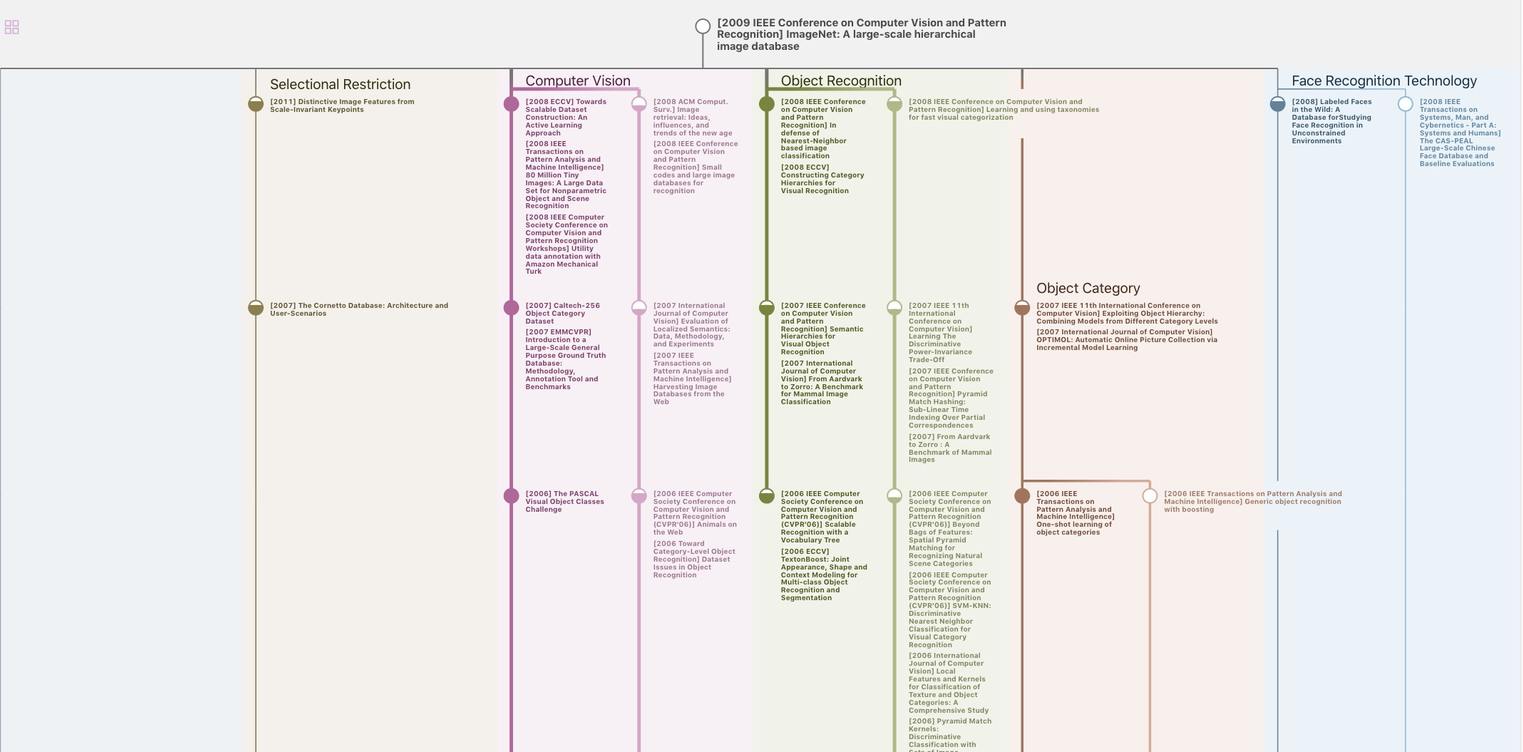
生成溯源树,研究论文发展脉络
Chat Paper
正在生成论文摘要