Proof of concept: Screening for REM sleep behaviour disorder with a minimal set of sensors
Clinical Neurophysiology(2021)
摘要
Objective
Rapid-Eye-Movement (REM) sleep behaviour disorder (RBD) is an early predictor of Parkinson’s disease, dementia with Lewy bodies, and multiple system atrophy. This study investigated the use of a minimal set of sensors to achieve effective screening for RBD in the population, integrating automated sleep staging (three state) followed by RBD detection without the need for cumbersome electroencephalogram (EEG) sensors.
Methods
Polysomnography signals from 50 participants with RBD and 50 age-matched healthy controls were used to evaluate this study. Three stage sleep classification was achieved using a random forest classifier and features derived from a combination of cost-effective and easy to use sensors, namely electrocardiogram (ECG), electrooculogram (EOG), and electromyogram (EMG) channels. Subsequently, RBD detection was achieved using established and new metrics derived from ECG and EMG channels.
Results
The EOG and EMG combination provided the optimal minimalist fully-automated performance, achieving 0.57 ± 0.19 kappa (3 stage) for sleep staging and an RBD detection accuracy of 0.90 ± 0.11, (sensitivity and specificity of 0.88 ± 0.13 and 0.92 ± 0.098, respectively). A single ECG sensor achieved three state sleep staging with 0.28 ± 0.06 kappa and RBD detection accuracy of 0.62 ± 0.10.
Conclusions
This study demonstrates the feasibility of using signals from a single EOG and EMG sensor to detect RBD using fully-automated techniques.
Significance
This study proposes a cost-effective, practical, and simple RBD identification support tool using only two sensors (EMG and EOG); ideal for screening purposes.
更多查看译文
关键词
Automated sleep staging,Electrocardiogram,Electrooculogram,Electromyography,Parkinson’s disease,Polysomnography,REM sleep behaviour disorder,RBD,Sleep diagnostic tool
AI 理解论文
溯源树
样例
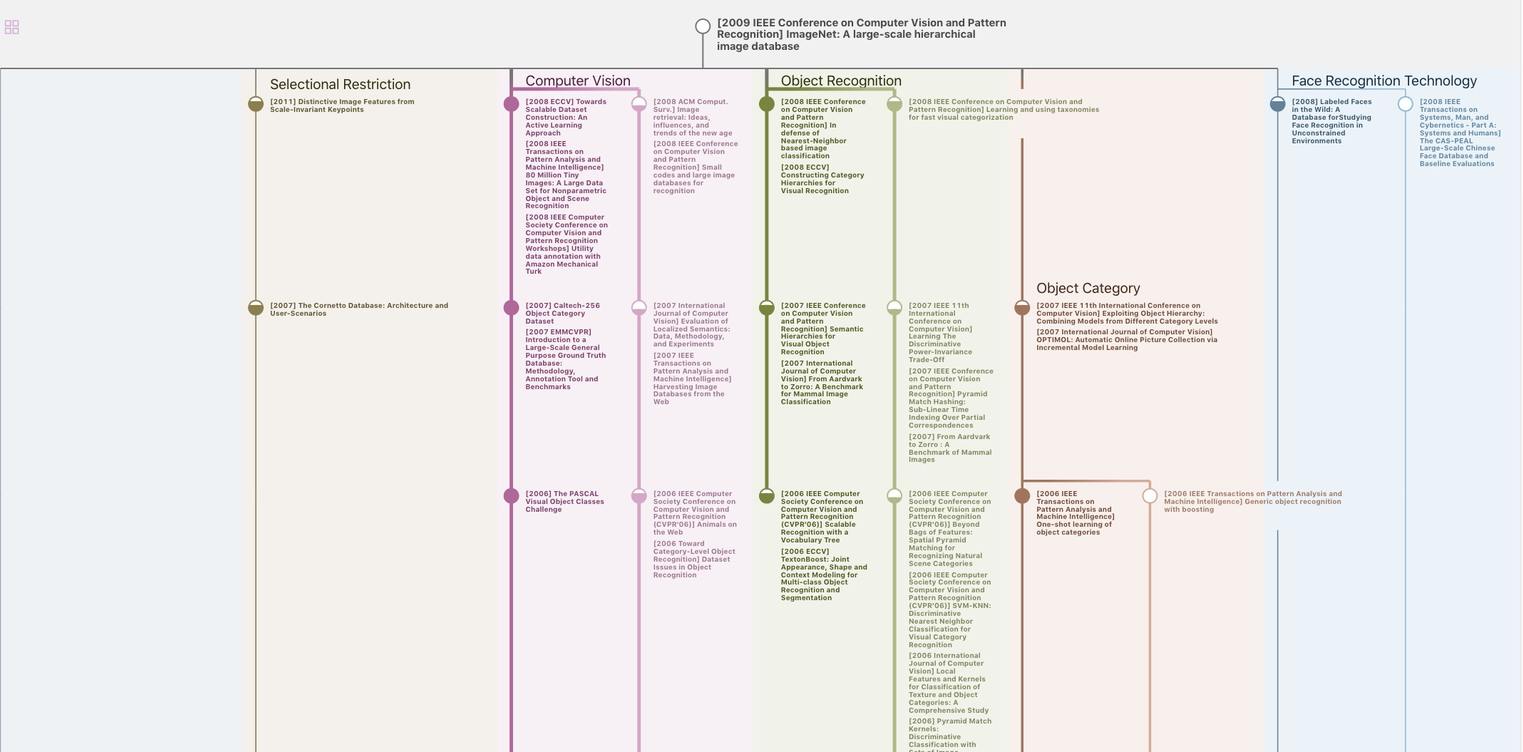
生成溯源树,研究论文发展脉络
Chat Paper
正在生成论文摘要