Optimising A Simple Fully Convolutional Network For Accurate Brain Age Prediction In The Pac 2019 Challenge
FRONTIERS IN PSYCHIATRY(2021)
摘要
Brain age prediction from brain MRI scans not only helps improve brain ageing modelling generally, but also provides benchmarks for predictive analysis methods. Brain-age delta, which is the difference between a subject's predicted age and true age, has become a meaningful biomarker for the health of the brain. Here, we report the details of our brain age prediction models and results in the Predictive Analysis Challenge 2019. The aim of the challenge was to use T1-weighted brain MRIs to predict a subject's age in multicentre datasets. We apply a lightweight deep convolutional neural network architecture, Simple Fully Convolutional Neural Network (SFCN), and combined several techniques including data augmentation, transfer learning, model ensemble, and bias correction for brain age prediction. The model achieved first place in both of the two objectives in the PAC 2019 brain age prediction challenge: Mean absolute error (MAE) = 2.90 years without bias removal (Second Place = 3.09 yrs; Third Place = 3.33 yrs), and MAE = 2.95 years with bias removal, leading by a large margin (Second Place = 3.80 yrs; Third Place = 3.92 yrs).
更多查看译文
关键词
predictive analysis, big data, deep learning, convolution neural network, brain age prediction, brain imaging
AI 理解论文
溯源树
样例
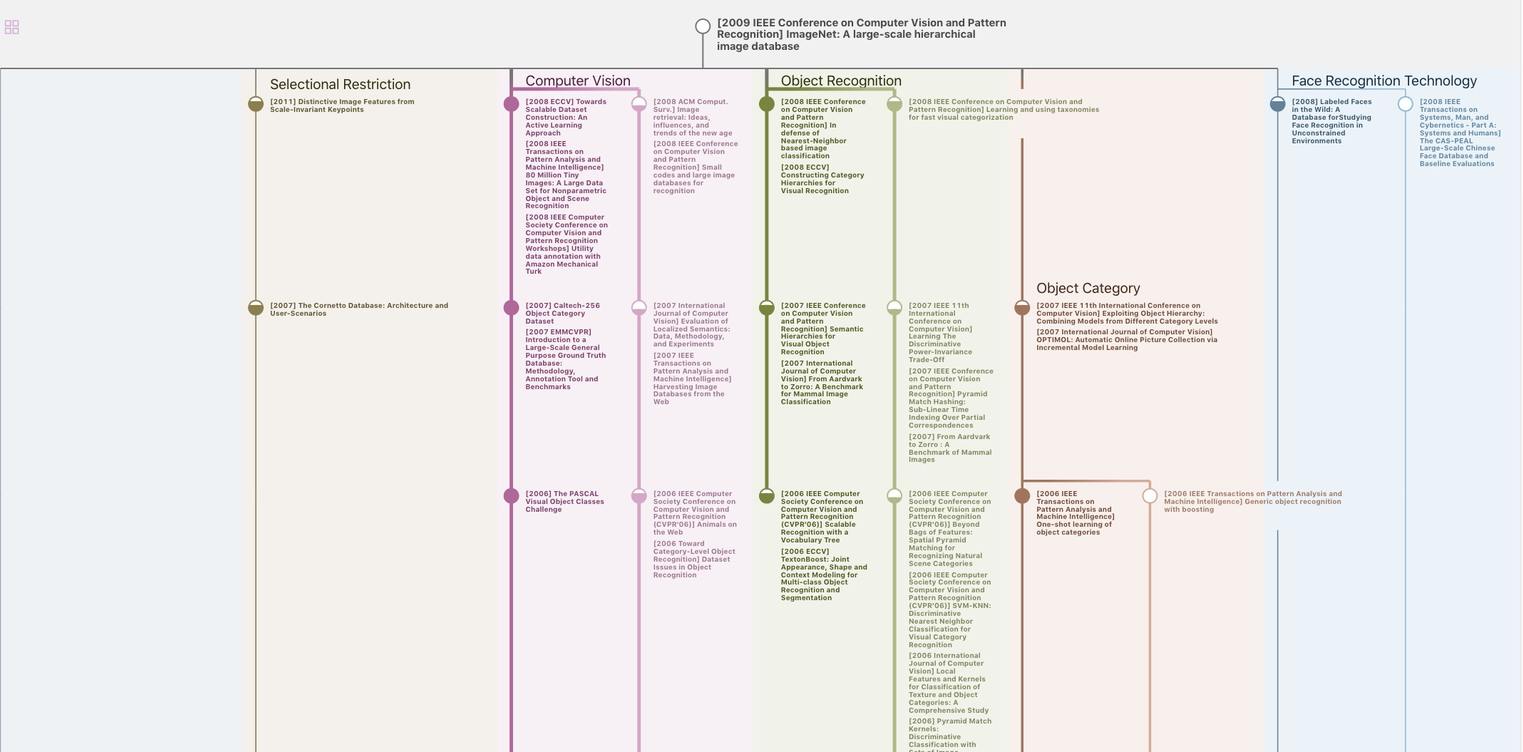
生成溯源树,研究论文发展脉络
Chat Paper
正在生成论文摘要