The Sensitivity of Word Embeddings-based Author Detection Models to Semantic-preserving Adversarial Perturbations
arxiv(2021)
摘要
Authorship analysis is an important subject in the field of natural language processing. It allows the detection of the most likely writer of articles, news, books, or messages. This technique has multiple uses in tasks related to authorship attribution, detection of plagiarism, style analysis, sources of misinformation, etc. The focus of this paper is to explore the limitations and sensitiveness of established approaches to adversarial manipulations of inputs. To this end, and using those established techniques, we first developed an experimental frame-work for author detection and input perturbations. Next, we experimentally evaluated the performance of the authorship detection model to a collection of semantic-preserving adversarial perturbations of input narratives. Finally, we compare and analyze the effects of different perturbation strategies, input and model configurations, and the effects of these on the author detection model.
更多查看译文
关键词
author detection models,adversarial perturbations,embeddings-based,semantic-preserving
AI 理解论文
溯源树
样例
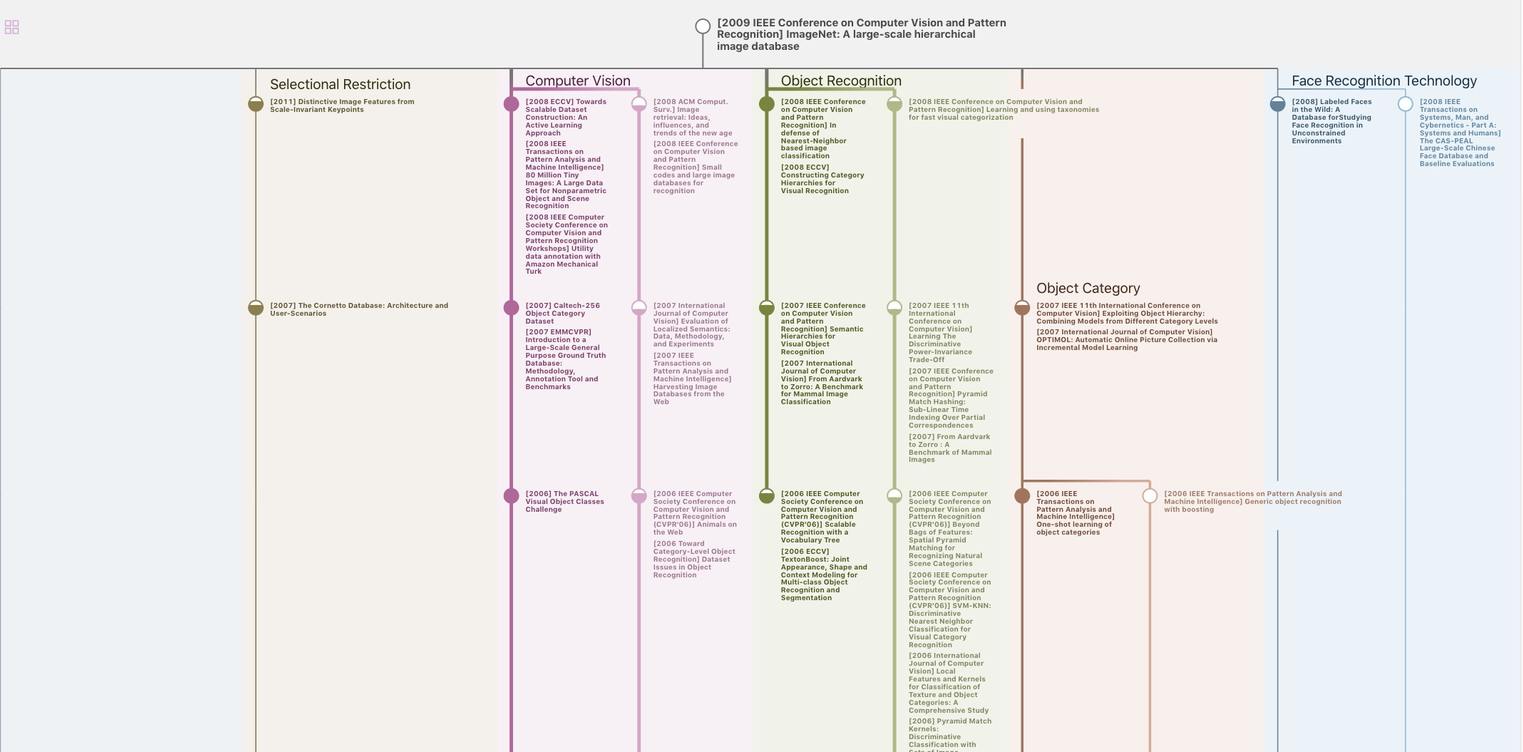
生成溯源树,研究论文发展脉络
Chat Paper
正在生成论文摘要