Multi-Group Multicast Beamforming by Superiorized Projections Onto Convex Sets
IEEE TRANSACTIONS ON SIGNAL PROCESSING(2021)
摘要
In this paper, we propose an iterative algorithm to address the nonconvex multi-group multicast beamforming problem with quality-of-service constraints and per-antenna power constraints. We formulate a convex relaxation of the problem as a semidefinite program in a real Hilbert space, which allows us to approximate a point in the feasible set by iteratively applying a bounded perturbation resilient fixed-point mapping. Inspired by the superiorization methodology, we use this mapping as a basic algorithm, and we add in each iteration a small perturbation with the intent to reduce the objective value and the distance to nonconvex rank-constraint sets. We prove that the sequence of perturbations is bounded, so the algorithm is guaranteed to converge to a feasible point of the relaxed semidefinite program. Simulations show that the proposed approach outperforms existing algorithms in terms of both computation time and approximation gap in many cases.
更多查看译文
关键词
Array signal processing, Signal processing algorithms, Quality of service, Approximation algorithms, Perturbation methods, Multicast algorithms, Convergence, Multicast beamforming, nonconvex optimization, semidefinite relaxation, projections onto convex sets, superiorization
AI 理解论文
溯源树
样例
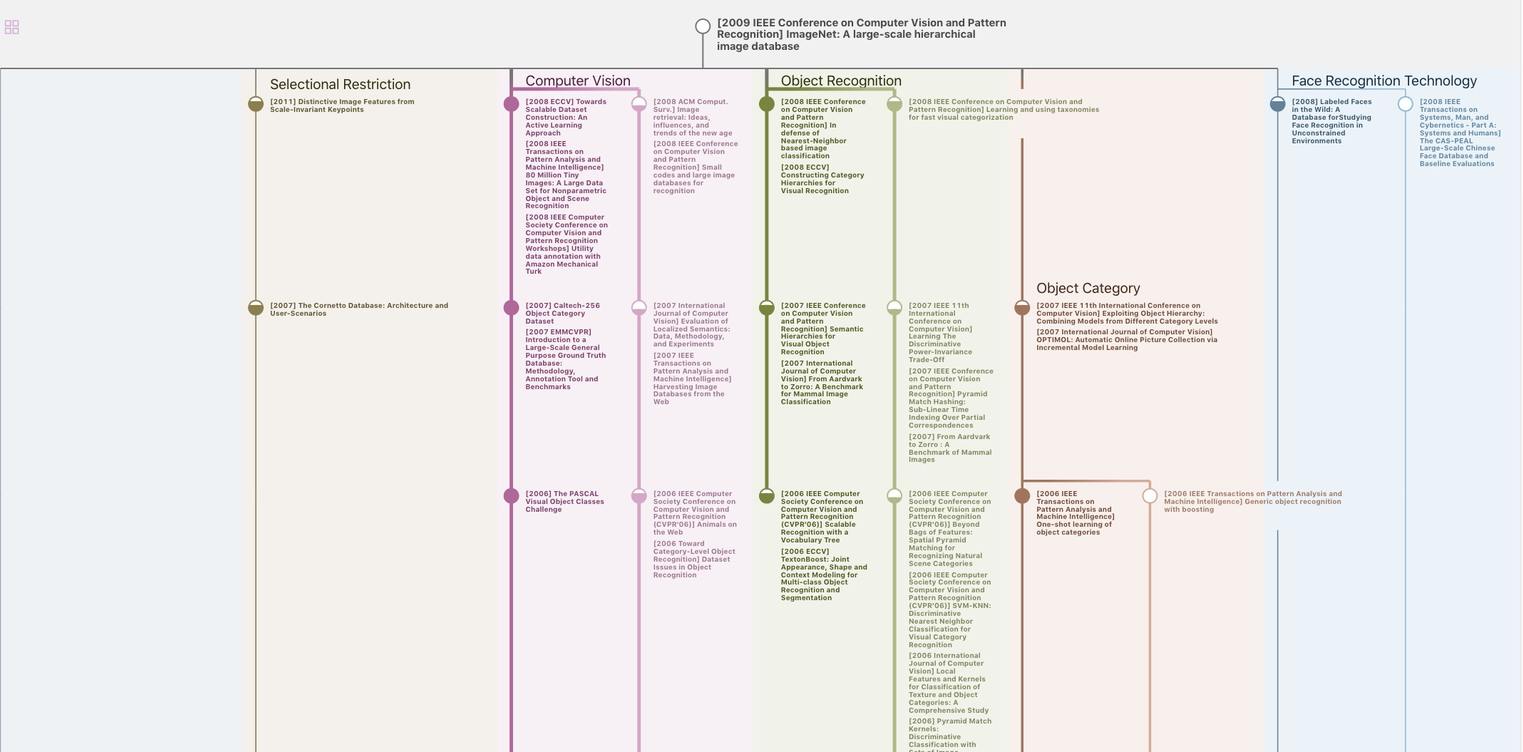
生成溯源树,研究论文发展脉络
Chat Paper
正在生成论文摘要