Heart rate variability-derived features based on deep neural network for distinguishing different anaesthesia states
BMC ANESTHESIOLOGY(2021)
摘要
Background Estimating the depth of anaesthesia (DoA) is critical in modern anaesthetic practice. Multiple DoA monitors based on electroencephalograms (EEGs) have been widely used for DoA monitoring; however, these monitors may be inaccurate under certain conditions. In this work, we hypothesize that heart rate variability (HRV)-derived features based on a deep neural network can distinguish different anaesthesia states, providing a secondary tool for DoA assessment. Methods A novel method of distinguishing different anaesthesia states was developed based on four HRV-derived features in the time and frequency domain combined with a deep neural network. Four features were extracted from an electrocardiogram, including the HRV high-frequency power, low-frequency power, high-to-low-frequency power ratio, and sample entropy. Next, these features were used as inputs for the deep neural network, which utilized the expert assessment of consciousness level as the reference output. Finally, the deep neural network was compared with the logistic regression, support vector machine, and decision tree models. The datasets of 23 anaesthesia patients were used to assess the proposed method. Results The accuracies of the four models, in distinguishing the anaesthesia states, were 86.2% (logistic regression), 87.5% (support vector machine), 87.2% (decision tree), and 90.1% (deep neural network). The accuracy of deep neural network was higher than those of the logistic regression ( p < 0.05), support vector machine ( p < 0.05), and decision tree ( p < 0.05) approaches. Our method outperformed the logistic regression, support vector machine, and decision tree methods. Conclusions The incorporation of four HRV-derived features in the time and frequency domain and a deep neural network could accurately distinguish between different anaesthesia states; however, this study is a pilot feasibility study. The proposed method—with other evaluation methods, such as EEG—is expected to assist anaesthesiologists in the accurate evaluation of the DoA.
更多查看译文
关键词
Depth of anaesthesia,Heart rate variability,Deep neural network,Discrete wavelet transform
AI 理解论文
溯源树
样例
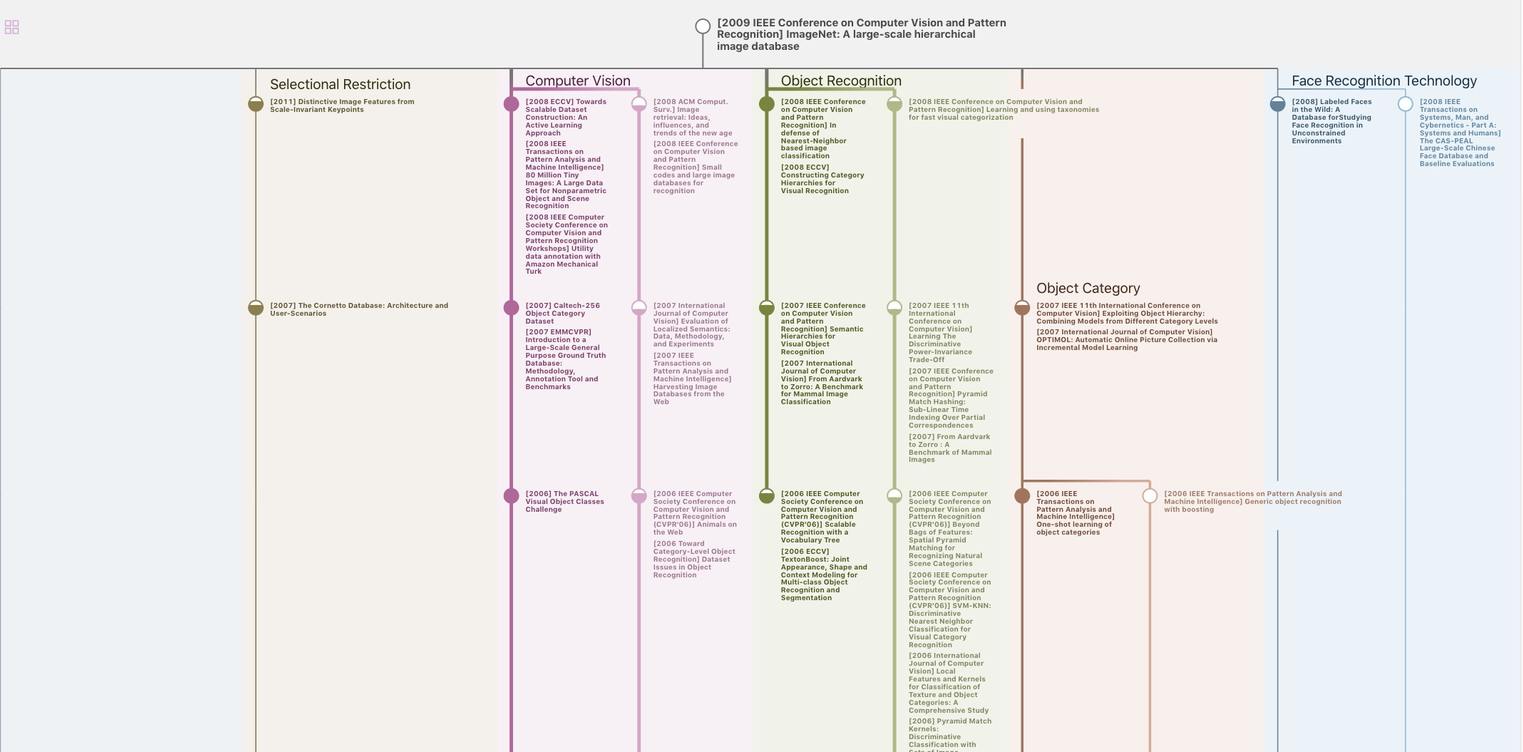
生成溯源树,研究论文发展脉络
Chat Paper
正在生成论文摘要