Latent Space Geometric Statistics.
ICPR Workshops(2020)
摘要
Deep generative models, e.g., variational autoencoders and generative adversarial networks, result in latent representation of observed data. The low dimensionality of the latent space provides an ideal setting for analysing high-dimensional data that would otherwise often be infeasible to handle statistically. The linear Euclidean geometry of the high-dimensional data space pulls back to a nonlinear Riemannian geometry on latent space where classical linear statistical techniques are no longer applicable. We show how analysis of data in their latent space representation can be performed using techniques from the field of geometric statistics. Geometric statistics provide generalisations of Euclidean statistical notions including means, principal component analysis, and maximum likelihood estimation of parametric distributions. Introduction to estimation procedures on latent space are considered, and the computational complexity of using geometric algorithms with high-dimensional data addressed by training a separate neural network to approximate the Riemannian metric and cometric tensor capturing the shape of the learned data manifold.
更多查看译文
关键词
statistics,space
AI 理解论文
溯源树
样例
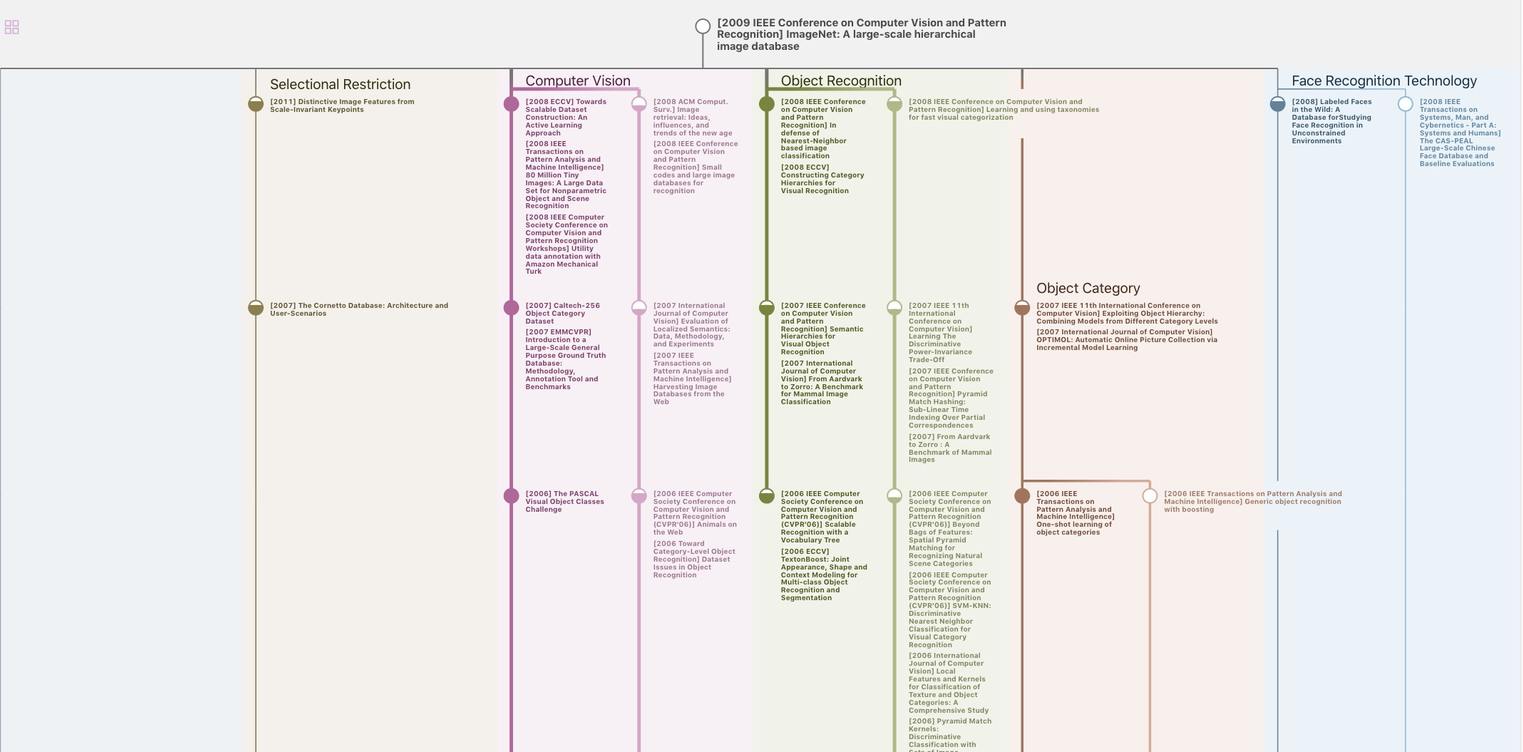
生成溯源树,研究论文发展脉络
Chat Paper
正在生成论文摘要