Exploration and Incentives in Reinforcement Learning
arxiv(2023)
摘要
How do you incentivize self-interested agents to $\textit{explore}$ when they prefer to $\textit{exploit}$? We consider complex exploration problems, where each agent faces the same (but unknown) MDP. In contrast with traditional formulations of reinforcement learning, agents control the choice of policies, whereas an algorithm can only issue recommendations. However, the algorithm controls the flow of information, and can incentivize the agents to explore via information asymmetry. We design an algorithm which explores all reachable states in the MDP. We achieve provable guarantees similar to those for incentivizing exploration in static, stateless exploration problems studied previously. To the best of our knowledge, this is the first work to consider mechanism design in a stateful, reinforcement learning setting.
更多查看译文
关键词
reinforcement learning,exploration,incentives
AI 理解论文
溯源树
样例
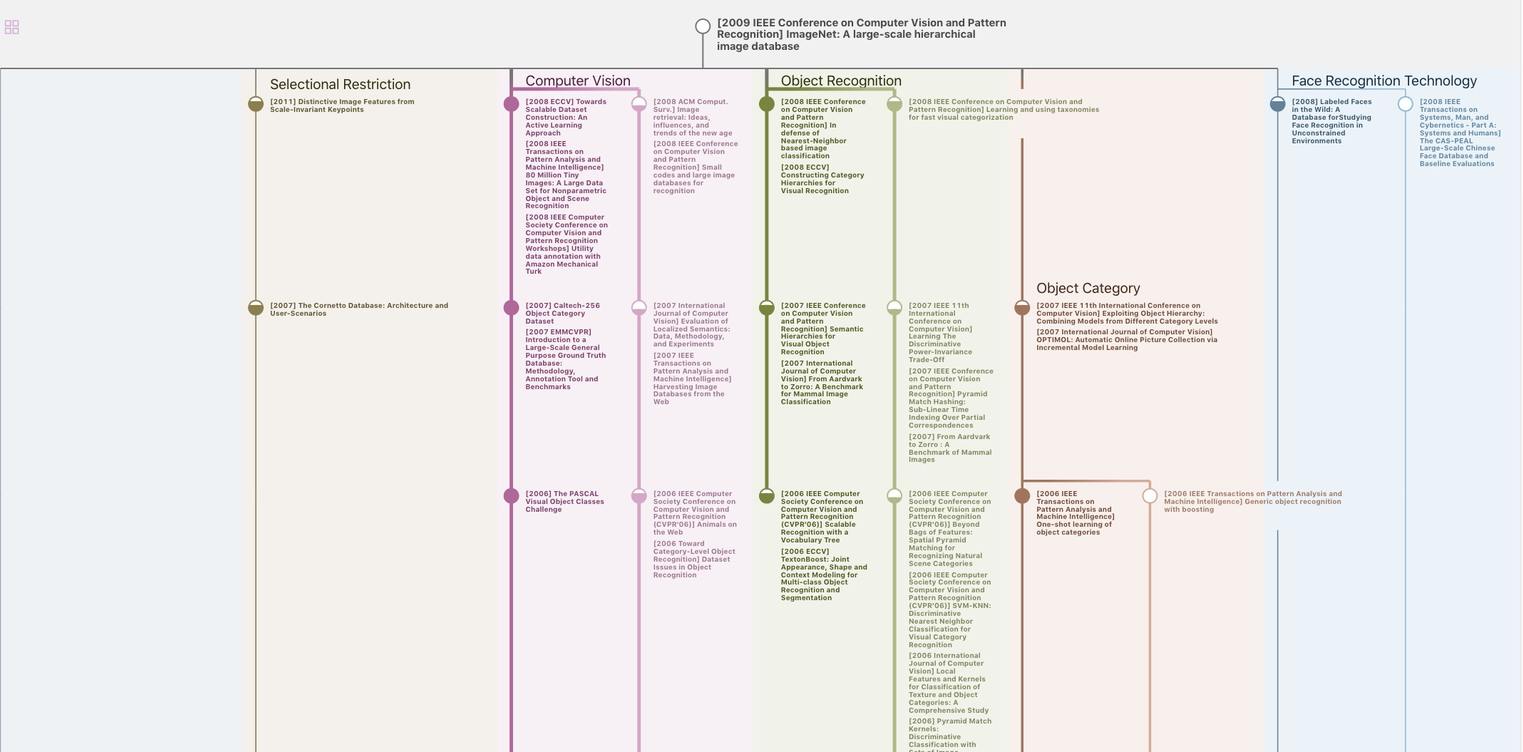
生成溯源树,研究论文发展脉络
Chat Paper
正在生成论文摘要