Image-to-image Translation via Hierarchical Style Disentanglement
2021 IEEE/CVF CONFERENCE ON COMPUTER VISION AND PATTERN RECOGNITION, CVPR 2021(2021)
摘要
Recently, image-to-image translation has made significant progress in achieving both multi-label (i.e., translation conditioned on different labels) and multi-style (i.e., generation with diverse styles) tasks. However, due to the unexplored independence and exclusiveness in the labels, existing endeavors are defeated by involving uncontrolled manipulations to the translation results. In this paper, we propose Hierarchical Style Disentanglement (HiSD) to address this issue. Specifically, we organize the labels into a hierarchical tree structure, in which independent tags, exclusive attributes, and disentangled styles are allocated from top to bottom. Correspondingly, a new translation process is designed to adapt the above structure, in which the styles are identified for controllable translations. Both qualitative and quantitative results on the CelebA-HQ dataset verify the ability of the proposed HiSD. The code has been released at https://github.com/imlixinyang/HiSD.
更多查看译文
关键词
image-to-image translation,hierarchical tree structure,disentangled styles,multilabel translation,hierarchical style disentanglement,exclusive attributes,CelebA-HQ dataset
AI 理解论文
溯源树
样例
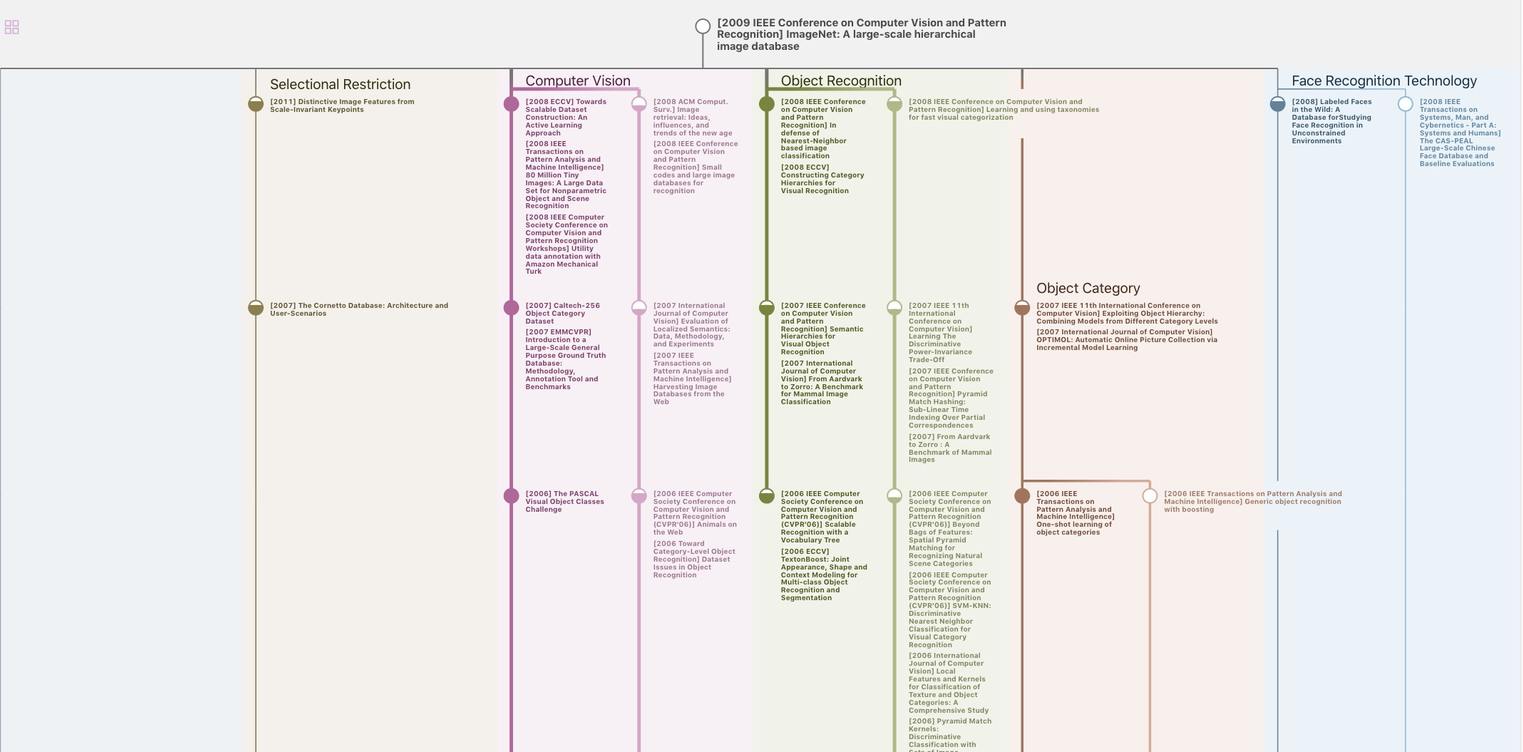
生成溯源树,研究论文发展脉络
Chat Paper
正在生成论文摘要