Language Model Adaptation through Shared Linear Transformations
user-5f8411ab4c775e9685ff56d3(2014)
摘要
Language model (LM) adaptation is an active area in natural language processing and has been successfully applied to speech recognition and to many other applications. To provide fine-grained probability adaptation for each n-grams, we in this work propose three adaptation methods based on shared linear transformations: n-gram-based linear regression, interpolation, and direct estimation. Further, in order to address the problem of data sparseness, n-grams are clustered and those in the same cluster group are made to share the same adaptation parameters. We carry out evaluation experiments on a domain adaptation task with limited adaptation data. The experimental results show that the best LM after our adaption method can reduce the perplexity by half compared with the baseline LM without adaptation, and that it also achieves a perplexity reduction of 15% compared with the earlier state-of-the-art LM adaptation methods. The speech recognition experimental results show that the proposed LM adaptation method reduces the WER by 20.8% compared with the baseline LM without adaptation.
更多查看译文
关键词
Perplexity,Language model,Interpolation,Linear regression,Natural language processing,Linear map,Computer science,A domain,Adaptation method,Artificial intelligence,Cluster group
AI 理解论文
溯源树
样例
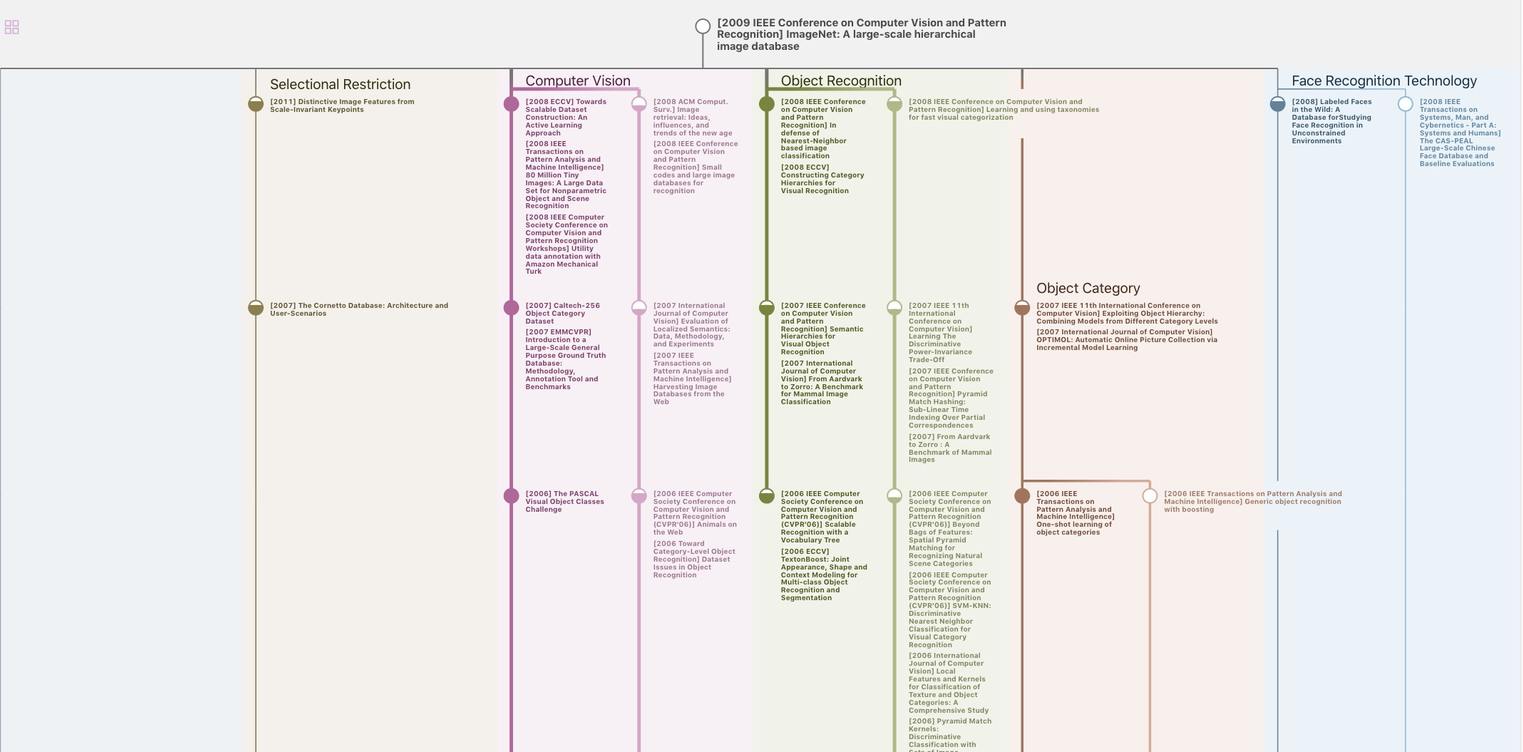
生成溯源树,研究论文发展脉络
Chat Paper
正在生成论文摘要