Universal Representation for Code
ADVANCES IN KNOWLEDGE DISCOVERY AND DATA MINING, PAKDD 2021, PT III(2021)
摘要
Learning from source code usually requires a large amount of labeled data. Despite the possible scarcity of labeled data, the trained model is highly task-specific and lacks transferability to different tasks. In this work, we present effective pre-training strategies on top of a novel graph-based code representation, to produce universal representations for code. Specifically, our graph-based representation captures important semantics between code elements (e.g., control flow and data flow). We pre-train graph neural networks on the representation to extract universal code properties. The pre-trained model then enables the possibility of fine-tuning to support various downstream applications. We evaluate our model on two real-world datasets - spanning over 30M Java methods and 770K Python methods. Through visualization, we reveal discriminative properties in our universal code representation. By comparing multiple benchmarks, we demonstrate that the proposed framework achieves state-of-the-art results on method name prediction and code graph link prediction.
更多查看译文
关键词
Code representation, Graph neural network, Pre-training
AI 理解论文
溯源树
样例
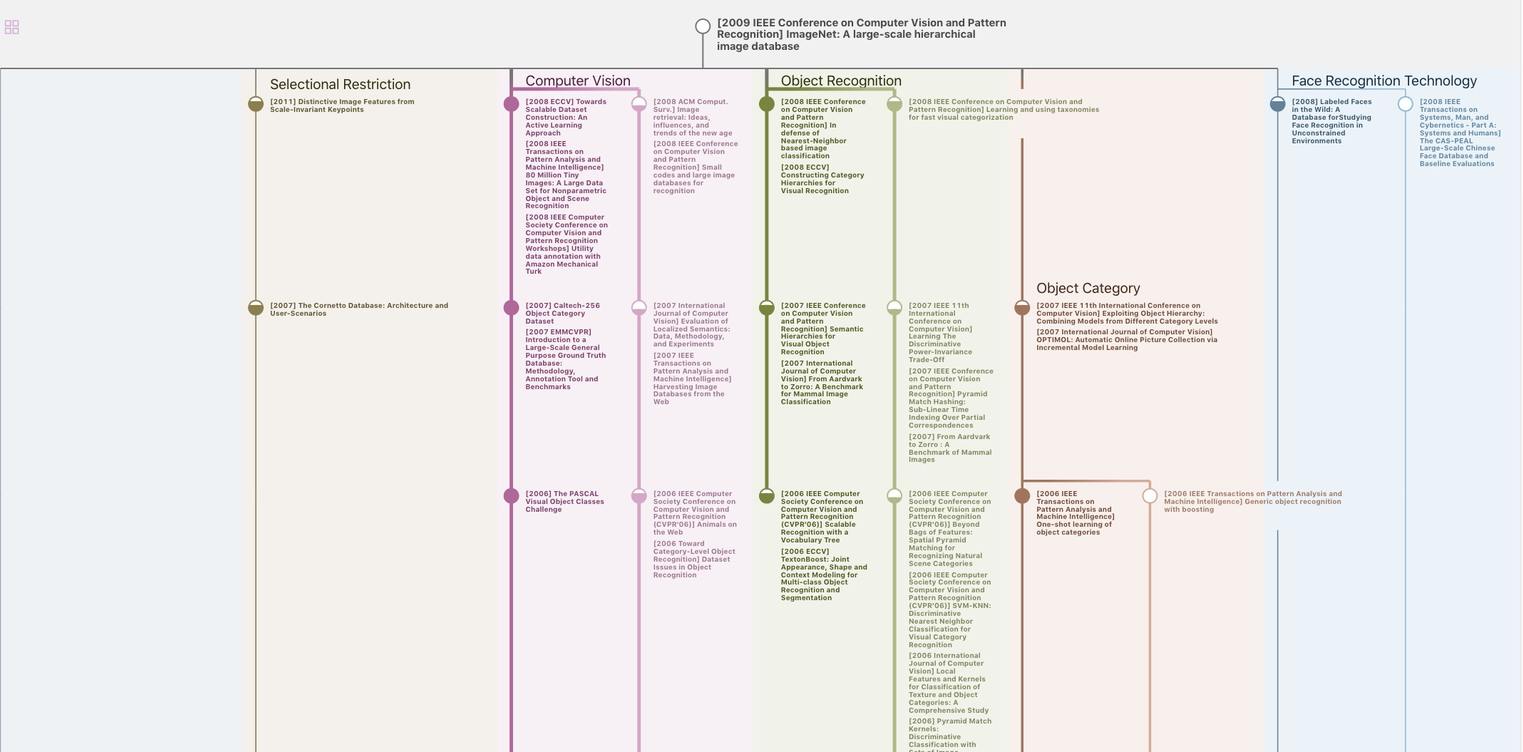
生成溯源树,研究论文发展脉络
Chat Paper
正在生成论文摘要