An artificial-neural-network based prediction of heat transfer behaviors for in-tube supercritical CO2 flow
Applied Soft Computing(2021)
摘要
Abstract Supercritical CO2 flowing in tubes has been researched intensively to confirm their potential applications in energy conversion systems. However its performance prediction is always damaged by the non-linear variable thermo-physical properties and conventional correlation methods. To this context, this paper considers GA-BP model performed in MATLAB software to improve prediction accuracy. Firstly, three heat-transfer regimes are defined and parametrical effects are evaluated. Then, an architecture of 2-5-5-1 network is well-trained for accurately redistributed reconstruction of non-linear density of sCO2 and achieves a reasonable root-mean-square error of 1.112 kg/m3 and a regression coefficient of 0.99. Finally, using 5895 sets of reliable experimental data with a verified architecture of 5-150-1 network makes the ANN model more adaptive and precise in interval predictions of heat-transfer behaviors, with a mean-absolute-percent error and as root-mean-square error far less than 2.97% and 3.11°C, respectively. Results also suggest that the ANN model is technically superior to those correlations by I. Pioro, T. Preda, H. Kim, J.D. Jackson and Bringer-Smith under established test 1-5 groups. This study can provide a methodological guidance and shed a new insight for the further prediction of heat-transfer problems with supercritical fluids.
更多查看译文
关键词
Artificial neural network, Supercritical CO2, Thermophysical property, Thermal behavior, Empirical correlation
AI 理解论文
溯源树
样例
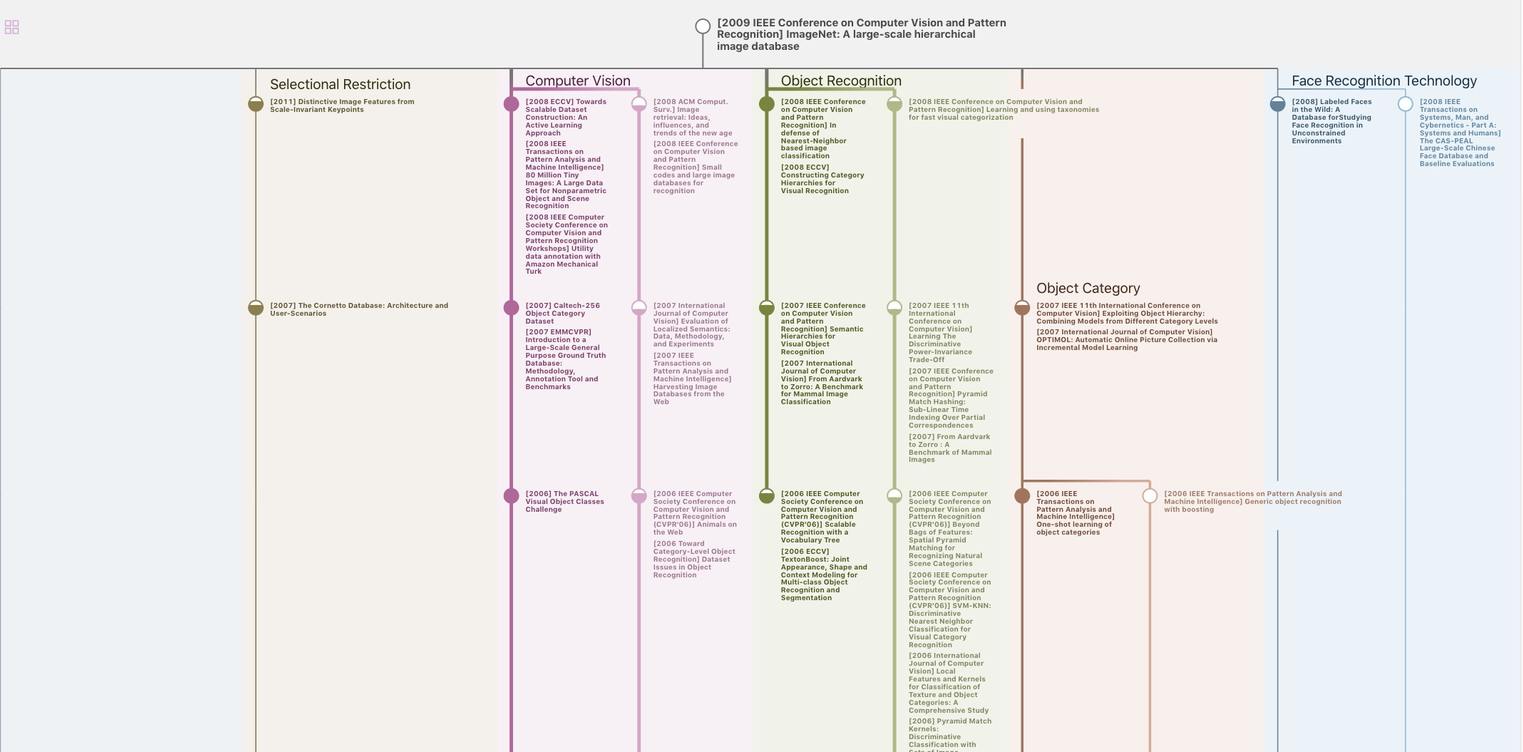
生成溯源树,研究论文发展脉络
Chat Paper
正在生成论文摘要