Anomaly detection frameworks for outlier and pattern anomaly of time series in wireless sensor networks
2020 International Conference on Networking and Network Applications (NaNA)(2020)
摘要
As a major form of data, the collection and analysis of time series have been widely used in many fields. In practice, wireless sensor network is a popular mechanism for data collection and it is suitable for time series. However, due to the diversity deployment area for a wireless sensor network, the external environmental factors pose a great challenge on the quality of data collected by a sensor node. Besides, the accuracy and reliability of collected data are also affected by internal factors of a sensor node and the performance of the whole wireless sensor network. Common internal factors are resource limitation such as battery capacity, computing ability, and memory size. Moreover, a wireless sensor network deployed for production use is very likely to suffer from malicious attacks from both inside and outside. Therefore, anomaly is inevitable for time series collected by a wireless sensor network. In the paper, we make an extensive review about two types of anomaly detection methods in time series for wireless sensor networks: outlier detection and pattern anomaly detection. For outlier detection, six categories of detection methods are elaborated. For pattern anomaly detection, two main ideas are discussed. Finally, we present the main drawbacks of existing approaches to outlier detection and pattern anomaly detection for time series. The possible future research directions are also summarized.
更多查看译文
关键词
anomaly detection,point anomaly,pattern anomaly,outlier,time series,wireless sensor networks,feature representation
AI 理解论文
溯源树
样例
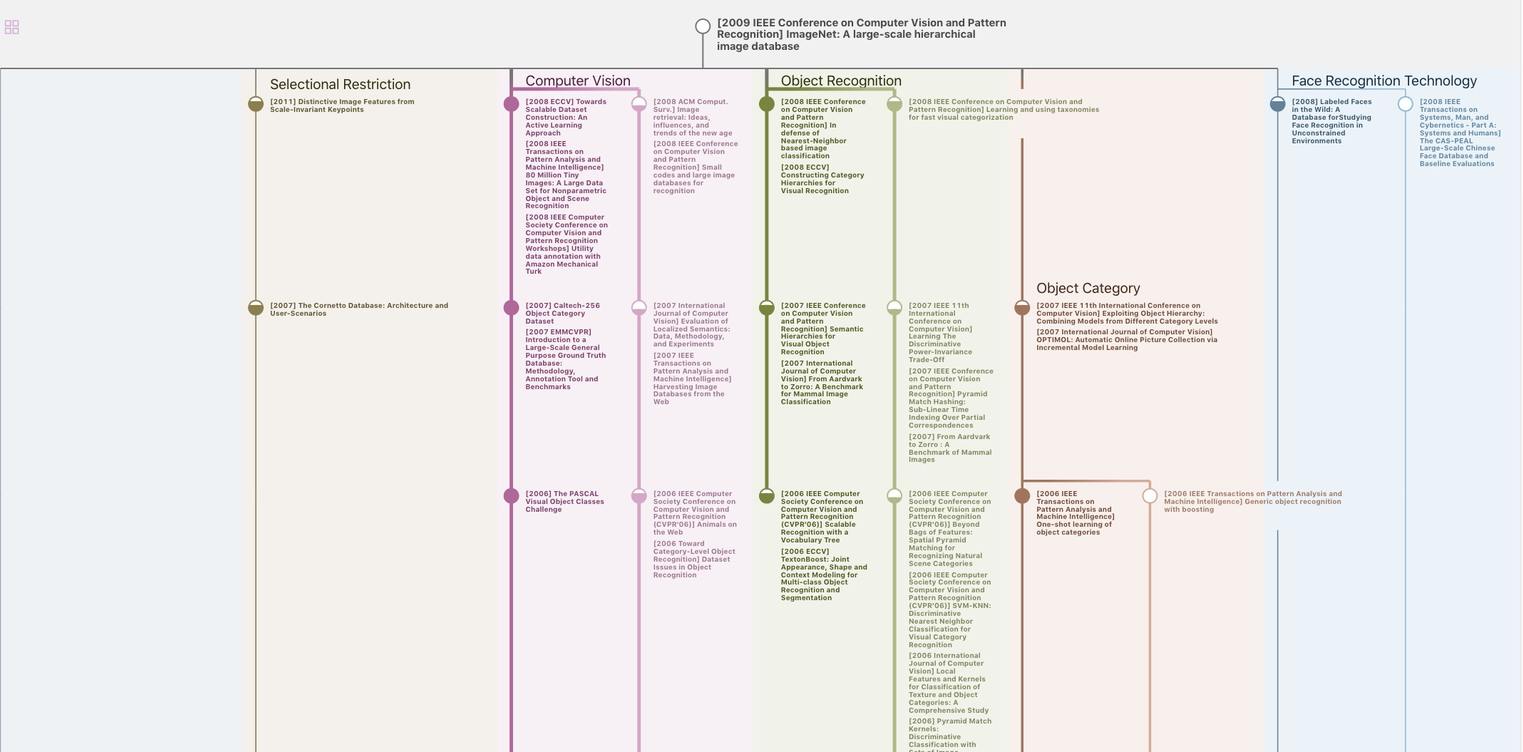
生成溯源树,研究论文发展脉络
Chat Paper
正在生成论文摘要