Explaining black-box classifiers using post-hoc explanations-by-example: The effect of explanations and error-rates in XAI user studies
Artificial Intelligence(2021)
摘要
•Series of controlled user studies examining post-hoc example-based explanations for black-box deep learners doing classification (XAI).•Black box AI models can be explained by “twinning” them with white-box models.•Explanations were only found to impact people’s perception of errors.•Explanations lead people to view errors as being “less incorrect”, but they do not improve trust.•Trust in an AI model is undermined by increases in error-rates (from 3% error-levels onwards).
更多查看译文
关键词
Explainable AI,Factual explanation,Trust,User testing,Convolutional neural network,Case-based reasoning,Deep learning,k-nearest neighbours
AI 理解论文
溯源树
样例
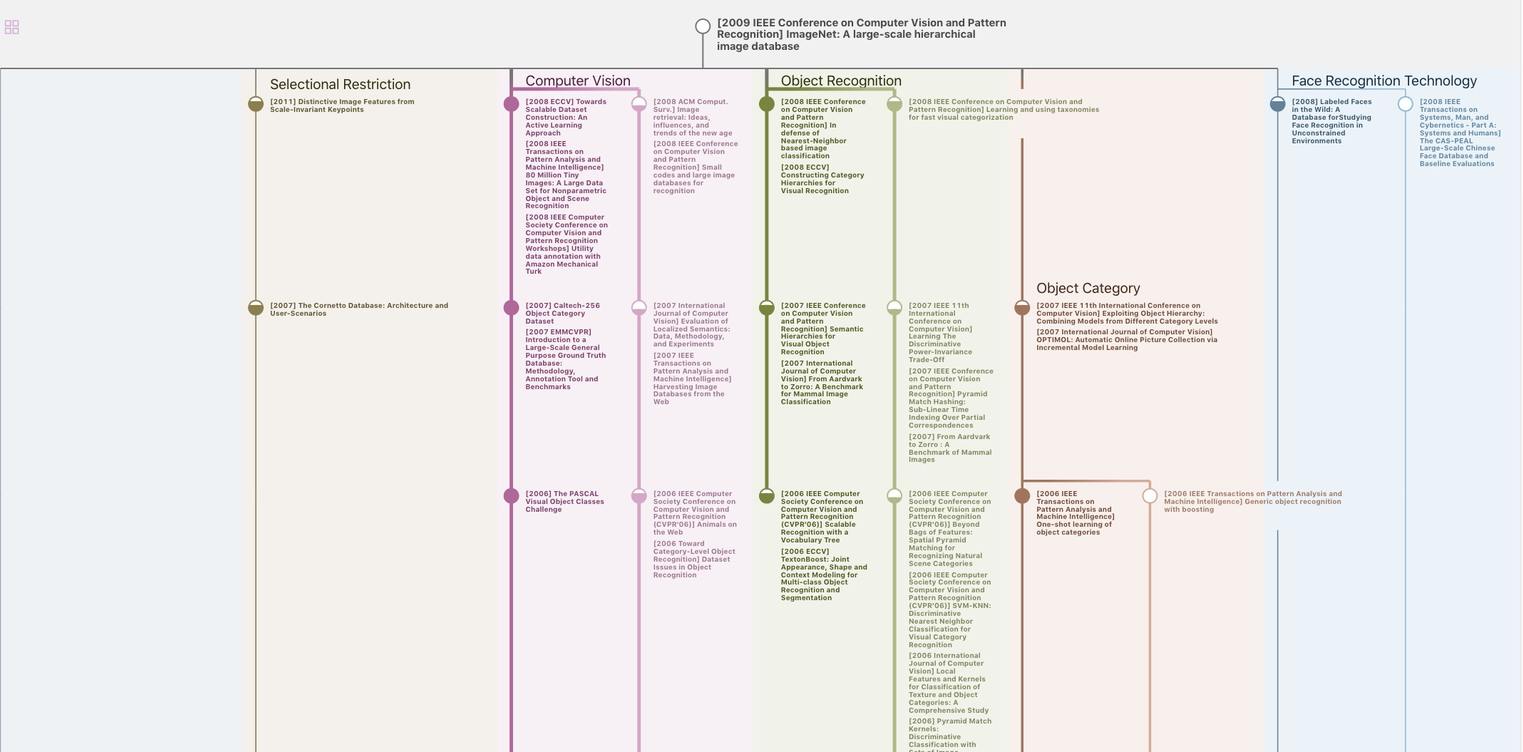
生成溯源树,研究论文发展脉络
Chat Paper
正在生成论文摘要